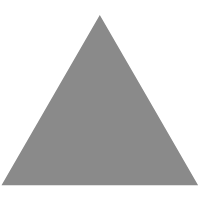
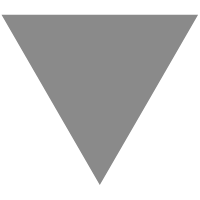
GitHub - yilirin/BDCI_Car_2018: BDCI 2018 汽车行业用户观点主题及情感识别 决赛一...
source link: https://github.com/yilirin/BDCI_Car_2018
Go to the source link to view the article. You can view the picture content, updated content and better typesetting reading experience. If the link is broken, please click the button below to view the snapshot at that time.
ReadMe.md
汽车行业用户观点主题及情感识别 (Just a test 团队决赛一等奖方案)
注意:
- 目前开源的代码按照下面的说明应该是可以跑通的,但是因为整个框架比较复杂所以可能有文档没有说清楚的地方,遇到问题可以给我们提issue,或者email
- 我们的实验表明,其实只用BERT就能达到一个非常好的结果,和全部模型比差距比较小,所以如果不是太关心完美复现,可以只跑bert的代码,这样会省去很多的时间。
- 我们的代码目前还没有进行优化,所以里面会有很多不完美的地方,比如我们很多网络没有用batch,请大家见谅。以后有时间的话,我们会考虑更新,优化一下代码结构。
- 如果有其他的问题也可以给我们反馈。
代码运行环境:
* 基于Anaconda的python3 (最好是python3.5)
* pytorch 0.4.*
* skmulti-learn
* tqdm
* hanlp (分词需要,不过我们已经提供了预处理过的文件,可以不装)
方案概述:
- 我们采用pipeline的方式,将这个任务拆为两个子任务,先预测主题,根据主题预测情感极性(ABSA),这两个任务我们都使用深度学习的方式来解决
- 主题分类是一个多标签分类问题,我们使用BCE来解决多标签问题,我们使用不同的模型不同的词向量(2*4)训练了8个模型,再加上微调的中文BERT,一种九个模型,我们使用stacking的方式在第二层利用LR极性模型融合,得到预测概率,并使用threshold得到最终预测的标签。
- 基于角度的情感分类是一个有两个输入的多分类问题,我们使用了三种比较新的网络设计和四种词向量再加上微调的BERT一共13个模型,同样我们也用LR来做stacking。
pretrained models:
- 我们将预训练好的模型放在了下面的链接中,可以直接拿来测试,免去长时间的训练。
- 链接: BaiduYun 提取码: 47e7
- 其中:
- backup_polarity.zip:保存了用来预测情感极性的三个模型四种embedding五折共60个checkpoint。请将其backup_polarity里面的各个文件夹放在polarity_level_aspect/目录下。
- backup_aspect.zip保存了用来预测主题的两个模型四种embedding五折共40个checkpoint。请将backup里面的各个文件夹放在attribute_level/目录下。
- backup_bert.zip保存了分别用来预测主题和情感极性五折共是十个checkpoint。请将其里面的两个个文件夹放在berrt/glue_data/目录下。并且要将polarity_ensemble_online_submit重命名为polarity_ensemble_online
- backup_chinese_bert.zip 保存了我们将谷歌开源的中文BERT转为pytorch版本的预训练模型,可以用来做fine tune。请将chinese_L-12_H-768_A-12文件夹放在bert/目录下。
- backup_embedding.zip 保存了我们使用的embedding, 主要是一个由elmo得到的句子表示。请将backup_embedding下的词向量放在embedding/目录下。
代码框架:
- dataset/: 存放原始的数据集,以及预处理脚本
- data/: 存放预处理过的数据集,最终主题的预测以及情感的预测也会存储在这里。
- train.txt: 预处理之后的训练集
- test.txt: 预处理之后的测试集
- vocabulary.pkl:词表
- test_predict_aspect_ensemble.txt: 预测主题的结果文件
- test_predict_polarity_ensemble.txt: 预测情感极性的结果文件
- submit2.py:生成最终的提交结果
- submit2.csv: 最终的提交结果。
- embedding/: 存储我们处理过的词向量文件以及elmo
- embedding_all_merge_300.txt, 来自于Chinese-Word-Vectors的mixed-large的Word feature.
