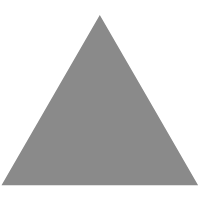
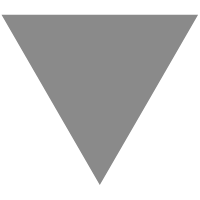
Numpy dispatcher: when Numpy becomes a protocol for an ecosystem
source link: https://www.tuicool.com/articles/niayYvi
Go to the source link to view the article. You can view the picture content, updated content and better typesetting reading experience. If the link is broken, please click the button below to view the snapshot at that time.
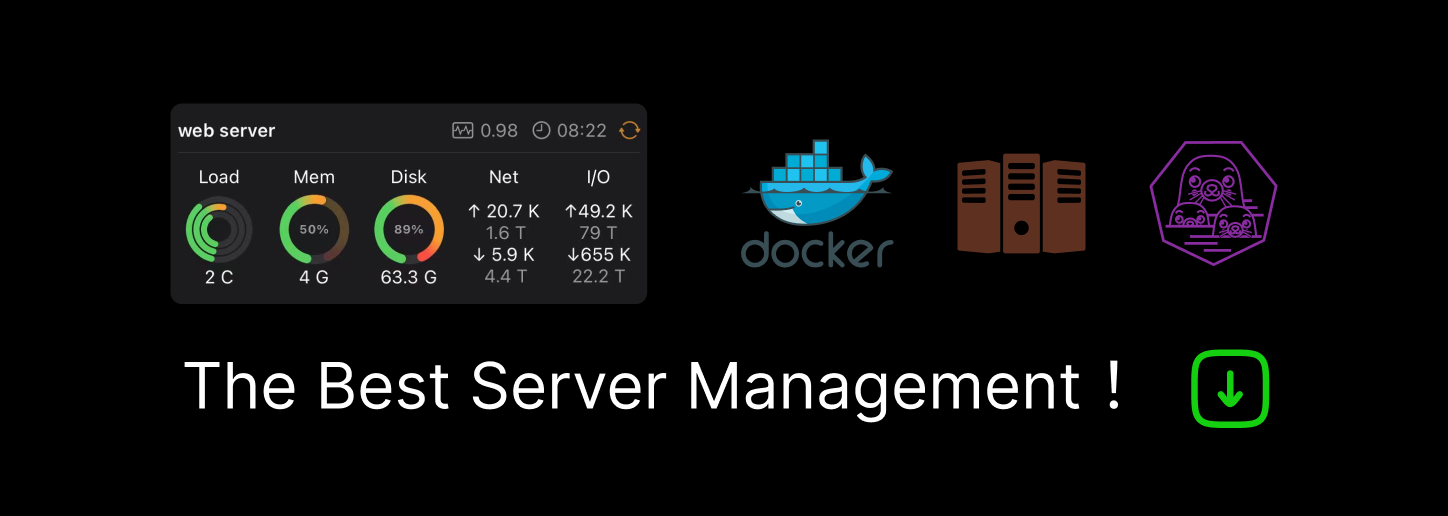
Introduction
Not a lot of people working with the Python scientific ecosystem are aware of the NEP 18 ( dispatch mechanism for NumPy’s high-level array functions ). Given the importance of this protocol, I decided to write this short introduction to the new dispatcher that will certainly bring a lot of benefits for the Python scientific ecosystem.
If you used PyTorch, TensorFlow, Dask, etc, you certainly noticed the similarity of their API contracts with Numpy. And it’s not by accident, Numpy’s API is one of the most fundamental and widely-used APIs for scientific computing. Numpy is so pervasive, that it ceased to be only an API and it is becoming more a protocol or an API specification.
There are countless advantages when an an entire ecosystem adopts an API specification. The wide adoption of the Numpy protocols will certainly help create a standardized API that will provide seamless integration across implementations, even for the ones that are quite different than the ndarray
.
The main goal of the NEP 18 , is to provide a protocol that is materialized on the method named __array_function__
, where it will allow developers of frameworks such as PyTorch, TensorFlow, Dask, etc, to provide their own implementations of the Numpy API specification.
