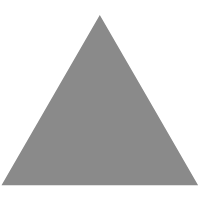
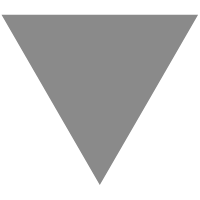
Linear Regression with PyTorch
source link: https://www.tuicool.com/articles/hit/UJZnMjZ
Go to the source link to view the article. You can view the picture content, updated content and better typesetting reading experience. If the link is broken, please click the button below to view the snapshot at that time.
Linear Regression is an approach that tries to find a linear relationship between a dependent variable and an independent variable by minimizing the distance as shown below.


In this post, I’ll show how to implement a simple linear regression model using PyTorch.
Let’s consider a very basic linear equation i.e., y=2x+1 . Here, ‘x’ is the independent variable and y is the dependent variable. We’ll use this equation to create a dummy dataset which will be used to train this linear regression model. Following is the code for creating the dataset.
import numpy as np
# create dummy data for training
x_values = [i for i in range(11)]
x_train = np.array(x_values, dtype=np.float32)
x_train = x_train.reshape(-1, 1)
y_values = [2*i + 1 for i in x_values]
y_train = np.array(y_values, dtype=np.float32)
y_train = y_train.reshape(-1, 1)
Once we have created the dataset, we can start writing the code for our model. First thing will be to define the model architecture. We do that using the following piece of code.
import torch from torch.autograd import Variable
class linearRegression(torch.nn.Module):
def __init__(self, inputSize, outputSize):
super(linearRegression, self).__init__()
self.linear = torch.nn.Linear(inputSize, outputSize)
def forward(self, x):
out = self.linear(x)
return out
We defined a class for linear regression, that inherits torch.nn.Module which is the basic Neural Network module containing all the required functions. Our Linear Regression model only contains one simple linear function.
Next, we instantiate the model using the following code.
inputDim = 1 # takes variable 'x'
outputDim = 1 # takes variable 'y'
learningRate = 0.01
epochs = 100
model = linearRegression(inputDim, outputDim)
##### For GPU #######
if torch.cuda.is_available():
model.cuda()
After that, we initialize the loss ( Mean Squared Error ) and optimization ( Stochastic Gradient Descent ) functions that we’ll use in the training of this model.
criterion = torch.nn.MSELoss() optimizer = torch.optim.SGD(model.parameters(), lr=learningRate)
After completing all the initializations, we can now begin to train our model. Following is the code for training the model.
for epoch in range(epochs):
# Converting inputs and labels to Variable
if torch.cuda.is_available():
inputs = Variable(torch.from_numpy(x_train).cuda())
labels = Variable(torch.from_numpy(y_train).cuda())
else:
inputs = Variable(torch.from_numpy(x_train))
labels = Variable(torch.from_numpy(y_train))
# Clear gradient buffers because we don't want any gradient from previous epoch to carry forward, dont want to cummulate gradients
optimizer.zero_grad()
# get output from the model, given the inputs
outputs = model(inputs)
# get loss for the predicted output
loss = criterion(outputs, labels)
print(loss)
# get gradients w.r.t to parameters
loss.backward()
# update parameters
optimizer.step()
print('epoch {}, loss {}'.format(epoch, loss.item()))
Now that our Linear Regression Model is trained, let’s test it. Since it’s a very trivial model, we’ll test this on our existing dataset and also plot to see the original vs the predicted outputs.
with torch.no_grad(): # we don't need gradients in the testing phase
if torch.cuda.is_available():
predicted = model(Variable(torch.from_numpy(x_train).cuda())).cpu().data.numpy()
else:
predicted = model(Variable(torch.from_numpy(x_train))).data.numpy()
print(predicted)
plt.clf()
plt.plot(x_train, y_train, 'go', label='True data', alpha=0.5)
plt.plot(x_train, predicted, '--', label='Predictions', alpha=0.5)
plt.legend(loc='best')
plt.show()
This plots the following graph.


Looks like our model has correctly figured out the linear relation between our dependent and independent variables.
If you have understood this, you should try and train a linear regression model for a little more complex linear equation with multiple independent variables.
Recommend
About Joyk
Aggregate valuable and interesting links.
Joyk means Joy of geeK