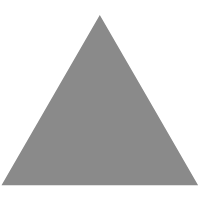
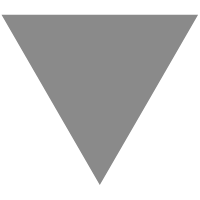
使用Python和Mask R-CNN自动寻找停车位,这是什么神操作?
source link: https://www.jiqizhixin.com/articles/2019-02-05?amp%3Butm_medium=referral
Go to the source link to view the article. You can view the picture content, updated content and better typesetting reading experience. If the link is broken, please click the button below to view the snapshot at that time.
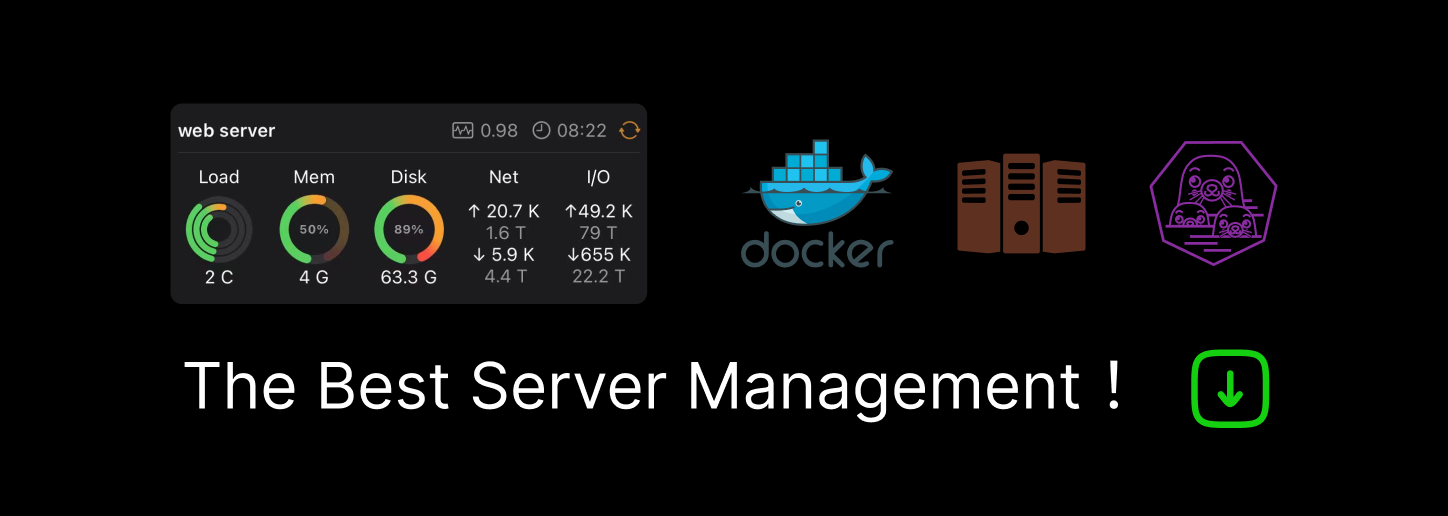
我居住在一个大城市。但是和在很多大城市一样,找个停车位总没那么容易。车位很快就被抢占一空,即使你有一个属于自己的专用车位,朋友们顺路来访也很难,因为他们找不到车位。
我的解决方案就是将一个摄像头伸出窗外,再用深度学习让我的计算机在有车位空出来的时候给我发短信:
这听起来也许很复杂,但是借助深度学习构建一个实用的版本实际上又简单又快。所有的工具都是可用的——你只要知道去哪儿找到工具并把它们组合在一起就行。
那么,我们花点时间用 python 和深度学习构建一个高准确率的停车位通知系统吧!