The __array_function__
protocol is still experimental and will be enabled by default on the next Numpy v.1.17, however, on the v1.16 you can enable it by setting the environment variable called NUMPY_EXPERIMENTAL_ARRAY_FUNCTION
to 1.
PyTorch example
It’s easy to provide an example of how the protocol can be implemented in PyTorch in order to get a feeling of what it will allow you to do.
First, we define the protocol that we will use to monkey-patch PyTorch ( but you can change the framework itself, of course, that’s the whole point ):
HANDLED_FUNCTIONS = {} def __array_function__(self, func, types, args, kwargs): if func not in HANDLED_FUNCTIONS: return NotImplemented if not all(issubclass(t, torch.Tensor) for t in types): return NotImplemented return HANDLED_FUNCTIONS[func](*args, **kwargs)
After that, we create a simple decorator to set the HANDLED_FUNCTIONS
dictionary with PyTorch’s implementation of the Numpy API:
def implements(numpy_function): def decorator(func): HANDLED_FUNCTIONS[numpy_function] = func return func return decorator
Later, we just have to monkey patch PyTorch ( not required if you change PyTorch code itself ) and provide a Numpy implementation of the PyTorch operations:
torch.Tensor.__array_function__ = __array_function__ @implements(np.sum) def npsum(arr, axis=0): return torch.sum(arr, dim=axis)
And that’s it ! You can now do things like this:
>>> torch_tensor = torch.ones(10) >>> type(torch_tensor) torch.Tensor >>> result = np.sum(torch_tensor) >>> result tensor(10.) >>> type(result) torch.Tensor
This is quite amazing, given that you can easily mix APIs together and write a single piece of code that will work in PyTorch, Numpy or other frameworks independently of the multi-dimensional array implementation and diverging to the correct backend implementation.
Dask example
Dask is already implementing the protocol , so you don’t have to monkey-patch it:
>>> import numpy as np >>> import dask.array as da >>> dask_array = da.random.random((5000, 1000), chunks=(1000, 1000)) >>> type(dask_array) dask.array.core.Array >>> u, s, v = np.linalg.svd(dask_array) # Numpy API ! >>> u
>>> s
>>> v
As you can see, when we used the singular decomposition from Numpy, we were able to define the Dask computational graph using the Numpy API:
>>> v.visualize()
And we can now compute the result using Dask lazy eval:
>>> result = v.compute() >>> result array([[ 0.03178809, 0.03147971, 0.0313492 , ..., 0.03201226, 0.03142089, 0.03180248], [ 0.00272678, -0.0182263 , 0.00839066, ..., 0.01195828, 0.01681703, -0.05086809], [-0.00851417, 0.0411336 , 0.02738713, ..., 0.03447079, 0.04614097, 0.02292196], ...,
And you can do the same with CuPy as well.
The new dispatch protocol will open the door for seamless integration among frameworks on the Python scientific ecosystem. I hope that more and more frameworks will start to adopt it in near future.
– Christian S. Perone
Recommend
-
207
dispatch.vim Leverage the power of Vim's compiler plugins without being bound by synchronicity. Kick off builds and test suites using one of several asynchronous adapters (including tmux, screen, iTerm, Windows, and a headless mode), and...
-
88
PermissionsDispatcher Fully Kotlin/Java support Special permissions support
-
87
symfony/event-dispatcher: The EventDispatcher component provides tools that allow your application components to communicate with each other by dispatching events and listening to them.
-
43
Simple and light-weight event dispatcher for Kotlin
-
45
Posts in this series: A Primer
-
128
README.md Brigadier Brigadier is a command parser & dispatcher, designed and developed for Minecraft: Java Edition and now freely available for use...
-
7
[enzymne protocol] Dispatcher & ControllerLib合约分析
-
8
MicroPython officially becomes part of the Arduino ecosystem Arduino Team — November 10th, 2022
-
5
BitGo Becomes First Custodian to Support Sui Ecosystem Sui Foundation treasury will be held in qualified custody and launch...
-
7
CORRECTING and REPLACING BitGo Becomes First Custodian to Support Sui Ecosystem Sui Foundation treasury will be held in qua...
About Joyk
Aggregate valuable and interesting links.
Joyk means Joy of geeK