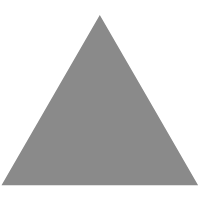
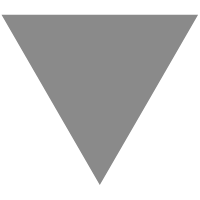
Building K-pop Idol Identifier with Amazon Rekognition
source link: https://www.tuicool.com/articles/IfUBniM
Go to the source link to view the article. You can view the picture content, updated content and better typesetting reading experience. If the link is broken, please click the button below to view the snapshot at that time.
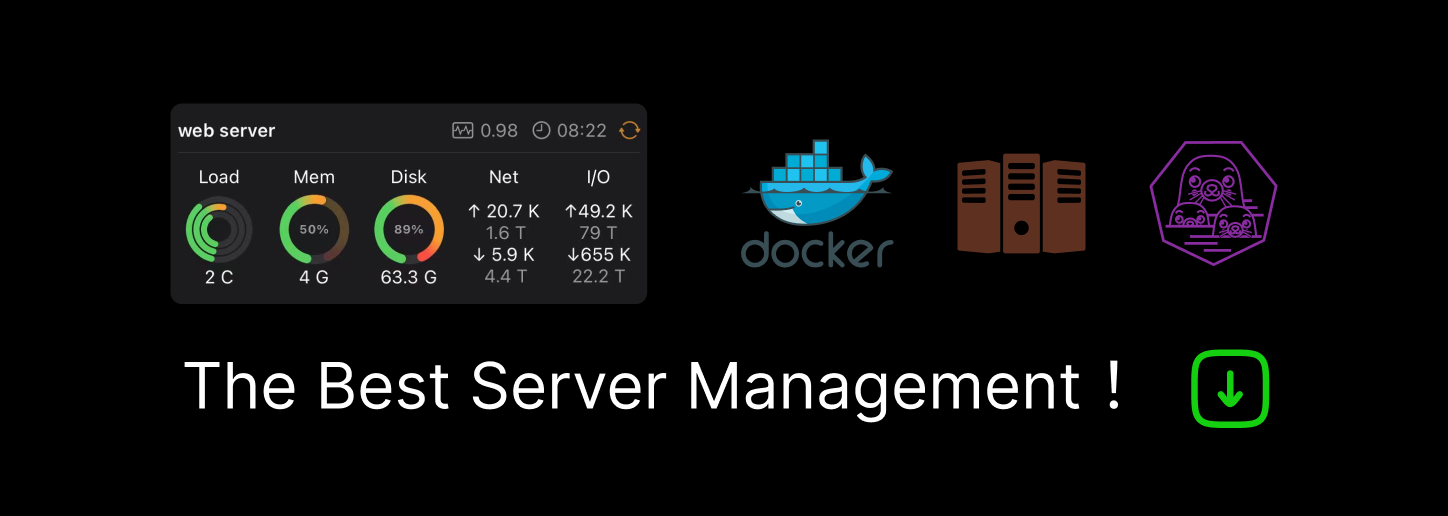


Building a data science model from scratch is quite a big job. There are many elements that make up a single model, many steps involved, and many iterations needed to create a decent model. Even though going through these steps will definitely help you to have a deeper understanding of the algorithm being used in the model, sometimes you just don’t have enough time to go through all the trials and errors especially when you have a tight deadline to meet.
Image recognition is a field in machine learning that has been intensively explored by many tech giants such as Google, Amazon, Microsoft. Among all the features of image processing, probably what’s most being discussed is facial recognition. There are lots of debates on ethical aspects of the technology, but that is beyond the scope of this post. I will simply share what I have tried with Amazon Rekognition, and hope you can get something out of this post.
The urge to write this post started when I played around with Amazon Rekognition demo on their web interface. It provides many useful services like “object and scene detection”, “facial recognition”, “facial analysis”, and “celebrity recognition”. I tried with a few pictures, and everything ran smoothly until I got to “celebrity recognition”. Celebrity recognition first seemed to work fine until I tried with K-pop celebrities’ pictures. The performance of the recognition significantly dropped with K-pop celebrities. Sometimes it gives me the right answer, sometimes it cannot recognise, sometimes it gives me the wrong name.


