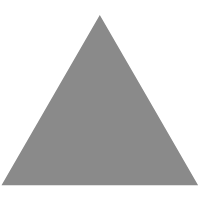
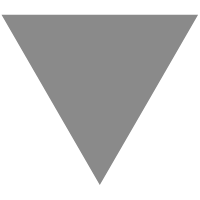
A Gentle Introduction to Channels First and Channels Last Image Formats for Deep...
source link: https://www.tuicool.com/articles/hit/n2yqeue
Go to the source link to view the article. You can view the picture content, updated content and better typesetting reading experience. If the link is broken, please click the button below to view the snapshot at that time.
Color images have height, width, and color channel dimensions.
When represented as three-dimensional arrays, the channel dimension for the image data is last by default, but may be moved to be the first dimension, often for performance-tuning reasons.
The use of these two “ channel ordering formats ” and preparing data to meet a specific preferred channel ordering can be confusing to beginners.
In this tutorial, you will discover channel ordering formats, how to prepare and manipulate image data to meet formats, and how to configure the Keras deep learning library for different channel orderings.
After completing this tutorial, you will know:
- The three-dimensional array structure of images and the channels first and channels last array formats.
- How to add a channels dimension and how to convert images between the channel formats.
- How the Keras deep learning library manages a preferred channel ordering and how to change and query this preference.
Let’s get started.
Tutorial Overview
This tutorial is divided into three parts; they are:
- Images as 3D Arrays
- Manipulating Image Channels
- Keras Channel Ordering
Images as 3D Arrays
An image can be stored as a three-dimensional array in memory.
Typically, the image format has one dimension for rows (height), one for columns (width) and one for channels.
If the image is black and white (grayscale), the channels dimension may not be explicitly present, e.g. there is one unsigned integer pixel value for each (row, column) coordinate in the image.
Colored images typically have three channels, for the pixel value at the (row, column) coordinate for the red, green, and blue components.
Deep learning neural networks require that image data be provided as three-dimensional arrays.
This applies even if your image is grayscale. In this case, the additional dimension for the single color channel must be added.
There are two ways to represent the image data as a three dimensional array. The first involves having the channels as the last or third dimension in the array. This is called “ channels last “. The second involves having the channels as the first dimension in the array, called “ channels first “.
- Channels Last . Image data is represented in a three-dimensional array where the last channel represents the color channels, e.g. [rows][cols][channels] .
- Channels First . Image data is represented in a three-dimensional array where the first channel represents the color channels, e.g. [channels][rows][cols] .
Some image processing and deep learning libraries prefer channels first ordering, and some prefer channels last. As such, it is important to be familiar with the two approaches to representing images.
Want Results with Deep Learning for Computer Vision?
Take my free 7-day email crash course now (with sample code).
Click to sign-up and also get a free PDF Ebook version of the course.
Manipulating Image Channels
You may need to change or manipulate the image channels or channel ordering.
This can be achieved easily using the NumPy python library.
Let’s look at some examples.
In this tutorial, we will use a photograph taken by Larry Koester, some rights reserved, of the Phillip Island Penguin Parade .
Download the image and place it in your current working directory with the filename “ penguin_parade.jpg “.
The code examples in this tutorials assume that the Pillow library is installed.
How to Add a Channel to a Grayscale Image
Grayscale images are loaded as a two-dimensional array.
Before they can be used for modeling, you may have to add an explicit channel dimension to the image. This does not add new data; instead, it changes the array data structure to have an additional third axis with one dimension to hold the grayscale pixel values.
For example, a grayscale image with the dimensions [rows][cols] can be changed to [rows][cols][channels] or [channels][rows][cols] where the new [channels] axis has one dimension.
This can be achieved using the expand_dims() NumPy function . The “ axis ” argument allows you to specify where the new dimension will be added to the first, e.g. first for channels first or last for channels last.
The example below loads the Penguin Parade photograph using the Pillow library as a grayscale image and demonstrates how to add a channel dimension.
# example of expanding dimensions from numpy import expand_dims from numpy import asarray from PIL import Image # load the image img = Image.open('penguin_arade.jpg') # convert the image to grayscale img = img.convert(mode='L') # convert to numpy array data = asarray(img) print(data.shape) # add channels first data_first = expand_dims(data, axis=0) print(data_first.shape) # add channels first data_last = expand_dims(data, axis=2) print(data_last.shape)
Running the example first loads the photograph using the Pillow library, then converts it to a grayscale image.
The image object is converted to a NumPy array and we confirm the shape of the array is two dimensional, specifically (424, 640).
The expand_dims() function is then used to add a channel via axis=0 to the front of the array and the change is confirmed with the shape (1, 424, 640). The same function is then used to add a channel to the end or third dimension of the array with axis=2 and the change is confirmed with the shape (424, 640, 1).
(424, 640) (1, 424, 640) (424, 640, 1)
Another popular alternative to expanding the dimensions of an array is to use the reshape() NumPy function and specify a tuple with the new shape; for example:
data = data.reshape((424, 640, 1))
How to Change Image Channel Ordering
After a color image is loaded as a three-dimensional array, the channel ordering can be changed.
This can be achieved using the moveaxis() NumPy function . It allows you to specify the index of the source axis and the destination axis.
This function can be used to change an array in channel last format such, as [rows][cols][channels] to channels first format, such as [channels][rows][cols] , or the reverse.
The example below loads the Penguin Parade photograph in channel last format and uses the moveaxis() function change it to channels first format.