问题分解
当我们面临想要用机器学习解决的复杂问题时,第一步就是将问题分解成若干简单问题。然后,使用详细分解作为指导,我们可以从机器学习工具箱中使用不同的工具来解决这些小问题。通过将几个不同的解决方案链接到一个流程中,我们就得到了能够做一些复杂事情的系统。
下面是我分解的空车位检测流程:
机器学习流程的输入是来自一个伸出窗外的普通网络摄像头的视频流:
从摄像头中截取的示例视频
我们将通过工作流程传送每一帧视频,一次一帧。
这个流程的第一步就是检测一帧视频中所有可能的停车位。显然,在我们能够检测哪个是没有被占用的停车位之前,我们需要知道图像中的哪些部分是停车位。
第二步就是检测每帧视频中的所有车辆。这样我们可以逐帧跟踪每辆车的运动。
第三步就是确定哪些车位目前是被占用的,哪些没有。这需要结合前两步的结果。
最后一步就是出现新车位时通知我。这需要基于视频中两帧之间车辆位置的变化。
这里的每一步,我们都可以使用多种技术用很多种方式实现。构建这个流程并没有唯一正确或者错误的方式,但不同的方法会有优劣之分。
第一步:检测一幅图像中的停车位
摄像头的视野是这样的:
我们需要扫描这幅图,然后返回一个有效停车区域列表,就像这样:
这个城市街道上的有效停车位。
一种比较懒的方法就是手动把每个停车位的位置硬编码到程序中,而不是自动检测停车位。但是如果我们移动了摄像头或者想要检测另一条街道上的车位时,就必须再一次手动硬编码车位的位置。这样很麻烦,还是找一种自动检测车位的方法吧。
一个思路是寻找停车计时器并假设每个计时器旁边都有一个停车位:
检测每幅图像中的停车计时器。
但这种方法有些复杂。首先,并非每个停车位都有停车计时器——实际上,我们最喜欢找的是无需付费的车位!其次,只知道停车计时器的位置并不能确切地告诉我们停车位的确切位置。它只是让我们更加接近停车位罢了。
另一个思路就是构建目标检测模型,让它寻找道路上绘制的停车位标志,就像这样:
请注意这些黄色的小标志——它们就是画在道路上的每个车位的边界。
但是这种方法也很令人痛苦。首先,我所在城市的停车位标志线特别小,在这么远的距离很难看见,所以很难用计算机检测到它们。其次,道路上有各种无关的线和标志。很难区分哪些线是停车位标志,哪些是车道分离线或者人行道线。
当你遇到似乎很困难的问题时,花几分钟时间想一想,你是否可以采用不同的方法来解决这个问题,避开一些技术性挑战。到底什么是停车位?停车位不就是车辆可以停放很长时间的地方吗。所以,也许我们根本就没必要去检测停车位。为何不能仅仅检测长时间没有移动的车辆并且假设它们就停在停车位呢?
换句话说,有效停车位就是包含非移动车辆的地方。
这里,每辆车的边界框实际上就是一个停车位!如果我们能够检测静态的车辆,就没必要检测停车位。
所以,如果我们能够检测车辆,并且可以判断哪些车辆在视频帧中是没有移动的,那我们就能够推测出停车位的位置。够简单了——让我们来检测车辆吧!
检测图像中的车辆
检测视频帧里的车辆就是目标检测中的一道练习题。我们可以用很多机器学习方法来检测图像中的目标。下面是我列出的几种最常用的目标检测算法:
-
训练一个 HOG(方向梯度直方图) 目标检测器,并用它滑过我们的图像以寻找所有的车辆。这个古老的非深度学习方法运行起来相当快,但是它并不能很好地处理向不同方向移动的车辆。
-
训练一个 CNN(卷积神经网络) 目标检测器,用它滑过我们的图像直到找到所有的车辆。这种方法很准确,但并不是很高效,因为我们必须多次扫描同一张图像来寻找所有的车辆。并且,虽然它可以轻易找到向不同方向移动的车辆,但它需要的训练数据要比 HOG 目标检测器多得多。
-
使用更新的深度学习方法,如Mask R-CNN、Faster R-CNN 或者YOLO。它们将灵活的设计和高效的技巧与 CNN 的准确性结合在了一起,能够极大地加速检测过程。只要我们有足够多的数据来训练模型,它能在 GPU 上运行地相对快一些。
通常情况下,我们希望选择最简单的方法来解决问题,使用最少的训练数据,并不认为需要最新、最流行的算法。但是在这个特殊的情况下,*Mask R-CNN*是一个比较合理的选择,虽然它是一个比较新、比较流行的算法。
Mask R-CNN架构在不使用滑动窗口的情况下以一种高效的计算方式在整幅图像中检测目标。换句话说,它运行得相当快。在具有比较先进的 GPU 时,我们应该能够以数帧每秒的速度检测到高分辨率视频中的目标。所以它应该比较适合这个项目。
此外,Mask R-CNN给我们提供了很多关于每个检测对象的信息。绝大多数目标检测算法仅仅返回了每个对象的边界框。但是Mask R-CNN并不会仅仅给我们提供每个对象的位置,它还会给出每个对象的轮廓 (掩模),就像这样:
为了训练Mask R-CNN,我们需要大量关于需要检测的目标的图像。我们可以拍一些车辆的图像,然后将这些图像中的汽车标注出来,但是这可能会花费几天的工作。幸运的是,汽车是很常见的检测目标,很多人都想检测,因此早就有了几个公开的汽车数据集。
有一个很流行的数据集叫做 COCO,它里面的图像都用目标掩膜标注过。在这个数据集中,已经有超过 12000 张汽车图像做好了轮廓标注。下面就是 COCO 数据集中的一张图像。
COCO 数据集中已标注轮廓的图像。
这个数据集非常适合用来训练Mask R-CNN模型。
使用 COCO 数据集来构建目标检测数据集是很常见的一件事情,所以好多人已经做过并且分享了他们的结果。因此,我们可以用一个训练好的模型作为开始,而不用从头去训练自己的模型。针对这个项目,我们可以使用很棒的开源Mask R-CNN,它是由 Matterport 公司实现的,还提供了训练好的模型。
地址:https://github.com/matterport/Mask_RCNN
旁注:你不必为训练定制化的Mask R-CNN而担心!标注数据是很耗时间的,但是并不困难。如果你想使用自己的数据完整地训练Mask R-CNN模型,可以参考这本书:
