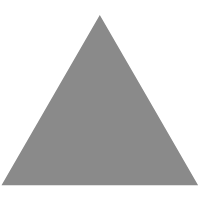
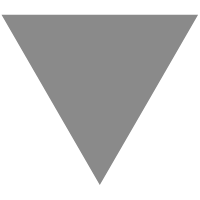
GitHub - apache/hive: Apache Hive
source link: https://github.com/apache/hive
Go to the source link to view the article. You can view the picture content, updated content and better typesetting reading experience. If the link is broken, please click the button below to view the snapshot at that time.
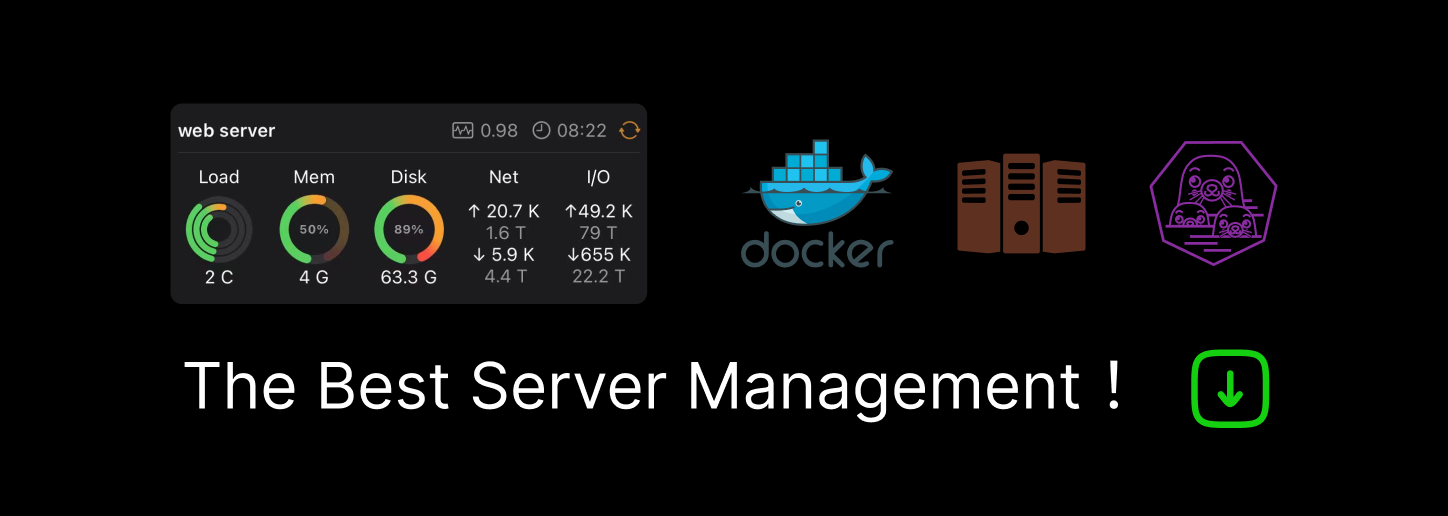
README.md
Apache Hive (TM)
The Apache Hive (TM) data warehouse software facilitates reading, writing, and managing large datasets residing in distributed storage using SQL. Built on top of Apache Hadoop (TM), it provides:
-
Tools to enable easy access to data via SQL, thus enabling data warehousing tasks such as extract/transform/load (ETL), reporting, and data analysis
-
A mechanism to impose structure on a variety of data formats
-
Access to files stored either directly in Apache HDFS (TM) or in other data storage systems such as Apache HBase (TM)
-
Query execution using Apache Hadoop MapReduce, Apache Tez or Apache Spark frameworks.
Hive provides standard SQL functionality, including many of the later 2003 and 2011 features for analytics. These include OLAP functions, subqueries, common table expressions, and more. Hive's SQL can also be extended with user code via user defined functions (UDFs), user defined aggregates (UDAFs), and user defined table functions (UDTFs).