By the way, the above picture is Tzuyu from a group called Twice, which is my favourite K-pop girl group, and I cannot accept that Amazon recognises this picture as Seolhyun (who’s a member of another group called AOA).
So I decided to write a simple Python script using Amazon Rekognition which can accurately detect the members of Twice.
- In addition to short code blocks you can find in the post, I will attach the link for the whole Jupyter Notebook at the end of this post.
- This post is based on the tutorial “ Build Your Own Face Recognition Service Using Amazon Rekognition ”, but modified from the original code to fit the specific purpose of the project.
Face Detection with Amazon Rekognition
There are a few prerequisites in order for you to run below steps in your Jupyter Notebook.
- Amazon AWS account
- AWS credentials configured with AWS CLI
- The latest version of Boto3
Let’s first start by importing some packages that will be directly used for the next step.
import boto3 from PIL import Image
%matplotlib inline
Now we need to have an image that we want to process. I chose the same image that I tried with the above web interface demo, and we will send this image to Rekognition API to get the result of its image recognition. (The image can also be found in the Github link that I will share at the end of this post.) Let’s take a quick look at the image.
display(Image.open('Tzuyu.jpeg'))


The most basic task we can ask Rekognition is facial recognition with the given image, and this can be done with just a few lines of codes.
import io
rekognition = boto3.client('rekognition')
image = Image.open("Tzuyu.jpeg") stream = io.BytesIO() image.save(stream,format="JPEG") image_binary = stream.getvalue()
rekognition.detect_faces( Image={'Bytes':image_binary}, Attributes=['ALL'] )
You can either send the image to Rekogntion as in-memory binary file object directly from your local machine or upload your image to S3 and give your bucket and key details as a parameter when calling rekognition.detect_faces(). In the above example, I am sending the binary object directly from my local machine. The response you will get from the above call will be quite long with all the information you can get from detect_faces function of Rekognition.
{'FaceDetails': [{'AgeRange': {'High': 38, 'Low': 20}, 'Beard': {'Confidence': 99.98848724365234, 'Value': False}, 'BoundingBox': {'Height': 0.1584049016237259, 'Left': 0.4546355605125427, 'Top': 0.0878104418516159, 'Width': 0.09999311715364456}, 'Confidence': 100.0, 'Emotions': [{'Confidence': 37.66959762573242, 'Type': 'SURPRISED'}, {'Confidence': 29.646778106689453, 'Type': 'CALM'}, {'Confidence': 3.8459930419921875, 'Type': 'SAD'}, {'Confidence': 3.134934186935425, 'Type': 'DISGUSTED'}, {'Confidence': 2.061260938644409, 'Type': 'HAPPY'}, {'Confidence': 18.516468048095703, 'Type': 'CONFUSED'}, {'Confidence': 5.1249613761901855, 'Type': 'ANGRY'}], 'Eyeglasses': {'Confidence': 99.98339080810547, 'Value': False}, 'EyesOpen': {'Confidence': 99.9864730834961, 'Value': True}, 'Gender': {'Confidence': 99.84709167480469, 'Value': 'Female'}, 'Landmarks': [{'Type': 'eyeLeft', 'X': 0.47338899970054626, 'Y': 0.15436244010925293}, {'Type': 'eyeRight', 'X': 0.5152773261070251, 'Y': 0.1474122554063797}, {'Type': 'mouthLeft', 'X': 0.48312342166900635, 'Y': 0.211111381649971}, {'Type': 'mouthRight', 'X': 0.5174261927604675, 'Y': 0.20560002326965332}, {'Type': 'nose', 'X': 0.4872787892818451, 'Y': 0.1808750480413437}, {'Type': 'leftEyeBrowLeft', 'X': 0.45876359939575195, 'Y': 0.14424000680446625}, {'Type': 'leftEyeBrowRight', 'X': 0.4760720133781433, 'Y': 0.13612663745880127}, {'Type': 'leftEyeBrowUp', 'X': 0.4654795229434967, 'Y': 0.13559915125370026}, {'Type': 'rightEyeBrowLeft', 'X': 0.5008187890052795, 'Y': 0.1317606270313263}, {'Type': 'rightEyeBrowRight', 'X': 0.5342025756835938, 'Y': 0.1317359358072281}, {'Type': 'rightEyeBrowUp', 'X': 0.5151524543762207, 'Y': 0.12679456174373627}, {'Type': 'leftEyeLeft', 'X': 0.4674917757511139, 'Y': 0.15510375797748566}, {'Type': 'leftEyeRight', 'X': 0.4817998707294464, 'Y': 0.15343616902828217}, {'Type': 'leftEyeUp', 'X': 0.47253310680389404, 'Y': 0.1514900177717209}, {'Type': 'leftEyeDown', 'X': 0.47370508313179016, 'Y': 0.15651680529117584}, {'Type': 'rightEyeLeft', 'X': 0.5069678425788879, 'Y': 0.14930757880210876}, {'Type': 'rightEyeRight', 'X': 0.5239912867546082, 'Y': 0.1460886150598526}, {'Type': 'rightEyeUp', 'X': 0.5144344568252563, 'Y': 0.1447771191596985}, {'Type': 'rightEyeDown', 'X': 0.5150220394134521, 'Y': 0.14997448027133942}, {'Type': 'noseLeft', 'X': 0.4858757555484772, 'Y': 0.18927086889743805}, {'Type': 'noseRight', 'X': 0.5023624897003174, 'Y': 0.1855706423521042}, {'Type': 'mouthUp', 'X': 0.4945952594280243, 'Y': 0.2002507448196411}, {'Type': 'mouthDown', 'X': 0.4980264902114868, 'Y': 0.21687346696853638}, {'Type': 'leftPupil', 'X': 0.47338899970054626, 'Y': 0.15436244010925293}, {'Type': 'rightPupil', 'X': 0.5152773261070251, 'Y': 0.1474122554063797}, {'Type': 'upperJawlineLeft', 'X': 0.46607205271720886, 'Y': 0.15965013206005096}, {'Type': 'midJawlineLeft', 'X': 0.47901660203933716, 'Y': 0.21797965466976166}, {'Type': 'chinBottom', 'X': 0.5062429904937744, 'Y': 0.24532964825630188}, {'Type': 'midJawlineRight', 'X': 0.5554487109184265, 'Y': 0.20579127967357635}, {'Type': 'upperJawlineRight', 'X': 0.561174750328064, 'Y': 0.14439250528812408}], 'MouthOpen': {'Confidence': 99.0997543334961, 'Value': True}, 'Mustache': {'Confidence': 99.99714660644531, 'Value': False}, 'Pose': {'Pitch': 1.8594770431518555, 'Roll': -11.335309982299805, 'Yaw': -33.68760681152344}, 'Quality': {'Brightness': 89.57070922851562, 'Sharpness': 86.86019134521484}, 'Smile': {'Confidence': 99.23001861572266, 'Value': False}, 'Sunglasses': {'Confidence': 99.99723815917969, 'Value': False}}], 'ResponseMetadata': {'HTTPHeaders': {'connection': 'keep-alive', 'content-length': '3297', 'content-type': 'application/x-amz-json-1.1', 'date': 'Sun, 19 May 2019 08:45:56 GMT', 'x-amzn-requestid': '824f5dc3-7a12-11e9-a384-dfb84e388b7e'}, 'HTTPStatusCode': 200, 'RequestId': '824f5dc3-7a12-11e9-a384-dfb84e388b7e', 'RetryAttempts': 0}}
As you can see from the above example response of the detect_faces call, it has not only bounding box information of the location of the face in the picture but also more advanced features such as emotions, gender, age range, etc.
Comparing Faces
With Amazon Rekognition, you can compare faces in two pictures. For example, if I set a picture of Tzuyu as my source picture, then send a group photo of Twice as my target picture, Rekognition will find the face in the target picture which is the most similar to the source picture. The group photo of Twice I’ll be using is below.