https://www.machinelearningisfun.com/get-the-book
如果在我自己的相机图像上运行预训练模型,以下是检测结果:
经过 COCO 默认目标识别的图像——车辆、人、交通信号灯和树。
我们不仅识别了车辆,还识别出了交通信号灯和人。而且比较滑稽的是,它将其中的一棵树识别成了「盆栽植物」。
对于图像中被检测到的每一个目标,我们从Mask R-CNN模型中得到了下面四个结果:
-
被检测到的目标(作为整数)类型。预训练的 COCO 模型知道如何检测 80 种不同的常见目标,例如汽车和卡车。
-
目标检测的置信得分。这个数字越大,越说明模型准确识别了目标。
-
中目标的边界框,以 X/Y 像素位置地形式给了出来。
-
位图「掩模」,能够分辨出边界框里哪些像素是目标的一部分,哪些不是。有了掩模数据,我们也可以标注目标的轮廓。
下面是 python 代码,用于根据 Matterport』sMask R-CNN实现和 OpneCV 预训练的模型来检测汽车边界框:
import os import numpy as np import cv2 import mrcnn.config import mrcnn.utils from mrcnn.model import MaskRCNN from pathlib import Path # Configuration that will be used by the Mask-RCNN library class MaskRCNNConfig(mrcnn.config.Config): NAME = "coco_pretrained_model_config" IMAGES_PER_GPU = 1 GPU_COUNT = 1 NUM_CLASSES = 1 + 80 # COCO dataset has 80 classes + one background class DETECTION_MIN_CONFIDENCE = 0.6 # Filter a list of Mask R-CNN detection results to get only the detected cars / trucks def get_car_boxes(boxes, class_ids): car_boxes = [] for i, box in enumerate(boxes): # If the detected object isn't a car / truck, skip it if class_ids[i] in [3, 8, 6]: car_boxes.append(box) return np.array(car_boxes) # Root directory of the project ROOT_DIR = Path(".") # Directory to save logs and trained model MODEL_DIR = os.path.join(ROOT_DIR, "logs") # Local path to trained weights file COCO_MODEL_PATH = os.path.join(ROOT_DIR, "mask_rcnn_coco.h5") # Download COCO trained weights from Releases if needed if not os.path.exists(COCO_MODEL_PATH): mrcnn.utils.download_trained_weights(COCO_MODEL_PATH) # Directory of images to run detection on IMAGE_DIR = os.path.join(ROOT_DIR, "images") # Video file or camera to process - set this to 0 to use your webcam instead of a video file VIDEO_SOURCE = "test_images/parking.mp4" # Create a Mask-RCNN model in inference mode model = MaskRCNN(mode="inference", model_dir=MODEL_DIR, config=MaskRCNNConfig()) # Load pre-trained model model.load_weights(COCO_MODEL_PATH, by_name=True) # Location of parking spaces parked_car_boxes = None # Load the video file we want to run detection on video_capture = cv2.VideoCapture(VIDEO_SOURCE) # Loop over each frame of video while video_capture.isOpened(): success, frame = video_capture.read() if not success: break # Convert the image from BGR color (which <mark data-type="technologies" data-id="9c38872b-5720-41cc-84ed-debc155db10c">OpenCV</mark> uses) to RGB color rgb_image = frame[:, :, ::-1] # Run the image through the