Hive users have a choice of 3 runtimes when executing SQL queries. Users can choose between Apache Hadoop MapReduce, Apache Tez or Apache Spark frameworks as their execution backend. MapReduce is a mature framework that is proven at large scales. However, MapReduce is a purely batch framework, and queries using it may experience higher latencies (tens of seconds), even over small datasets. Apache Tez is designed for interactive query, and has substantially reduced overheads versus MapReduce. Apache Spark is a cluster computing framework that's built outside of MapReduce, but on top of HDFS, with a notion of composable and transformable distributed collection of items called Resilient Distributed Dataset (RDD) which allows processing and analysis without traditional intermediate stages that MapReduce introduces.
Users are free to switch back and forth between these frameworks at any time. In each case, Hive is best suited for use cases where the amount of data processed is large enough to require a distributed system.
Hive is not designed for online transaction processing. It is best used for traditional data warehousing tasks. Hive is designed to maximize scalability (scale out with more machines added dynamically to the Hadoop cluster), performance, extensibility, fault-tolerance, and loose-coupling with its input formats.
General Info
For the latest information about Hive, please visit out website at:
Getting Started
-
Installation Instructions and a quick tutorial: https://cwiki.apache.org/confluence/display/Hive/GettingStarted
-
A longer tutorial that covers more features of HiveQL: https://cwiki.apache.org/confluence/display/Hive/Tutorial
-
The HiveQL Language Manual: https://cwiki.apache.org/confluence/display/Hive/LanguageManual
Requirements
-
Java 1.7 or 1.8
-
Hadoop 1.x, 2.x, 3.x (3.x required for Hive 3.x)
Upgrading from older versions of Hive
-
Hive includes changes to the MetaStore schema. If you are upgrading from an earlier version of Hive it is imperative that you upgrade the MetaStore schema by running the appropriate schema upgrade scripts located in the scripts/metastore/upgrade directory.
-
We have provided upgrade scripts for MySQL, PostgreSQL, Oracle, Microsoft SQL Server, and Derby databases. If you are using a different database for your MetaStore you will need to provide your own upgrade script.
Useful mailing lists
-
[email protected] - To discuss and ask usage questions. Send an empty email to [email protected] in order to subscribe to this mailing list.
-
[email protected] - For discussions about code, design and features. Send an empty email to [email protected] in order to subscribe to this mailing list.
-
[email protected] - In order to monitor commits to the source repository. Send an empty email to [email protected] in order to subscribe to this mailing list.
Recommend
-
58
对于数据密集型任务,I/O操作和网络数据传输需要花费相当长的时间才能完成。通过在 Hive 中启用压缩功能,我们可以提高 Hive 查询的性能,并节省 HDFS 集群上的存储空间。 1. Hive中的可用压缩编解码器要在 Hive 中启用压缩,首先我们需要找出 Hadoop 集群上可用的...
-
66
-
45
Home Blog
-
14
Apache Hive Installation on Ubuntu Last updated on May 22,2019 118.7K Views
-
8
Apache Hive 2.1 Installation with TroubleShooting Reading Time: 3 minutesApache Hive is considered the defacto stan...
-
8
Understanding Apache Hive LLAPApache Hive is a complex system when you look at it, but once you go looking for more info, it’s more interesting than complex. There are multiple query engines available for Hive, and then there’s LLAP on top of...
-
12
Reading Time: 2 minutesPartitions Hive is a good tool for performing queries on large datasets, especially datasets that require full table scans. But quite often there are instances where users need to filter the data on specific c...
-
5
This article was published as a part of the Data Science Blogathon. Introduction I’ve always wondered how big companies like Google process their information...
-
8
This article was published as a part of the Data Science Blogathon. Introduction With the increasing daily data, the need for business intelligence is fl...
-
3
Offline Data Pipeline Best Practices Part 2:Optimizing Airflow Job Parameters for Apache Hive ...
About Joyk
Aggregate valuable and interesting links.
Joyk means Joy of geeK