It might be difficult even for humans, especially if you’re not Asian (or not a Twice fan). You can take your guess who is Tzuyu in the picture. As a Korean, and at the same time a Twice fan, I know the answer, but let’s see how well Rekognition can find Tzuyu from this picture.
sourceFile='Tzuyu.jpeg' targetFile='twice_group.jpg' imageSource=open(sourceFile,'rb') imageTarget=open(targetFile,'rb')
response = rekognition.compare_faces(SimilarityThreshold=80, SourceImage={'Bytes': imageSource.read()}, TargetImage={'Bytes': imageTarget.read()}) response['FaceMatches']


The response of the above compare_faces will also output information of all the unmatched faces in the group picture, and this can get quite long, so I’m just outputting the match that Rekognition found by specifying response[‘FaceMatches’]. It seems like a matching face has been found from the group photo with the similarity of around 97%. With the bounding box information, let’s check which face that Rekognition is referring to as Tzuyu’s face.
By the way, the values in the BoundingBox section are ratios of the overall image size. So, in order to draw box with the values in BoundingBox, you need to calculate the location of the box’s each point by multiplying ratios to the actual image height or width. You can find how it can be done in the below code snippet.
from PIL import ImageDraw
image = Image.open("twice_group.jpg") imgWidth,imgHeight = image.size draw = ImageDraw.Draw(image) box = response['FaceMatches'][0]['Face']['BoundingBox'] left = imgWidth * box['Left'] top = imgHeight * box['Top'] width = imgWidth * box['Width'] height = imgHeight * box['Height'] points = ( (left,top), (left + width, top), (left + width, top + height), (left , top + height), (left, top)
) draw.line(points, fill='#00d400', width=2)
display(image)


