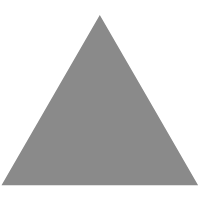
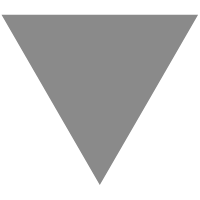
GitHub - SoftwareGift/FeatheNets_Face-Anti-spoofing-Attack-Detection-Challenge-C...
source link: https://github.com/SoftwareGift/FeatheNets_Face-Anti-spoofing-Attack-Detection-Challenge-CVPR2019
Go to the source link to view the article. You can view the picture content, updated content and better typesetting reading experience. If the link is broken, please click the button below to view the snapshot at that time.
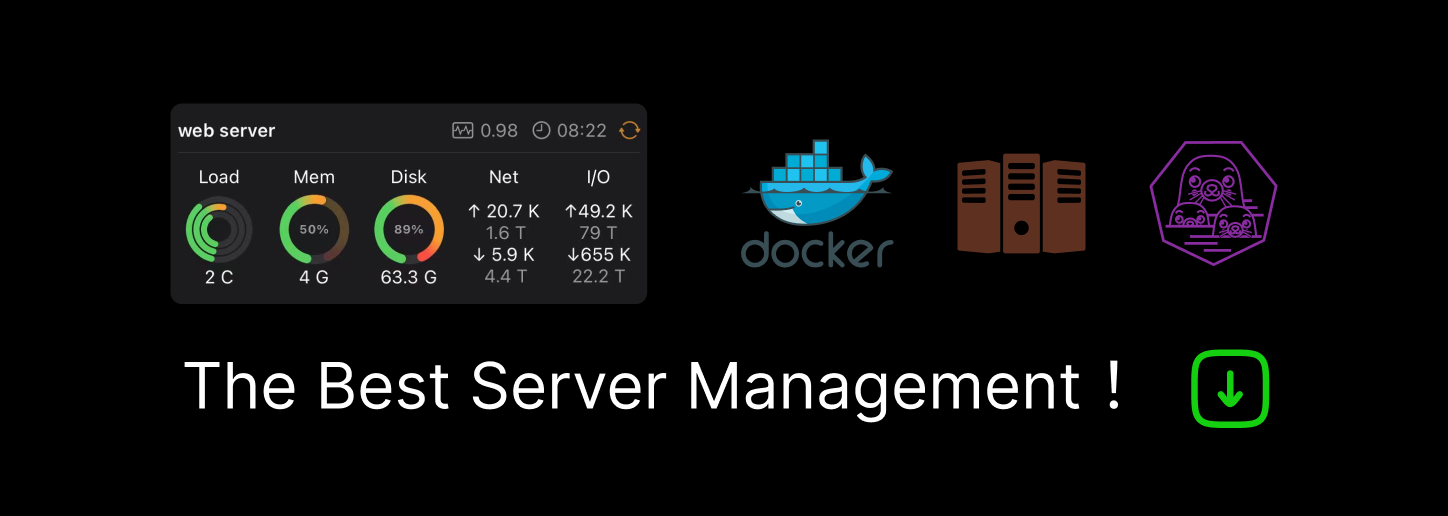
README.md
FeatherNets for Face Anti-spoofing Attack Detection Challenge@CVPR2019[1]
Params only 0.35M!! FLOPs 80M !!
Results on the validation set
model name ACER TPR@FPR=10E-2 TPR@FPR=10E-3 FP FN epoch params FLOPs FishNet150 0.00144 0.999668 0.998330 19 0 27 24.96M 6452.72M FishNet150 0.00181 1.0 0.9996 24 0 52 24.96M 6452.72M FishNet150 0.00496 0.998664 0.990648 48 8 16 24.96M 6452.72M MobileNet v2 0.00228 0.9996 0.9993 28 1 5 2.23M 306.17M MobileNet v2 0.00387 0.999433 0.997662 49 1 6 2.23M 306.17M MobileNet v2 0.00402 0.9996 0.992623 51 1 7 2.23M 306.17M MobileLiteNet54 0.00242 1.0 0.99846 32 0 41 0.57M 270.91M MobileLiteNet54-se 0.00242 1.0 0.996994 32 0 69 0.57M 270.91M FeatherNetA 0.00261 1.00 0.961590 19 7 51 0.35M 79.99M FeatherNetB 0.00168 1.0 0.997662 20 1 48 0.35M 83.05M Ensembled all 0.0000 1.0 1.0 0 0 - - -Prerequisites
install requeirements
conda env create -n env_name -f env.yml
Data
CASIA-SURF Dataset[2]
Our Private Dataset(Available Soon)
Data index tree
├── data
│ ├── our_realsense
│ ├── Training
│ ├── Val
│ ├── Testing
Download and unzip our private Dataset into the ./data directory. Then run data/fileList.py to prepare the file list.
Data Augmentation
Method Settings Random Flip True Random Crop 8% ~ 100% Aspect Ratio 3/4 ~ 4/3 Random PCA Lighting 0.1Train the model
Download pretrained models
download fishnet150 pretrained model from FishNet150 repo(Model trained without tricks )
download mobilenetv2 pretrained model from MobileNet V2 repo
move them to ./checkpoints/pre-trainedModels/
1.train FishNet150
nohup python main.py --config="cfgs/fishnet150-32.yaml" --b 32 --lr 0.01 --every-decay 30 --fl-gamma 2 >> fishnet150-train.log &
2.train MobileNet V2
nohup python main.py --config="cfgs/mobilenetv2.yaml" --b 32 --lr 0.01 --every-decay 40 --fl-gamma 2 >> mobilenetv2-bs32-train.log &
Commands to train the model:
3Train MobileLiteNet54
python main.py --config="cfgs/MobileLiteNet54-32.yaml" --every-decay 60 -b 32 --lr 0.01 --fl-gamma 3 >>FNet54-bs32-train.log
4Train MobileLiteNet54-SE
python main.py --config="cfgs/MobileLiteNet54-se-64.yaml" --b 64 --lr 0.01 --every-decay 60 --fl-gamma 3 >> FNet54-se-bs64-train.log
5Train FeatherNetA
python main.py --config="cfgs/FeatherNetA-32.yaml" --b 32 --lr 0.01 --every-decay 60 --fl-gamma 3 >> MobileLiteNetA-bs32-train.log
6Train FeatherNetB
python main.py --config="cfgs/FeatherNetB-32.yaml" --b 32 --lr 0.01 --every-decay 60 --fl-gamma 3 >> MobileLiteNetB-bs32--train.log
How to create a submission file
example:
python main.py --config="cfgs/mobilenetv2.yaml" --resume ./checkpoints/mobilenetv2_bs32/_4_best.pth.tar --val True --val-save True
Ensemble
for validation
run EnsembledCode_val.ipynb
for test
run EnsembledCode_test.ipynb
notice:Choose a few models with large differences in prediction results
Serialized copy of the trained model
You can download my artifacts folder which I used to generate my final submissions: Available Soon
[1] ChaLearn Face Anti-spoofing Attack Detection Challenge@CVPR2019,link
[2] Shifeng Zhang, Xiaobo Wang, Ajian Liu, Chenxu Zhao, Jun Wan, Sergio Escalera, Hailin Shi, Zezheng Wang, Stan Z. Li, " CASIA-SURF: A Dataset and Benchmark for Large-scale Multi-modal Face Anti-spoofing ", arXiv, 2018 PDF
Recommend
About Joyk
Aggregate valuable and interesting links.
Joyk means Joy of geeK