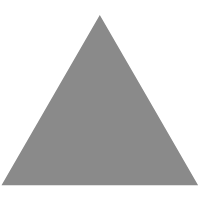
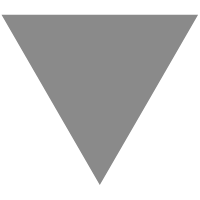
Counting to infinity at compile time
source link: https://www.tuicool.com/articles/7fuMvqu
Go to the source link to view the article. You can view the picture content, updated content and better typesetting reading experience. If the link is broken, please click the button below to view the snapshot at that time.
Higher better faster longer
Jun 2 ·10min read
We saw in aprevious blog post of mine an introduction to counting at compile time in scala, using the conventional Nat
type.
Unfortunately, Nat
is slow. Really, really slow, and there are hard limits on what we can make the scala compiler do, as it currently stands.
For example, here are some rough and ready and entirely-unscientific benchmarks I’ve just run on approximate compile times for the Sum
typeclass we defined last time. The numbers would be completely different on your machine, but probably to the right order of magnitude:
Sum Approx Time ------------------------ 3+6 0s 8+8 0s 10+7 0s 12+12 2s 15+15 <does not complete> 17+4 1s 20+5 7s 20+8 104s 22+11 <does not complete> 22+22 <does not complete>
So, as you can see, it’s less than ideal for real, practical uses. It’s true we’re asking the compiler to do a lot, and the scala compiler is famously slow, but we’d still like to have Nat
computations complete in a reasonable time. I don’t want the scala compiler to be the limiting factor, I want the JVM to be the limiting factor.
But first, why do we want that?
What is it good for
FString
If you’re a very dedicated reader of my blog, you’ll no doubt remember that a long time ago I wrote ablog post about preventing data-too-wide exceptions in database-based code.
The overall concept is a type FString
which is parameterised by a flavour of Nat
:
The idea being that an FString[_7]
contains a String
which you have proven to be of length ≤7, by going through the safe constructor from
. If these types are present in your class which represents your database table, and the lengths mimic the column widths in your database, then you can never have a data-too-wide exception again. The length evidence will stretch all the way from your API to your database, and any invalid data will be kicked out correctly at the boundaries to your program.
You can write methods on FString
which use Nat
typeclasses like the following:
So you can see why in this case it’s important to have a speedy Sum
in particular. Every time you add an FString
to another we add their lengths. This may never occur in your code, or it could be very frequent such as concatenating first and last names, or address components.
Matrices
Another use would be the encoding of matrix dimensions. When you multiply two matrices together, the width of one must match the height of the other. But this information is traditionally not represented at compile time, meaning there’s a (very frequent) exception case just waiting to jump out.
But if you defined your matrices parameterised by their dimensions as:
then you can set things up so multiplying two invalid matrices is a compile-time impossibility.
In particular, in data science and machine learning very large matrices are necessary. Very, very large. Billions of columns and rows large. Clearly using traditional Nat
for such a case is a non-starter.
Etc
And so on. Any time you have bounded data or size limits, or exceptions caused by arithmetic problems, Nat
can remove entire swathes of run time problems from your code.
Why is it so slow
Recall the algorithm for Nat
’s Sum
. It’s recursive — it calls itself after it has taken one layer of Succ
from one parameter and put it on top of the other. The base case, where the recursion terminates, is when the side it is deconstructing reaches zero, at which point we return whatever we have calculated on the other.
The first thing that jumps out is that the algorithm itself is pretty inefficient. The complexity for calculating n+m
is O(n)
.
An immediate ‘fix’ for this is to consider that we needn’t unwrap one Succ
layer at a time: we could do two if we want, and have the single Succ
case as a ‘back-up’ low-priority implicit for the final case.
Now when you add 11+5
, the following flow would be observed:
11 + 5 = 9 + 7 = 7 + 9 = 5 + 11 = 3 + 13 = 1 + 15 = 0 + 16 = 16
So we’ve improved the complexity of the operation. But why stop at 2? If we can do 2 we can do 4, and 8, and 16.
By this point we’re encoding the operation of Sum
by deconstructing our Nat
into its binary form. If we’re operating on _27
then we would deconstruct it as 16+8+2+1
in our implicit searching whilst summing.
But this idea is not satisfying, because there’s no way in this approach to encode an arbitrary binary solution. We have to encode the 8 and 16 and etc cases manually.
Also, it turns out, it’s not that efficient. Here is an implementation with the first extra layer, handling two Succ
layers at once:
Again with the entirely unscientific benchmarks, but it sped up my local calculation compile time by about 40%.
Not as fast as we would expect. We should expect a halving of the compile time based purely on the algorithm. What’s the problem?
The problem is the encoding of the number itself: Succ
. It’s too many nested types. Each time you add one you increase the nesting and the entire thing becomes more cumbersome for the compiler to handle and compare.
Imagine we strip four layers of Succ
from _11
: the compiler has to examine _11
, count four layers of type nesting, unwrap it, then apply four layers of type nesting to the other number.
While we decreased the complexity of the numerical algorithm, we didn’t do much to the actual job the compiler has to do. Wouldn’t it be great if we had just one operation to do at every stage, instead of four or more like in the above example?
Binary?
Succ
is the problem. It’s elegant, but not fit for purpose. We know a binary form of Sum
makes Sum
, by some measure, more efficient. We should try and move this binary encoding into the very definition of Nat
.
This becomes extraordinarily simple if you realise the the Succ
encoding is just the unary encoding of integers. It’s just the repetition of a symbol to represent the magnitude of the number, like a tally chart. Usually in unary that symbol is 1
and here it happens to be Succ
.