- embedding_all_fasttext2_300.txt, 来自于fasttext
- embedding_all_tencent_200.txt, 来自于Tencent AI Lab Embedding Corpus
- embeddings_elmo_ly-1.hdf5, 使用中文elmo得到的句子表示,elmo来自于ELMoForManyLangs 。因为太大,所以我们没有放在代码这里,你可以在百度云链接中的backup_embedding.zip中找到它
- attribute_level/:运行主题分类的模块, 里面有:
- attribute_level.py: 主要运行文件,主要接受以下命令行参数:
- --mode: 运行模式,
- 0: 代表leave out 训练,
- 1: 代表五折交叉训练, 用于后面的stacking
- 2: stacking, 利用五折交叉训练好的模型进行预测并stacking。
- --model: 训练使用的模型:
- CNN
- AttA3: 一种使用label attention的RNN模型
- --w2v: 指定使用的词向量:
- merge: embedding_all_merge_300.txt
- fasttext2: fasttext词向量
- tencent: 腾讯词向量
- --use_elmo: 是否使用elmo
- 0 : 不使用elmo
- 2 : 只使用elmo,读取embedding/中的elmo的hdf5文件,最终表示和词向量无关。
- --EPOCHS: 训练轮数
- --saved: stacking测试时是从头测试,还是直接读取存储好的预测结果
- 0 : 读取checkpoint对dev集和测试集进行预测
- 1 :直接读取存储好的dev集和测试集预测结果
- --check_dir:训练时指定checkpoint的存储位置
- --test_dir: 指定测试时读取checkpoint或者预测结果的文件夹位置, 因为做stacking同时读取多个模型,所以可以用指定多个文件夹,用‘#’做分隔
- --mode: 运行模式,
- networks2.py: 我们实现的模型网络代码文件
- 保存各个模型的checkpoint的文件夹:命名格式为cp_ModelName_w2vName,
- w2vName中,0代表merge 词向量, 2 代表使用了elmo(没有用词向量),ft2 代表fasttext词向量, tc代表腾讯词向量。
- attribute_level.py: 主要运行文件,主要接受以下命令行参数:
- polarity_level_aspect: 给定主题的情感分类模块:
- ab_polarity.py :主要运行文件, 命令行参数类似于attribute_level.py
- networks.py :模型实现文件
- utils: 一些代码工具,比如封装数据集类,训练骨架,评测函数等。
One Step:
- 因为训练模型比较久而且模型比较大,所以我们提供了所有checkpoint对OOF和测试集的预测结果,只需要简单的做一下stacking就可以得到我们提交的最好结果:
cd attribute_level
python attribute.py --mode 2 --test_dir cp_CNN_0#cp_CNN_ft2#cp_CNN_2#cp_CNN_tc#cp_AttA3_0#cp_AttA3_ft2#cp_AttA3_2#cp_AttA3_tc#cp_Bert --saved 1
cd ../polarity_level_aspect
python ab_polarity.py --mode 2 --test_dir cp_HEAT_0#cp_AT_LSTM_0#cp_HEAT_ft2#cp_AT_LSTM_ft2#cp_HEAT_2#cp_AT_LSTM_2#cp_HEAT_tc#cp_AT_LSTM_tc#cp_GCAE_0#cp_GCAE_2#cp_GCAE_ft2#cp_GCAE_tc#cp_Bert --saved 1
cd ../data
python submit2.py
最后生成的submit2.csv即可用于提交。
- 当然如果想要从头复现,可以看下面的说明:
预处理模块:
- 主要就是分词,分别运行clean_data.py, 和clean_test.py文件在data文件夹中生成预处理好的train.txt和test.txt
- 不过我们已经提供了预处理好的文件,所以不需要运行。
运行主题分类模块:
-
训练阶段:(由于训练时间比较长,你可以直接跳到第三步加载我们预训练好的模型)
首先进入attribute_level文件夹:
cd attribute_level
以五折交叉训练基于fasttext词向量的CNN模型为例:只需运行:
python attribute.py --mode 1 --model CNN --use_elmo 0 --w2v fasttext2 --EPOCHS 5 --check_dir cp_CNN_ft2