Mask R-CNN model to get results. results = model.detect([rgb_image], verbose=0) # Mask R-CNN assumes we are running detection on multiple images. # We only passed in one image to detect, so only grab the first result. r = results[0] # The r variable will now have the results of detection: # - r['rois'] are the bounding box of each detected object # - r['class_ids'] are the class id (type) of each detected object # - r['scores'] are the confidence scores for each detection # - r['masks'] are the object masks for each detected object (which gives you the object outline) # Filter the results to only grab the car / truck bounding boxes car_boxes = get_car_boxes(r['rois'], r['class_ids']) print("Cars found in frame of video:") # Draw each box on the frame for box in car_boxes: print("Car: ", box) y1, x1, y2, x2 = box # Draw the box cv2.rectangle(frame, (x1, y1), (x2, y2), (0, 255, 0), 1) # Show the frame of video on the screen cv2.imshow('Video', frame) # Hit 'q' to quit if cv2.waitKey(1) & 0xFF == ord('q'): break # Clean up everything when finished video_capture.release() cv2.destroyAllWindows()
当你运行这段脚本时,会在屏幕上得到一幅图,每辆检测到的汽车都有一个边界框,像这样:
每辆检测到的汽车都有一个绿色的边界框。
你还会看到被检测到的汽车坐标被打印在了控制台上,就像这样:
Cars found in frame of video: Car: [492 871 551 961] Car: [450 819 509 913] Car: [411 774 470 856]
经过以上这些步骤,我们已经成功地检测到了图像中的汽车。
检测空置的停车位
我们知道了每张图像中每辆车的像素位置。通过查看视频中按顺序出现的多帧,我们可以轻易知道哪些车没有动,并且假设它们所在的位置就是车位。但是,当一辆车离开车位的时候,我们如何检测得到呢?
问题在于我们图像中的边界框是部分重叠的。
即使是在不同车位中的车辆,每辆车的边界框都会有一小部分的重叠。
所以,如果我们假设每个边界框代表一个车位,那么,即使车位是空的,也有可能显示为被部分占用。我们需要一个方法来测量两个对象的重叠度,以便检查「大部分是空的」边界框。
我们将要使用的测量方法为交并比(IoU)。IoU 通过两个对象重叠的像素数量除以两个对象覆盖的像素数量计算得到。像这样:
这将为我们提供汽车边界框与停车位边界框重叠的程度。有了这个,我们可以轻易确定汽车是否在停车位。如果 IoU 测量值很低,如 0.15,那意味着汽车并没有真正占用大部分停车位。但如果指标很高,如 0.6,这意味着汽车占据了大部分停车位区域,因此我们可以确定该空间被占用。
由于 IoU 是计算机视觉中常见的测量方法,因此你在使用的库通常已经实现了它的计算。事实上,MatterportMask R-CNN库将它作为一个名为 mrcnn.utils.compute_overlaps()的函数包含在内,因此我们可以直接使用该函数。
假设我们有一个表示图像中停车区域的边界框列表,查看检测到的车辆是否在这些边界框内就像添加一行或两行代码一样简单:
# Filter the results to only grab the car / truck bounding boxes car_boxes = get_car_boxes(r['rois'], r['class_ids']) # See how much cars overlap with the known parking spaces overlaps = mrcnn.utils.compute_overlaps(car_boxes, parking_areas) print(overlaps)
结果是这样子的:
[ [1. 0.07040032 0. 0.] [0.07040032 1. 0.07673165 0.] [0. 0. 0.02332112 0.] ] In that 2D array, each
在这个二维数组中,每一行代表一个停车位的边界框。同样,每一列代表着这个停车位被检测到的汽车占用了多少。1.0 分表示完全被占用,较低的分,如 0.02 则表示这辆汽车接触到了车位的空间,但是并没有占据大部分区域。
要寻找未被占用的停车位,我们只需要检查此阵列中的每一行。如果所有数字都为零或非常小,那意味着没有任何东西占据那个空间,它就是空着的!