# change image from channels last to channels first format from numpy import moveaxis from numpy import asarray from PIL import Image # load the color image img = Image.open('penguin_arade.jpg') # convert to numpy array data = asarray(img) print(data.shape) # change channels last to channels first format data = moveaxis(data, 2, 0) print(data.shape) # change channels first to channels last format data = moveaxis(data, 0, 2) print(data.shape)
Running the example first loads the photograph using the Pillow library and converts it to a NumPy array confirming that the image was loaded in channels last format with the shape (424, 640, 3).
The moveaxis() function is then used to move the channels axis from position 2 to position 0 and the result is confirmed showing channels first format (3, 424, 640). This is then reversed, moving the channels in position 0 to position 2 again.
(424, 640, 3) (3, 424, 640) (424, 640, 3)
Keras Channel Ordering
The Keras deep learning library is agnostic to how you wish to represent images in either channel first or last format, but the preference must be specified and adhered to when using the library.
Keras wraps a number of mathematical libraries, and each has a preferred channel ordering. The three main libraries that Keras may wrap and their preferred channel ordering are listed below:
- TensorFlow : Channels last order.
- Theano : Channels first order.
- CNTK : Channels last order.
By default, Keras is configured to use TensorFlow and the channel ordering is also by default channels last. You can use either channel ordering with any library and the Keras library.
Some libraries claim that the preferred channel ordering can result in a large difference in performance. For example, use of the MXNet mathematical library as the backend for Keras recommends using the channels first ordering for better performance.
We strongly recommend changing the image_data_format to channels_first. MXNet is significantly faster on channels_first data.
— Performance Tuning Keras with MXNet Backend, Apache MXNet
Default Channel Ordering
The library and preferred channel ordering are listed in the Keras configuration file, stored in your home directory under ~/.keras/keras.json .
The preferred channel ordering is stored in the “ image_data_format ” configuration setting and can be set as either “ channels_last ” or “ channels_first “.
For example, below is the contents of a keras.json configuration file. In it, you can see that the system is configured to use tensorflow and channels_last order.
{ "image_data_format": "channels_last", "backend": "tensorflow", "epsilon": 1e-07, "floatx": "float32" }
Based on your preferred channel ordering, you will have to prepare your image data to match the preferred ordering.
Specifically, this will include tasks such as:
- Resizing or expanding the dimensions of any training, validation, and test data to meet the expectation.
- Specifying the expected input shape of samples when defining models (e.g. input_shape=(28, 28, 1) ).
Model-Specific Channel Ordering
In addition, those neural network layers that are designed to work with images, such as Conv2D, also provide an argument called “ data_format ” that allows you to specify the channel ordering. For example:
... model.add(Conv2D(..., data_format='channels_first'))
By default, this will use the preferred ordering specified in the “ image_data_format ” value of the Keras configuration file. Nevertheless, you can change the channel order for a given model, and in turn, the datasets and input shape would also have to be changed to use the new channel ordering for the model.
This can be useful when loading a model used for transfer learning that has a channel ordering different to your preferred channel ordering.
Query Channel Ordering
You can confirm your current preferred channel ordering by printing the result of the image_data_format() function. The example below demonstrates.
# show preferred channel order from keras import backend print(backend.image_data_format())
Running the example prints your preferred channel ordering as configured in your Keras configuration file. In this case, the channels last format is used.
channels_last
Accessing this property can be helpful if you want to automatically construct models or prepare data differently depending on the systems preferred channel ordering; for example:
if backend.image_data_format() == 'channels_last': ... else: ...
Force Channel Ordering
Finally, the channel ordering can be forced for a specific program.
This can be achieved by calling the set_image_dim_ordering() function on the Keras backend to either ‘ th ‘ (theano) for channel-first ordering, or ‘ tf ‘ (tensorflow) for channel-last ordering.
This can be useful if you want a program or model to operate consistently regardless of Keras default channel ordering configuration.
# force a channel ordering from keras import backend # force channels-first ordering backend.set_image_dim_ordering('th') print(backend.image_data_format()) # force channels-last ordering backend.set_image_dim_ordering('tf') print(backend.image_data_format())
Running the example first forces channels-first ordering, then channels-last ordering, confirming each configuration by printing the channel ordering mode after the change.
channels_first channels_last
Further Reading
This section provides more resources on the topic if you are looking to go deeper.
- Pillow Python library
- numpy.expand_dims API
- numpy.reshape API
- numpy.moveaxis API
- Keras Backend API
- Keras Convolutional Layers API
Summary
In this tutorial, you discovered channel ordering formats, how to prepare and manipulate image data to meet formats, and how to configure the Keras deep learning library for different channel orderings.
Specifically, you learned:
- The three-dimensional array structure of images and the channels first and channels last array formats.
- How to add a channels dimension and how to convert images between the channel formats.
- How the Keras deep learning library manages a preferred channel ordering and how to change and query this preference.
Do you have any questions?
Ask your questions in the comments below and I will do my best to answer.
Develop Deep Learning Models for Vision Today!
Develop Your Own Vision Models in Minutes
…with just a few lines of python code
Discover how in my new Ebook:
Deep Learning for Computer VisionIt provides self-study tutorials on topics like: classification , object detection (yolo and rcnn) , face recognition (vggface and facenet) , data preparation and much more…
Finally Bring Deep Learning to your Vision Projects
Skip the Academics. Just Results.
Recommend
About Joyk
Aggregate valuable and interesting links.
Joyk means Joy of geeK