这样就会在cp_CNN_ft2文件夹中生成五个checkpoint,名称为如下格式:checkpoint_Model_score_fold.pt 类似地所有模型和embedding执行命令如下:
# CNN + merge: python attribute.py --mode 1 --model CNN --use_elmo 0 --w2v merge --EPOCHS 5 --check_dir cp_CNN_0 # CNN + fasttext: python attribute.py --mode 1 --model CNN --use_elmo 0 --w2v fasttext2 --EPOCHS 5 --check_dir cp_CNN_ft2 # CNN + tencent: python attribute.py --mode 1 --model CNN --use_elmo 0 --w2v tencent --EPOCHS 5 --check_dir cp_CNN_tc # CNN + elmo: python attribute.py --mode 1 --model CNN --use_elmo 2 --EPOCHS 5 --check_dir cp_CNN_2
训练AttA3模型如下:
# AttA3 + merge: python attribute.py --mode 1 --model AttA3 --use_elmo 0 --w2v merge --EPOCHS 5 --check_dir cp_AttA3_0 # AttA3 + fasttext: python attribute.py --mode 1 --model AttA3 --use_elmo 0 --w2v fasttext2 --EPOCHS 5 --check_dir cp_AttA3_ft2 # AttA3 + tencent: python attribute.py --mode 1 --model AttA3 --use_elmo 0 --w2v tencent --EPOCHS 5 --check_dir cp_AttA3_tc # AttA3 + elmo: python attribute.py --mode 1 --model AttA3 --use_elmo 2 --EPOCHS 5 --check_dir cp_AttA3_2
至此我们在各对应文件夹中共得到了40个checkpoint。
-
微调Bert阶段:
- 我们修改了一个开源的pytorch版本的BERT, 并在本数据集上fine tune了谷歌放出来的中文BERT
- 首先我们我们将数据集按五折处理成tsv格式,放在bert/glue_data下,(我们已经帮你处理过了)
- 下载预训练的BERT模型,在backup_chinese_bert.zip中,我们已经帮你将模型转为了pytorch模型,只需将chinese_L-12_H-768_A-12文件夹放在bert/目录下
- 设置环境变量:
export GLUE_DIR=glue_data export BERT_BASE_DIR=chinese_L-12_H-768_A-12
- 在bert/文件夹下运行下面的命令进行fine-tune (5cv): (需要一块8GB显存的GPU)
python run_classifier_ensemble.py --task_name Aspect --do_train --do_eval --do_lower_case --data_dir $GLUE_DIR/aspect_ensemble_online --vocab_file $BERT_BASE_DIR/vocab.txt --bert_config_file $BERT_BASE_DIR/bert_config.json --init_checkpoint $BERT_BASE_DIR/pytorch_model.bin --max_seq_length 128 --train_batch_size 24 --learning_rate 2e-5 --num_train_epochs 5 --output_dir $GLUE_DIR/aspect_ensemble_online --seed 42
- fine-tune之后会在各自的fold的文件夹下得到对应的预测结果oof_test.npy
-
使用预训练好的模型:
- 以上两步的所有checkpoint我们都放在了百度云链接里,下载解压之后,放入对应的文件目录下即可,这样可以免去长时间的训练。
- 注意文件夹的对应关系
- 很遗憾我们没有保存Aspect的BERT checkpoint, 我们只保存了它的预测结果,因为在训练过程中,我们已经预测过了。
- load 模型时, 我们都是在GPU上读取和保存的,我们没有在CPU上进行过测试,所以如果load有问题可以自行修改load处语法,或者联系我们。
- 预测和stacking阶段:
- 不管是从头训练还是直接下载,我们现在已经有了训练好的模型,我们可以进行预测。