但请记住,目标检测并非总是与实时视频完美配合。即使Mask R-CNN非常准确,偶尔也会在单帧视频中错过一两辆车。因此,在将停车位标记为空闲之前,我们应该确保它在一段时间内保持空闲 - 可能是 5 或 10 个连续的视频帧。这将防止系统仅仅因为目标检测在一帧视频上有短暂的停顿就错误地检测到空闲的停车位。但是,只要我们看到至少有一个空闲停车位出现在连续几帧视频中,我们就可以发送短信了!
发送短信
这个项目的最后一步就是当检测到一个空闲停车位出现在视频的连续几帧中时就发送短信提醒。
使用 Twilio 从 Python 中发送短信很简单。Twilio 是一个很流行的 API,它可以让你用任何编程语言只需几行代码就可以发送短信。当然,如果你更喜欢使用其它短信服务提供商,也可以。我和 Twilio 并没有利益关系。它只是我想到的第一个工具而已。
要使用 Twilio,你需要注册一个试用账户,创建两个 Twilio 电话号码,然后认证账户。然后,你需要安装 Twilio Python 客户端。
pip3 install twilio
安装完成后,这是用 Python 发送短信的完整代码(只需用你自己的帐户详细信息替换这些值即可):
from twilio.rest import Client # Twilio account details twilio_account_sid = 'Your Twilio SID here' twilio_auth_token = 'Your Twilio Auth Token here' twilio_source_phone_number = 'Your Twilio phone number here' # Create a Twilio client object instance client = Client(twilio_account_sid, twilio_auth_token) # Send an SMS message = client.messages.create( body="This is my SMS message!", from_=twilio_source_phone_number, to="Destination phone number here" )
为了在脚本中添加短信发送功能,我们可以把这些代码丢进去。但需要注意的是,我们并不需要在每一个有空闲车位的新视频帧中发送短信。所以我们需要一个标志来跟踪是否已经发过短信了,这是为了保证不会在短期内再次发送或者在新车位空出来之前再次发送。
总结
将以上流程中的所有步骤整合在一起,构成一个独立的 Python 脚本,完整代码如下所示:
import os import numpy as np import cv2 import mrcnn.config import mrcnn.utils from mrcnn.model import MaskRCNN from pathlib import Path from twilio.rest import Client # Configuration that will be used by the Mask-RCNN library class MaskRCNNConfig(mrcnn.config.Config): NAME = "coco_pretrained_model_config" IMAGES_PER_GPU = 1 GPU_COUNT = 1 NUM_CLASSES = 1 + 80 # COCO dataset has 80 classes + one background class DETECTION_MIN_CONFIDENCE = 0.6 # Filter a list of Mask R-CNN detection results to get only the detected cars / trucks def get_car_boxes(boxes, class_ids): car_boxes = [] for i, box in enumerate(boxes): # If the detected object isn't a car / truck, skip it if class_ids[i] in [3, 8, 6]: car_boxes.append(box) return np.array(car_boxes) # Twilio config twilio_account_sid = 'YOUR_TWILIO_SID' twilio_auth_token = 'YOUR_TWILIO_AUTH_TOKEN' twilio_phone_number = 'YOUR_TWILIO_SOURCE_PHONE_NUMBER' destination_phone_number = 'THE_PHONE_NUMBER_TO_TEXT' client = Client(twilio_account_sid, twilio_auth_token) # Root directory of the project ROOT_DIR = Path(".") # Directory to save logs and trained model MODEL_DIR = os.path.join(ROOT_DIR, "logs") # Local path to trained weights file COCO_MODEL_PATH = os.path.join(ROOT_DIR, "mask_rcnn_coco.h5") # Download COCO trained weights from Releases if needed if not os.path.exists(COCO_MODEL_PATH): mrcnn.utils.download_trained_weights(COCO_MODEL_PATH) # Directory of images to run detection on IMAGE_DIR = os.path.join(ROOT_DIR, "images") # Video file or camera to process - set this to 0 to use your webcam instead of a video file VIDEO_SOURCE = "test_images/parking.mp4" # Create a Mask-RCNN model in inference mode model = MaskRCNN(mode="inference", model_dir=MODEL_DIR, config=MaskRCNNConfig()) # Load pre-trained model model.load_weights(COCO_MODEL_PATH, by_name=True) # Location of parking spaces parked_car_boxes = None # Load the video file we want to run detection on video_capture = cv2.VideoCapture(VIDEO_SOURCE) # How many frames of video we've seen in a row with a parking space open free_space_frames = 0 # Have we sent an SMS alert yet? sms_sent = False # Loop over each frame of video while video_capture.isOpened(): success, frame = video_capture.read() if not success: break # Convert the image from BGR color (which <mark data-type="technologies" data-id="9c38872b-5720-41cc-84ed-debc155db10c">OpenCV</mark> uses) to RGB color rgb_image = frame[:, :, ::-1] # Run the image through the Mask R-CNN model to get results. results = model.detect([rgb_image], verbose=0) # Mask