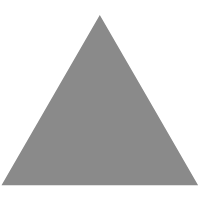
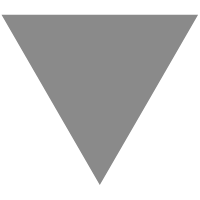
Hello TVM
source link: https://www.tuicool.com/articles/aqIRz2m
Go to the source link to view the article. You can view the picture content, updated content and better typesetting reading experience. If the link is broken, please click the button below to view the snapshot at that time.
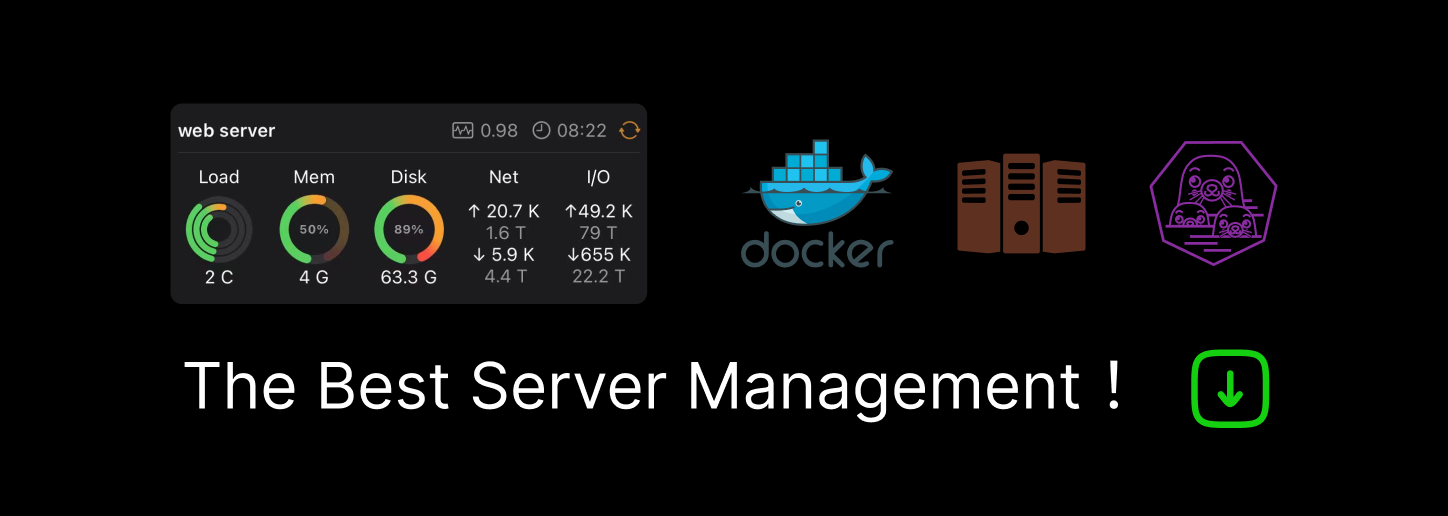
TVM 是什幺?A compiler stack,graph level / operator level optimization,目的是(不同框架的)深度学习模型在不同硬件平台上提高 performance (我要更快!)
TVM, a compiler that takes a high-level specification of a deep learning program from existing frameworks and generates low-level optimized code for a diverse set of hardware back-ends.
compiler比较好理解。C编译器将C代码转换为汇编,再进一步处理成CPU可以理解的机器码。TVM的compiler是指将不同前端深度学习框架训练的模型,转换为统一的中间语言表示。stack我的理解是,TVM还提供了后续处理方法,对IR进行优化(graph / operator level),并转换为目标硬件上的代码逻辑(可能会进行benchmark,反复进行上述优化),从而实现了端到端的深度学习模型部署。
我刚刚接触TVM,这篇主要介绍了如何编译TVM,以及如何使用TVM加载mxnet模型,进行前向计算。Hello TVM!