So we just have to replace Succ
with two wrappers, one representing 0
and one representing 1
for the binary expansion of integers. I chose Zero
and One
respectively.
We have a choice to make though — which direction our encoding runs in. For example, 4 could be One[Zero[Zero[b0]]
or it could be Zero[Zero[One[b0]]]
. We could encode the binary expansion 100
forwards or backwards. (Here b0
is the ‘initial’ binary number representing 0, like Nat._0
represents the initial unary number (also 0)).
If you imagine you were doing a long sum or multiplication by hand, like one of these from primary school:
1783 + 434 -------- ...7
You’ll remember that we operate from the right inwards, from the lowest denomination. For that reason, we should choose a reverse binary expansion for our new Nat
, so all our encodings start at the lowest denomination on the outer layer.
So One[X]
becomes a simple arithmetic operation, and represents the number 2 * X + 1
. Zero[X]
similarly represents the number 2 * X
.
Let’s call our new version BNat
to avoid any confusion.
Here’s what a basic setup might look like:
But before we go any further, we have to ask ourselves something.
Why stop at Binary?
Binary is the natural thing software developers reach for, for obvious reasons. But is it the best choice?
We saw above that we’re trying to minimise the length of the expansion of the number N
when encoded in some flavour of Nat
, some base , unary being base 1 and binary being base 2.
The ‘most efficient’ encoding in these terms is when the base used to represent our N
is infinite — give each number its own unique symbol and suddenly every type is 1 deep and there’s no recursion at all. Solved it!
Obviously that’s absurd. We’re also limiting the number of symbols themselves. That’s what Succ
is optimised for: there is only one symbol and the typeclasses as a result are very simple and easy to reason about.
We’d like to be more balanced. We’re trying to minimise the number of symbols used — our base — while also minimising the length of the number N
in our base. We’re trying to balance having a high base so things compile faster, and keeping the complexity of our typeclasses as low as possible.
So we’re trying to minimise L*B
where L = Length of expansion of N in base B
and B = Chosen base
, with respect to B
.
We know L = log_b(N)
so we have to find the minimum of B * log_b(N)
. This is the same as finding the minimum of B / log(B)
, where log
is the natural logarithm.
Do the maths yourself, plug it into wolfram alpha, whatever you like. Turns out the function is minimised when B = e
, the natural logarithm base, roughly 2.71828...
Which is sadly no good to us, since we definitely can’t operate with a non-integer base in the scala compiler.
Handily though, B=3
gives a lower value in the above function than B=2
. So we’re abandoning binary, and ploughing full steam ahead on to the provably more efficient base ternary.
Ternary Encoding
Same rules apply as binary. We’re going to produce a reverse ternary encoding of the natural numbers using three symbols instead of two this time: Zero
, One
, and Two
.
The arithmetic operations these represent are as follows:
Zero[X] = 3X One[X] = 3X + 1 Two[X] = 3X + 2
Here is the basic setup:
We’re going to be defining Sum
for TNat
, but we need a couple of utilities first. They serve as good examples to see what ternary algorithms look like:
Incr
An easy way of increasing a TNat
by 1
would be nice. In unary’s case this operation was just to wrap a Succ
round it.
Here’s an Incr
implementation for TNat
:
Four cases, telling us how to increment each possible form of a TNat
:
-
incr0
says0 + 1 = 1
-
incrZero
says(3x) + 1 = (3x + 1)
which isOne[x]
-
incrOne
says(3x + 1) + 1 = (3x + 2)
which isTwo[x]
-
incrTwo
says(3x + 2) + 1 = 3(x + 1)
which isZero[Incr[x]]
Hopefully by now such typelevel functions are becoming easy to deconstruct.
Treble
Now we’re working with a more complex structure, there is one thing it makes no sense to express: Zero[t0]
. That’s a different way of just saying t0
— since Zero
represents multiplying by 3. We can’t allow different representations of the same number as it would make any automatic calculation very difficult indeed. So we need a safe way of wrapping a TNat
in Zero
, of trebling it:
and now we can safely apply Zero
to any number we like.
Sum
Sum
is… not nice to implement in ternary. That’s one of the reasons we try to minimise the number of symbols used in the above efficiency calculation. The Sum
typeclass gets really big really fast. There are 11 different cases, compared to Succ
‘s 2 cases.
I prevaricated over whether to include it here or not and in the end decided not to, in case it scared you off. This article is already too long. But it is available for you linked at the end of the article. It’s mostly grunt work anyway, there’s nothing ground-breaking in there. If you understand the approach above in Incr
and Treble
then figuring out Sum
is just elbow grease.
But, it does exist and the definition looks like this:
Let’s take it for a spin.
Benchmarks
Let’s dump some random numbers in to our new addition typeclass and see what falls out.
We will be using the following method to test:
Recall the Succ
encoding could not compile 22+22=44
for us. Well:
So far so good, we’ve broken our previous record! Let’s kick things up to 11:
Those are billions . Compiling in negligible time.
Quintillions? This is in the realm of long overflow:
Negligible. 10⁴⁵?
Negligible! 10¹⁵⁰?
We’ve finally hit something that takes a noticeable amount of time to compile (it also breaks the github syntax highlighter). Two random numbers in the order of 10¹⁵⁰. Succ
was hitting this time calculating 20+5
. Let’s see how far we can push it: 10³⁰⁰?
So we’ve pretty much hit the limit here — 40s is not suitable for use in a project, and any longer and the compiler will start crashing.
10³⁰⁰ though is a 10²⁹⁹ times improvement over Succ
, so it’s a pretty good improvement¹. And it is definitely large enough to encode your string lengths or matrix dimensions, no matter how much data you’re processing.
Recommend
About Joyk
Aggregate valuable and interesting links.
Joyk means Joy of geeK