R-CNN assumes we are running detection on multiple images. # We only passed in one image to detect, so only grab the first result. r = results[0] # The r variable will now have the results of detection: # - r['rois'] are the bounding box of each detected object # - r['class_ids'] are the class id (type) of each detected object # - r['scores'] are the confidence scores for each detection # - r['masks'] are the object masks for each detected object (which gives you the object outline) if parked_car_boxes is None: # This is the first frame of video - assume all the cars detected are in parking spaces. # Save the location of each car as a parking space box and go to the next frame of video. parked_car_boxes = get_car_boxes(r['rois'], r['class_ids']) else: # We already know where the parking spaces are. Check if any are currently unoccupied. # Get where cars are currently located in the frame car_boxes = get_car_boxes(r['rois'], r['class_ids']) # See how much those cars overlap with the known parking spaces overlaps = mrcnn.utils.compute_overlaps(parked_car_boxes, car_boxes) # Assume no spaces are free until we find one that is free free_space = False # Loop through each known parking space box for parking_area, overlap_areas in zip(parked_car_boxes, overlaps): # For this parking space, find the max amount it was covered by any # car that was detected in our image (doesn't really matter which car) max_IoU_overlap = np.max(overlap_areas) # Get the top-left and bottom-right coordinates of the parking area y1, x1, y2, x2 = parking_area # Check if the parking space is occupied by seeing if any car overlaps # it by more than 0.15 using IoU if max_IoU_overlap < 0.15: # Parking space not occupied! Draw a green box around it cv2.rectangle(frame, (x1, y1), (x2, y2), (0, 255, 0), 3) # Flag that we have seen at least one open space free_space = True else: # Parking space is still occupied - draw a red box around it cv2.rectangle(frame, (x1, y1), (x2, y2), (0, 0, 255), 1) # Write the IoU measurement inside the box font = cv2.FONT_HERSHEY_DUPLEX cv2.putText(frame, f"{max_IoU_overlap:0.2}", (x1 + 6, y2 - 6), font, 0.3, (255, 255, 255)) # If at least one space was free, start counting frames # This is so we don't alert based on one frame of a spot being open. # This helps prevent the script triggered on one bad detection. if free_space: free_space_frames += 1 else: # If no spots are free, reset the count free_space_frames = 0 # If a space has been free for several frames, we are pretty sure it is really free! if free_space_frames > 10: # Write SPACE AVAILABLE!! at the top of the screen font = cv2.FONT_HERSHEY_DUPLEX cv2.putText(frame, f"SPACE AVAILABLE!", (10, 150), font, 3.0, (0, 255, 0), 2, cv2.FILLED) # If we haven't sent an SMS yet, sent it! if not sms_sent: print("SENDING SMS!!!") message = client.messages.create( body="Parking space open - go go go!", from_=twilio_phone_number, to=destination_phone_number ) sms_sent = True # Show the frame of video on the screen cv2.imshow('Video', frame) # Hit 'q' to quit if cv2.waitKey(1) & 0xFF == ord('q'): break # Clean up everything when finished video_capture.release() cv2.destroyAllWindows()
要运行这份代码,你首先需要安装 python 3.6+,MatterportMask R-CNN以及OpenCV。
我特意保留了比较简单的代码。例如,它只是假设第一帧视频中出现的任何车辆都是停放的汽车。试用一下,看看你是否能够提升它的可用性。
不必担心为了在其它场景中使用而修改代码。仅仅改变模型寻找的目标 ID,你就能够将这份代码完全转换成另一个东西。例如,假设你在滑雪场工作。经过一些调整,你就可以将这份脚本转换为一个系统,它可以自动检测滑雪板从斜坡上跳越,并创建出很酷的滑雪板跳越路线。或者如果你在野生动物保护区工作,你可以将这份代码转换成一个统计野生斑马数量的系统。唯一的限制只是你的想象力。祝你玩得开心!
参考原文:https://medium.com/@ageitgey/snagging-parking-spaces-with-mask-r-cnn-and-python-955f2231c400
Recommend
About Joyk
Aggregate valuable and interesting links.
Joyk means Joy of geeK