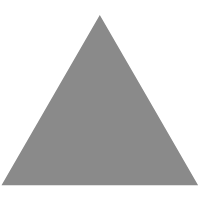
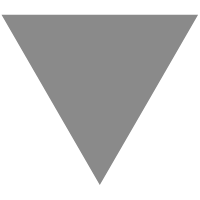
On the poor performance of classifiers in insurance models
source link: https://www.tuicool.com/articles/hit/NBJfYna
Go to the source link to view the article. You can view the picture content, updated content and better typesetting reading experience. If the link is broken, please click the button below to view the snapshot at that time.
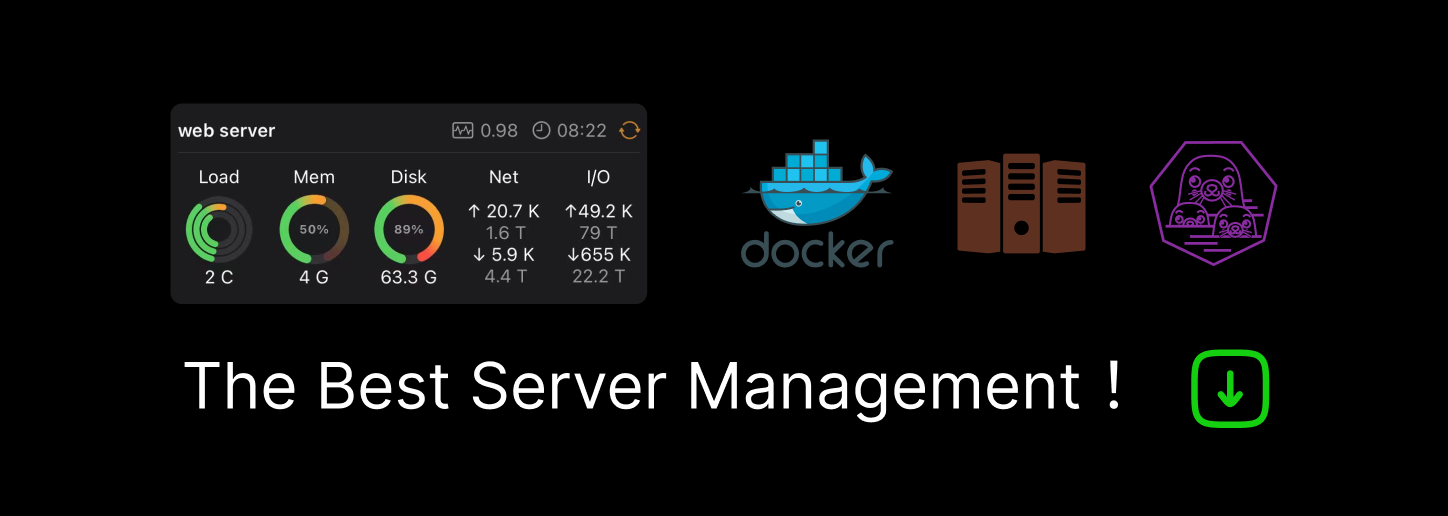
(This article was first published on R-english – Freakonometrics , and kindly contributed toR-bloggers)
Each time we have a case study in my actuarial courses (with real data), students are surprised to have hard time getting a “good” model, and they are always surprised to have a low AUC , when trying to model the probability to claim a loss, to die, to fraud, etc. And each time, I keep saying, “yes, I know, and that’s what we expect because there a lot of ‘randomness’ in insurance”. To be more specific, I decided to run some simulations, and to compute AUCs to see what’s going on. And because I don’t want to waste time fitting models, we will assume that we have each time a perfect model. So I want to show that the upper bound of the AUC is actually quite low ! So it’s not a modeling issue, it is a fondamental issue in insurance !
By ‘perfect model’ I mean the following :














). That’s the idea with randomness, right ?
So, here
denote the probabilities to claim a loss, to die, to fraud, etc. There is heterogeneity here, and this heterogenity can be small, or large. Consider the graph below, to illustrate,
In both cases, there is, on average, 25% chance to claim a loss. But on the left, there is more heterogeneity, more dispersion. To illustrate, I used the arrow, which is a classical 90% interval : 90% of the individuals have a probability to claim a loss in that interval. (here 10%-40%), 5% are below 10% (low risk), and 5% are above 40% (high risk). Later on, we will say that we have 25% on average, with a dispersion of 30% (40% minus 10%). On the right, it’s more 25% on average, with a dispersion of of 15%. What I call dispersion is the difference between the 95% and the 5% quantiles.