背景介绍
随着深度学习逐渐从研究所的“伊甸园”迅速在工业界的铺开,摆在大家面前的问题是如何将深度学习模型部署到目标硬件平台上,能够多快好省地完成前向计算,从而提供更好的用户体验,
同时为老板省钱,还能减少碳排放来造福子孙。
和单纯做研究相比,在工业界我们主要遇到了两个问题:
深度学习框架实在是太$^{\text{TM}}$多了。caffe / mxnet / tensorflow / pytorch训练出来的模型都彼此有不同的分发格式。如果你和我一样,做过不同框架的TensorRT的部署,我想你会懂的。。。
GPU实在是太$^{\text{TM}}$贵了。深度学习春风吹满地,老黄股票真争气。另一方面,一些嵌入式平台没有使用GPU的条件。同时一些人也开始在做FPGA/ASIC的深度学习加速卡。如何将深度学习模型部署适配到多样的硬件平台上?
为了解决第一个问题,TVM内部实现了自己的IR,可以将上面这些主流深度学习框架的模型转换为统一的内部表示,以便后续处理。若想要详细了解,可以看下NNVM这篇博客: NNVM Compiler: Open Compiler for AI Frameworks 。这张图应该能够说明NNVM在TVM中起到的作用。
为了解决第二个问题,TVM内部有多重机制来做优化。其中一个特点是,使用机器学习(结合专家知识)的方法,通过在目标硬件上跑大量trial,来获得该硬件上相关运算(例如卷积)的最优实现。这使得TVM能够做到快速为新型硬件或新的op做优化。我们知道,在GPU上我们站在Nvidia内部专家的肩膀上,使用CUDA / CUDNN / CUBLAS编程。但相比于Conv / Pooling等Nvidia已经优化的很好了的op,我们自己写的op很可能效率不高。或者在新的硬件上,没有类似CUDA的生态,如何对网络进行调优?TVM这种基于机器学习的方法给出了一个可行的方案。我们只需给定参数的搜索空间(少量的人类专家知识),就可以将剩下的工作交给TVM。如果对此感兴趣,可以阅读TVM中关于AutoTuner的介绍和tutorial: Auto-tuning a convolutional network for ARM CPU 。
编译
我的环境为Debian 8,CUDA 9。
准备代码
git clone https://github.com/dmlc/tvm.git cd tvm git checkout e22b5802 git submodule update --init --recursive
config文件
cd tvm mkdir build cp ../cmake/config.cmake ./build cd build
编辑config文件,打开CUDA / BLAS / cuBLAS / CUDNN的开关。注意下LLVM的开关。LLVM可以从这个页面 LLVM Download 下载,我之前就已经下载好,版本为7.0。如果你像我一样是Debian8,可以使用for Ubuntu14.04的那个版本。由于是已经编译好的二进制包,下载之后解压即可。
找到这一行,改成
set(USE_LLVM /path/to/llvm/bin/llvm-config)
编译
这里有个坑,因为我们使用了LLVM,最好使用LLVM中的clang。否则可能导致tvm生成的代码无法二次导入。见这个讨论帖: _cc.create_shared error while run tune_simple_template 。
export LLVM=/path/to/llvm cmake -DCMAKE_C_COMPILER=$LLVM/bin/clang -DCMAKE_CXX_COMPILER=$LLVM/bin/clang++ .. # 火力全开,let's rock make -j$(nproc)
python包安装
cd /path/to/tvm # 我一般用清华的镜像,你呢。。。 export THU_MIRROR=https://pypi.tuna.tsinghua.edu.cn/simple pip install tornado tornado psutil xgboost numpy decorator attrs --user -i $THU_MIRROR cd python; python setup.py install --user; cd .. cd topi/python; python setup.py install --user; cd ../.. cd nnvm/python; python setup.py install --user; cd ../..