Yes! Well done, Rekognition! That is Tzuyu indeed!
Creating Collection
Now we can detect face from a picture, and find the most similar face to the source picture from the target picture. But, these are all one-off call, and we need something more to store the information of each member’s face and their name, so that when we send a new picture of Twice, it can retrieve data and detect each member’s face and display their names. In order to implement this, we need to use what Amazon calls “ Storage-Based API Operations ”. There are two Amazon-specific terms for this type of operations. The “collection” is a virtual space where Rekognition stores information about detected faces. With a collection, we can “index” faces, which means to detect faces in an image, then store the information in the specified collection. What’s important is that the information Rekognition stores in the collection is not actual images, but feature vectors extracted by Rekognition’s algorithm. Let’s see how we can create a collection and add indexes.
collectionId='test-collection' rekognition.create_collection(CollectionId=collectionId)


Yes. It is as simple as that. Since this is a new collection we just created, we don’t have any information stored in the collection. But, let’s double check.
rekognition.describe_collection(CollectionId=collectionId)


In the above response, you can see ‘FaceCount’ is 0. This will change if we index any face and store that information in the collection.
Indexing Faces
Indexing faces is again as simple as one line of code with Rekognition.
sourceFile='Tzuyu.jpeg' imageSource=open(sourceFile,'rb')
rekognition.index_faces(Image={'Bytes':imageSource.read()},ExternalImageId='Tzuyu',CollectionId=collectionId)
From the above code, you can see that I am passing ExternalImageId parameter and give it the value of string “Tzuyu”. Later when we try to recognise Tzuyu from a new picture, Rekognition will search for faces that are matching any of the indexed faces. As you will see later, when indexing a face, Rekognition will give it a unique face ID. But I want to display the name “Tzuyu” when a matching face is found from a new picture. For this purpose, I am using ExternalImageId. Now we if we check our collection, we can see 1 face has been added to the collection.
rekognition.describe_collection(CollectionId=collectionId)


Search Faces by Image
Now with Tzuyu’s face indexed in our collection, we can send a new unseen picture to Rekognition and find the matching face. But a problem with search_faces_by_image function is that it can only detect one face (the largest in the image). So if we want to send a group picture of Twice and find Tzuyu from there, we will need to do an additional step. Below we will first detect all the faces in the picture by using detect_faces, then with the bounding box information of each face, we will call search_faces_by_image one by one. First let’s detect each face.
imageSource=open('twice_group.jpg','rb') resp = rekognition.detect_faces(Image={'Bytes':imageSource.read()}) all_faces = resp['FaceDetails'] len(all_faces)

Rekognition detected 9 faces from the group picture. Good. Now let’s crop each face and call serach_faces_by_image one by one.
image = Image.open("twice_group.jpg") image_width,image_height = image.size
for face in all_faces: box=face['BoundingBox'] x1 = box['Left'] * image_width y1 = box['Top'] * image_height x2 = x1 + box['Width'] * image_width y2 = y1 + box['Height'] * image_height image_crop = image.crop((x1,y1,x2,y2)) stream = io.BytesIO() image_crop.save(stream,format="JPEG") image_crop_binary = stream.getvalue()
response = rekognition.search_faces_by_image( CollectionId=collectionId, Image={'Bytes':image_crop_binary} ) print(response) print('-'*100)


Among the 9 search_faces_by_image calls we have made, Rekognition has found one face that matches the indexed face in our collection. We only indexed one face of Tzuyu, so what it has found is Tzuyu’s face from the group picture. Let’s display this on the image with the bounding box and the name. For the name part, we will use the ExternalImageId we set when we indexed the face. By the way, from the search_faces_by_image response, ‘FaceMatches’ part is an array, and if there are more than one matches found from the collection, then it will show all the matches. According to Amazon this array is ordered by similarity score with the highest similarity first. We will get the match with the highest score by specifying the first item of the array.
from PIL import ImageFont import io
image = Image.open("twice_group.jpg") image_width,image_height = image.size for face in all_faces: box=face['BoundingBox'] x1 = box['Left'] * image_width y1 = box['Top'] * image_height x2 = x1 + box['Width'] * image_width y2 = y1 + box['Height'] * image_height image_crop = image.crop((x1,y1,x2,y2)) stream = io.BytesIO() image_crop.save(stream,format="JPEG") image_crop_binary = stream.getvalue()
response = rekognition.search_faces_by_image( CollectionId=collectionId, Image={'Bytes':image_crop_binary} ) if len(response['FaceMatches']) > 0: draw = ImageDraw.Draw(image) points = ( (x1,y1), (x2, y1), (x2, y2), (x1 , y2), (x1, y1)
) draw.line(points, fill='#00d400', width=2) fnt = ImageFont.truetype('/Library/Fonts/Arial.ttf', 15) draw.text((x1,y2),response['FaceMatches'][0]['Face']['ExternalImageId'], font=fnt, fill=(255, 255, 0)) display(image)