Consider now some dataset, with Bernoulli variables

. Then, let us assume that we are able to get a perfect model : I do not estimate a model based on some covariates, here, I assume that I know perfectly the probability (which is true, because I did generate those data). More specifically, to generate a vector of probabilities, here I use a Beta distribution with a given mean, and a given variance (to capture the heterogeneity I mentioned above)
a=m*(m*(1-m)/v-1) b=(1-m)*(m*(1-m)/v-1) p=rbeta(n,a,b)
from those probabilities, I generate occurences of claims, or deaths,
Y=rbinom(n,size = 1,prob = p)
Then, I compute the AUC of my “perfect” model,
auc.tmp=performance(prediction(p,Y),"auc")
And then, I will generate many samples, to compute the average value of the AUC. And actually, we can do that for many values of the mean and the variance of the Beta distribution. Here is the code
library(ROCR) n=1000 ns=200 ab_beta = function(m,inter){ a=uniroot(function(a) qbeta(.95,a,a/m-a)-qbeta(.05,a,a/m-a)-inter, interval=c(.0000001,1000000))$root b=a/m-a return(c(a,b)) } Sim_AUC_mean_inter=function(m=.5,i=.05){ V_auc=rep(NA,ns) b=-1 essai = try(ab<-ab_beta(m,i),TRUE) if(inherits(essai,what="try-error")) a=-1 if(!inherits(essai,what="try-error")){ a=ab[1] b=ab[2] } if((a>=0)&(b>=0)){ for(s in 1:ns){ p=rbeta(n,a,b) Y=rbinom(n,size = 1,prob = p) auc.tmp=performance(prediction(p,Y),"auc") V_auc[s]=as.numeric([email protected])} L=list(moy_beta=m, var_beat=v, q05=qbeta(.05,a,b), q95=qbeta(.95,a,b), moy_AUC=mean(V_auc), sd_AUC=sd(V_auc), q05_AUC=quantile(V_auc,.05), q95_AUC=quantile(V_auc,.95)) return(L)} if((a<0)|(b<0)){return(list(moy_AUC=NA))}} Vm=seq(.025,.975,by=.025) Vi=seq(.01,.5,by=.01) V=outer(X = Vm,Y = Vi, Vectorize(function(x,y) Sim_AUC_mean_inter(x,y)$moy_AUC)) library("RColorBrewer") image(Vm,Vi,V, xlab="Probability (Average)", ylab="Dispersion (Q95-Q5)", col= colorRampPalette(brewer.pal(n = 9, name = "YlGn"))(101)) contour(Vm,Vi,V,add=TRUE,lwd=2)
On the x -axis, we have the average probability to claim a loss. Of course, there is a symmetry here. And on the y -axis, we have the dispersion : the lower, the less heterogeneity in the portfolio. For instance, with a 30% chance to claim a loss on average, and 20% dispersion (meaning that in the portfolio, 90% of the insured have between 20% and 40% chance to claim a loss, or 15% and 35% chance), we have on average a 60% AUC. With a perfect model ! So with only a few covariates, having 55% should be great !
My point here is that with a low dispersion, we cannot expect to have a great AUC (again, even with a perfect model). In motor insurance, from my experience, 90% of the insured are between 3% chance and 20% chance to claim a loss ! That’s less than 20% dispersion ! and in that case, even if the (average) probability is rather small, it is very difficult to expect an AUC above 60% or 65% !
Recommend
-
36
README.md Adversarial Robustness Toolbox (ART v0.1)
-
41
Learn how to create a training project, add classification tags, upload images, train the project, obtain the endpoint URL, and use that to test an image.
-
35
Coastal classifiers: using AutoML Vision to assess and track environmental...
-
45
This article covers how to detect data drift for models that ingest image data as their input in order to prevent their silent degradation in production. Run the example in a complementaryDomino project. Intr...
-
12
Degrees and certs are poor indicators of performance I just love certifications and degrees. They make excellent kindling. Back when I was working for a school district, one of the "tech people" at a certain elementary sch...
-
8
MachineX: SVM as Non-Linear Classifiers Reading Time: 3 minutes In our previous blogs, we have already looked and had a hi...
-
10
MC387035: Microsoft Purview: Additional classifiers for Communication Compliance (preview) Coming soon to public preview, we’re rolling out several new classifiers for Communication Compliance to assist you in detectin...
-
6
Project: Create An App That Allows Anyone To Build Image Classifiers On Phone Projects ...
-
7
New insights into training dynamics of deep classifiers MIT researchers uncover the st...
-
4
The K-Nearest Neighbors (K-NN) algorithm is a popular Machine Learning algorithm used mostly for solving classification problems. In this article, you'll learn how the K-NN algorithm works with practical examples.We'll use diagrams,...
About Joyk
Aggregate valuable and interesting links.
Joyk means Joy of geeK