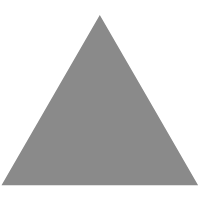
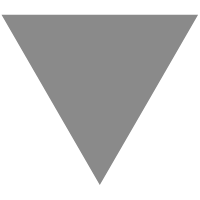
推荐广告入门:DeepCTR-Torch,基于深度学习的CTR预测算法库
source link: https://www.tuicool.com/articles/V7Nnyy
Go to the source link to view the article. You can view the picture content, updated content and better typesetting reading experience. If the link is broken, please click the button below to view the snapshot at that time.
在计算广告和推荐系统中, CTR预估 一直是一个核心问题。无论在工业界还是学术界都是一个热点研究问题,近年来也有若干相关的算法竞赛陆续举办。本文介绍一个使用PyTorch编写的深度学习的点击率预测算法库 DeepCTR-Torch ,具有简洁易用、模块化和可扩展的优点,非常适合初学者快速入门学习。
(本文作者: 沈伟臣,阿里巴巴算法工程师)
点击率预估问题
点击率预估问题通常形式化描述为给定用户,物料,上下文的情况下,计算用户点击物料的概率即: pCTR = p(click=1|user,item,context) 。
简单来说,在广告业务中使用pCTR来计算广告的预期收益,在推荐业务中通过使用pCTR来确定候选物料的一个排序列表。
DeepCTR-Torch
人们通过构造有效的组合特征和使用复杂的模型来学习数据中的模式来提升效果。基于因子分解机的方法,可以通过向量乘积的形式学习特征的交互,并且泛化到那些没有出现过的组合上。
随着深度神经网络在若干领域的巨大发展,近年来研究者也提出了若干基于深度学习的分解模型来同时学习低阶和高阶的特征交互,如:
PNN,Wide&Deep,DeepFM,Attentional FM,Neural FM,DCN,xDeepFM,AutoInt,FiBiNET
以及基于用户历史行为序列建模的 DIN,DIEN,DSIN 等。
对于刚接触这方面的同学来说,可能对这些方法的细节还不太了解,虽然网上有很多介绍,但是代码却没有统一的形式,且当想要迁移到自己的数据集进行实验时也很不方便。本文介绍的一个使用PyTorch实现的基于深度学习的CTR模型包DeepCTR-PyTorch,无论是使用还是学习都很方便。
DeepCTR-PyTorch是一个 简洁易用、模块化 和 可扩展 的基于深度学习的CTR模型包。除了近年来主流模型外,还包括许多可用于轻松构建您自己的自定义模型的核心组件层。
您简单的通过 model.fit()
和 model.predict()
来使用这些复杂的模型执行训练和预测任务,以及在通过模型初始化列表的 device
参数来指定运行在cpu还是gpu上。
安装与使用
-
安装
pip install -U deepctr-torch
-
使用例子
下面用一个简单的例子告诉大家,如何快速的应用一个基于深度学习的CTR模型,代码地址在:
https://github.com/shenweichen/DeepCTR-Torch/blob/master/examples/run_classification_criteo.py 。
The Criteo Display Ads dataset是 kaggle 上的一个CTR预估竞赛数据集。里面包含13个数值特征 I1-I13 和26个类别特征 C1-C26 。
# -*- coding: utf-8 -*-
# 使用pandas 读取上面介绍的数据,并进行简单的缺失值填充
import pandas as pd
from sklearn.metrics import log_loss, roc_auc_score
from sklearn.model_selection import train_test_split
from sklearn.preprocessing import LabelEncoder, MinMaxScaler
from deepctr_torch.models import *
from deepctr_torch.inputs import SparseFeat, DenseFeat, get_fixlen_feature_names
import torch
# 使用pandas 读取上面介绍的数据,并进行简单的缺失值填充
data = pd.read_csv('./criteo_sample.txt')
# 上面的数据在:https://github.com/shenweichen/DeepCTR-Torch/blob/master/examples/criteo_sample.txt
sparse_features = ['C' + str(i) for i in range(1, 27)]
dense_features = ['I' + str(i) for i in range(1, 14)]
data[sparse_features] = data[sparse_features].fillna('-1', )
data[dense_features] = data[dense_features].fillna(0, )
target = ['label']
#这里我们需要对特征进行一些预处理,对于类别特征,我们使用LabelEncoder重新编码(或者哈希编码),对于数值特征使用MinMaxScaler压缩到0~1之间。
for feat in sparse_features:
lbe = LabelEncoder()
data[feat] = lbe.fit_transform(data[feat])
mms = MinMaxScaler(feature_range=(0, 1))
data[dense_features] = mms.fit_transform(data[dense_features])
# 这里是比较关键的一步,因为我们需要对类别特征进行Embedding,所以需要告诉模型每一个特征组有多少个embbedding向量,我们通过pandas的nunique()方法统计。
fixlen_feature_columns = [SparseFeat(feat, data[feat].nunique())
for feat in sparse_features] + [DenseFeat(feat, 1,)
for feat in dense_features]
dnn_feature_columns = fixlen_feature_columns
linear_feature_columns = fixlen_feature_columns
fixlen_feature_names = get_fixlen_feature_names(
linear_feature_columns + dnn_feature_columns)
#最后,我们按照上一步生成的特征列拼接数据
train, test = train_test_split(data, test_size=0.2)
train_model_input = [train[name] for name in fixlen_feature_names]
test_model_input = [test[name] for name in fixlen_feature_names]
# 检查是否可以使用gpu
device = 'cpu'
use_cuda = True
if use_cuda and torch.cuda.is_available():
print('cuda ready...')
device = 'cuda:0'
# 初始化模型,进行训练和预测
model = DeepFM(linear_feature_columns=linear_feature_columns, dnn_feature_columns=dnn_feature_columns, task='binary',
l2_reg_embedding=1e-5, device=device)
model.compile("adagrad", "binary_crossentropy",
metrics=["binary_crossentropy", "auc"],)
model.fit(train_model_input, train[target].values,
batch_size=256, epochs=10, validation_split=0.2, verbose=2)
pred_ans = model.predict(test_model_input, 256)
print("")
print("test LogLoss", round(log_loss(test[target].values, pred_ans), 4))
print("test AUC", round(roc_auc_score(test[target].values, pred_ans), 4))
相关资料
-
DeepCTR-Torch代码主页
https://github.com/shenweichen/DeepCTR-Torch
-
DeepCTR-Torch文档:
https://deepctr-torch.readthedocs.io/en/latest/index.html
-
DeepCTR(tensorflow版)代码主页 :
https://github.com/shenweichen/DeepCTR
-
DeepCTR(tensorflow版)文档:
https://deepctr-doc.readthedocs.io/en/latest/index.html
作者简介
沈伟臣,浙江大学计算机硕士,阿里巴巴集团算法工程师
沈伟臣曾经参与了《DeepLearning.ai深度学习 》 笔记的编写。
github主页:
https://github.com/shenweichen
知乎专栏 浅梦的学习笔记
https://zhuanlan.zhihu.com/weichennote
本站简介↓↓↓
“机器学习初学者”是帮助人工智能爱好者入门的个人公众号(创始人: 黄海广)
初学者入门的道路上,最需要的是“雪中送炭”,而不是“锦上添花”。
本站的知识星球(黄博的机器学习圈子)ID:92416895
目前在机器学习方向的知识星球排名第一(上图二维码)
往期
精彩
回顾
Recommend
About Joyk
Aggregate valuable and interesting links.
Joyk means Joy of geeK