Hooray! Again the correct answer!
Identifying All Group Members of Twice
Now let’s expand the project to identify all members from the group picture. In order to do that, we first need to index faces of all members (there are 9 members). I have prepared 4 pictures of each member. I have added multiple pictures of the same person following the logic of Amazon tutorial written by Christian Petters. According to Petters, “adding multiple reference images per person greatly enhances the potential match rate for a person”, which makes intuitive sense. From the Github link I’ll share at the end, you will find all the pictures that are used in this project.
collectionId='twice' rekognition.create_collection(CollectionId=collectionId)


import os
path = 'Twice'
for r, d, f in os.walk(path): for file in f: if file != '.DS_Store': sourceFile = os.path.join(r,file) imageSource=open(sourceFile,'rb') rekognition.index_faces(Image={'Bytes':imageSource.read()},ExternalImageId=file.split('_')[0],CollectionId=collectionId) rekognition.describe_collection(CollectionId=collectionId)


OK. It seems like all 36 pictures are indexed in our “twice” collection. Now it’s time to check the final result. Can Rekognition be enhanced to identify each member of Twice?
from PIL import ImageFont
image = Image.open("twice_group.jpg") image_width,image_height = image.size for face in all_faces: box=face['BoundingBox'] x1 = box['Left'] * image_width y1 = box['Top'] * image_height x2 = x1 + box['Width'] * image_width y2 = y1 + box['Height'] * image_height image_crop = image.crop((x1,y1,x2,y2)) stream = io.BytesIO() image_crop.save(stream,format="JPEG") image_crop_binary = stream.getvalue()
response = rekognition.search_faces_by_image( CollectionId=collectionId, Image={'Bytes':image_crop_binary} ) if len(response['FaceMatches']) > 0: draw = ImageDraw.Draw(image) points = ( (x1,y1), (x2, y1), (x2, y2), (x1 , y2), (x1, y1)
) draw.line(points, fill='#00d400', width=2) fnt = ImageFont.truetype('/Library/Fonts/Arial.ttf', 15) draw.text((x1,y2),response['FaceMatches'][0]['Face']['ExternalImageId'], font=fnt, fill=(255, 255, 0))
display(image)


YES! It can! It identified all the members correctly!
Thank you for reading. You can find the Jupyter Notebook and the pictures used for the project from the below link.
Recommend
-
17
Moderating Image Content in Slack with Amazon Rekognition and Amazon AppFlow | AWS White Paper Summary Jul 24 ...
-
12
AWS Rekognition简介 fendouai...
-
5
李佳琦终成idol,饭圈进攻直播间 脱离主播,玉泽们还能怎么走?
-
11
-
2
Amazon Rekognition Introduces Streaming Video Events May 28, 2022 2...
-
4
The Case of the Golden Idol is a rewarding series of point-and-click murder mysteriesThe Case of the Golden Idol is a rewarding series of point-and-click murder mysteries / The cases can be tough, but they’re...
-
3
The Idol’s latest trailer is a Max-branded bacchanal / Sam Levinson, The Weeknd, and Reza Fahim’s upcoming HBO drama The Idol looks like a twisted portrait of an imperiled pop star in a new teaser.
-
6
The Case of the Golden Idol is coming to Nintendo Switch very soon / The excellent murder mystery puzzler was previously only available on PC and Mac, but you’ll be able to get it on Switch on May 25th.
-
6
The Idol’s latest trailer feels pretty tryhard / Everything about The Idol’s latest trailer makes it seem like the show’s creators might not exactly know how to tell a story about seduction, desire, and power.
-
6
Thousands of Software Engineers Say the Job Market Is Getting Much Worse9,388 engineers polled by Motherboard and Blind said AI will lead to less hiring. Only 6% were confident they'd get another job with the s...
About Joyk
Aggregate valuable and interesting links.
Joyk means Joy of geeK