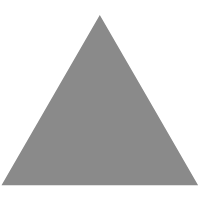
38
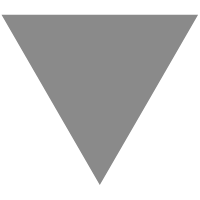
GitHub - megvii-model/DetNAS
source link: https://github.com/megvii-model/DetNAS
Go to the source link to view the article. You can view the picture content, updated content and better typesetting reading experience. If the link is broken, please click the button below to view the snapshot at that time.
README.md
DetNAS
This project provides the implementation for DetNAS: Backbone Search for Object Detection. As we originally conducted the experiments in the paper using the internal framework Brain++, this project is a reimplemented version on PyTorch. In addition, this project is based on maskrcnn-benchmark.
Installation
- Modify the path to your coco dataset in config.sh.
bash config.sh
Trained Models
Model ImageNet acc AP (minival) GoogleDrive DetNAS-COCO-FPN-300M 26.2 36.6 ImageNet COCO DetNAS-COCO-FPN-1.3G 22.8 40.3 ImageNet COCO DetNAS-COCO-FPN-3.8G 21.6 42.0 ImageNet COCO DetNAS-COCO-RetinaNet-300M 26.0 34.1 ImageNet COCOThe training scripts of these model are in the dirctory scripts/
. For training,
- Download the ImageNet model to the directory
ImageNet-Pretrain-models/
. bash scripts/run_detnas_coco_fpn_300M.sh
Search for networks
Step 1: setup Dataset
- We have splitted 5000 images from
coco_2014_train
+coco_2014_valminusminival
as the validation set for search. The remainings are used for supernet training. - Download the splitted train and val json files (GoogleDrive) to
datasets/coco/annotations
. - (You can replace them with your own datasets.)
Step 2: Supernet training
ImageNet pre-training
- Download the ImageNet supernet model (GoogleDrive) to the directory
ImageNet-Pretrain-models/
. - If necessary, you can also train models ImageNet by yourselves. Please refer to the folder
Supernet-ImageNet/
.
COCO training
bash scripts/run_detnas_coco_fpn_300M_search.sh
- ('-search' in cfg.MODEL.BACKBONE.CONV_BODY is to distinguish supernet training from single model.)
Step 3: setup a server for the distributed search
tmux new -s mq_server
sudo apt update
sudo apt install rabbitmq-server
sudo service rabbitmq-server start
sudo rabbitmqctl add_user test test
sudo rabbitmqctl set_permissions -p / test '.*' '.*' '.*'
Step 4: start a new tmux for search
tmux new -s search
- modify
host
andlog_dir
in the config filedistributed_arch_search/arch_search_config.py
. bash distributed_arch_search/run_search.sh
- (
run_search.sh
requires no GPUs.)
Step 5: start new tmuxs for model evaluation (concurrent with Step 4)
tmux new -s server_x
- modify
config-file
andMODEL.WEIGHT
in the script filedistributed_arch_search/run_server.sh
. bash distributed_arch_search/run_server.sh
- (You can start more than one
run_server.sh
to speed up, if you have enough GPUs and memory researces.)
Citation
Please cite DetNAS in your publications if it helps your research.
@misc{chen2019detnas,
title={DetNAS: Backbone Search for Object Detection},
author={Yukang Chen, Tong Yang, Xiangyu Zhang, Gaofeng Meng, Xinyu Xiao, Jian Sun},
year={2019},
booktitle = {NeurIPS},
}
Recommend
About Joyk
Aggregate valuable and interesting links.
Joyk means Joy of geeK