demo
使用tvm为mxnet symbol计算图生成CUDA代码,并进行前向计算。
import numpy import tvm from tvm import relay from tvm.relay import testing from tvm.contrib import graph_runtime import mxnet as mx ## load mxnet model prefix = '/your/mxnet/checkpoint/prefix' epoch = 0 mx_sym, arg_params, aux_params = mx.model.load_checkpoint(prefix, epoch) ## import model into tvm from mxnet shape_dict = {'data': (1, 3, 224, 224)} ## tvm提供了 frontend.from_XXX 接口,从不同的框架中导入模型 relay_func, relay_params = relay.frontend.from_mxnet(mx_sym, shape_dict, arg_params=arg_params, aux_params=aux_params) # 设定目标硬件为 GPU,生成TVM模型 ## ---------------------------- # graph:execution graph in json format # lib: tvm module library of compiled functions for the graph on the target hardware # params: parameter blobs ## --------------------------- target = 'cuda' with relay.build_config(opt_level=3): graph, lib, params = relay.build(relay_func, target, params=relay_params) # run forward ## 直接使用tvm提供的cat示例图片 from tvm.contrib.download import download_testdata img_url = 'https://github.com/dmlc/mxnet.js/blob/master/data/cat.png?raw=true' img_path = download_testdata(img_url, 'cat.png', module='data') from PIL import Image image = Image.open(img_path).resize((224, 224)) def transform_image(im): im = np.array(im).astype(np.float32) im = np.transpose(im, [2, 0, 1]) im = im[np.newaxis, :] return im x = transform_image(image) # let's go ctx = tvm.gpu(0) dtype = 'float32' ## 加载模型 m = graph_runtime.create(graph, lib, ctx) ## set input data m.set_input('data', tvm.nd.array(x.astype(dtype))) ## set input params m.set_input(**params) m.run() # get output outputs = m.get_output(0) top1 = np.argmax(outputs.asnumpy()[0]) # save model ## lib存为tar包文件,解压后可以发现,就是打包了动态链接库 path_lib = './deploy_resnet50_v2_lib.tar' lib.export_library(path_lib) ## 计算图存为json文件 with open('./deploy_resnet50_v2_graph.json', 'w') as f: f.write(graph) ## 权重存为二进制文件 with open('./deploy_params', 'wb') as f: f.write(relay.save_param_dict(params)) # load model back loaded_json = open('./deploy_resnet50_v2_graph.json').read() loaded_lib = tvm.module.load(path_lib) loaded_params = bytearray(open('./deploy_params', 'rb').read()) module = graph_runtime.create(loaded_json, loaded_lib, ctx) ## 好了,剩下的就都一样了
最后的话
我个人的观点,TVM是一个很有意思的项目。在深度学习模型的优化和部署上做了很多探索,在官方放出的benchmark上表现还是不错的。如果使用非GPU进行模型的部署,TVM值得一试。不过在GPU上,得益于Nvidia的CUDA生态,目前TensorRT仍然用起来更方便,综合性能更好。如果你和我一样,主要仍然在GPU上搞事情,可以密切关注TVM的发展,并尝试使用在自己的项目中,不过我觉得还是优先考虑TensorRT。
Recommend
-
58
Note: The data and scripts here are all stale. Please go to https://github.com/dmlc/tvm/wiki/Benchmark#mobile-gpu For the latest results. Benchmarking De...
-
22
tvd&&tvx&&tvm(Lucene 8.7.0) 在索引(Indexing)阶段,当某个域被设置为需要记录词向量(term vector)信息后,那么随后在
-
8
OpenGL Backend for MXNet/TVM 15-418/618 Parallel Computer Architecture and Programming Zhixun Tan (zhixunt), Peng Wang (pwang1) TVM part:
-
11
Brought to you by 90% of AI / ML applications never make it to market, because fine tuning models for maximum performance across disparate ML software solutions and hardware backends...
-
3
TVM:成为深度学习领域的“Linux”
-
8
TVM export_lib函数分析 发表于 2021-10-08 更新于 2021-10-...
-
9
在TVM中实现Instance Normalization层 0x00 背景 TVM不用多解释了,深度学习领域的编译器,直接从其他框架训练好的模型编译到目标平台上的可执行代码,速度和显存的都有大幅优化,该...
-
9
本文主要分析动态库(.so)文件的加载过程,其中包括模块的反序列化和GraphExecuter 的创建流程。 东台条子泥
-
14
Sisley L. Leads Metaverse of the Sports Market as TVM CEO ...
-
7
tvm dynamic shape 学习探究tvm dynamic shape的实现. tvm ir design
About Joyk
Aggregate valuable and interesting links.
Joyk means Joy of geeK