- 我们首先用BERT模型进行预测,事实上我们在每个fold训练时已经将预测结果保存为npy,我们现在只需要将五折结合起来。
在 bert\glue_data\文件夹下运行下面命令:
python generate_npy.py aspect_ensemble_online
这样我们在aspect_ensemble_online路径下得到一个npy文件夹,将它拷贝到aspect level 下的cp_Bert目录即可。
cp -r ../bert/glue_data/aspect_ensemble_online/npy cp_Bert/
然后我们用之前的40个checkpoint对测试集进行预测:
python attribute.py --mode 2 --saved 0 --use_elmo 2 --test_dir cp_CNN_0#cp_CNN_ft2#cp_CNN_2#cp_CNN_tc#cp_AttA3_0#cp_AttA3_ft2#cp_AttA3_2#cp_AttA3_tc
这样会在对应checkpoint的目录下生成一个npy文件夹,里面存放了oof的预测,oof的label,以及test的预测结果。
最后我们将这9个模型的npy进行stacking:
python attribute.py --mode 2 --saved 1 --test_dir cp_CNN_0#cp_CNN_ft2#cp_CNN_2#cp_CNN_tc#cp_AttA3_0#cp_AttA3_ft2#cp_AttA3_2#cp_AttA3_tc#cp_Bert
最终预测的主题结果, 存放在data/test_predict_aspect_ensemble.txt中。
运行情感分类模块:
- 训练阶段:(由于训练时间比较长,你可以直接跳到第三步加载我们预训练好的模型)
- 和主题分类类似:
最终我们得到3种网络4种embedding 在5折下的60个checkpoint保存在对应的文件夹中。# AT_LSTM + merge: python ab_polarity.py --mode 1 --model AT_LSTM --use_elmo 0 --w2v merge --EPOCHS 5 --check_dir cp_AT_LSTM_0 # AT_LSTM + fasttext: python ab_polarity.py --mode 1 --model AT_LSTM --use_elmo 0 --w2v fasttext2 --EPOCHS 5 --check_dir cp_AT_LSTM_ft2 # AT_LSTM + tencent: python ab_polarity.py --mode 1 --model AT_LSTM --use_elmo 0 --w2v tencent --EPOCHS 5 --check_dir cp_AT_LSTM_tc # AT_LSTM + elmo: python ab_polarity.py --mode 1 --model AT_LSTM --use_elmo 2 --EPOCHS 5 --check_dir cp_AT_LSTM_2 # HEAT + merge: python ab_polarity.py --mode 1 --model HEAT --use_elmo 0 --w2v merge --EPOCHS 5 --check_dir cp_HEAT_0 # HEAT + fasttext: python ab_polarity.py --mode 1 --model HEAT --use_elmo 0 --w2v fasttext2 --EPOCHS 5 --check_dir cp_HEAT_ft2 # HEAT + tencent: python ab_polarity.py --mode 1 --model HEAT --use_elmo 0 --w2v tencent --EPOCHS 5 --check_dir cp_HEAT_tc # HEAT + elmo: python ab_polarity.py --mode 1 --model HEAT --use_elmo 2 --EPOCHS 5 --check_dir cp_HEAT_2 # GCAE + merge: python ab_polarity.py --mode 1 --model GCAE --use_elmo 0 --w2v merge --EPOCHS 5 --check_dir cp_GCAE_0 # GCAE + fasttext: python ab_polarity.py --mode 1 --model GCAE --use_elmo 0 --w2v fasttext2 --EPOCHS 5 --check_dir cp_GCAE_ft2 # GCAE + tencent: python ab_polarity.py --mode 1 --model GCAE --use_elmo 0 --w2v tencent --EPOCHS 5 --check_dir cp_GCAE_tc # GCAE + elmo: python ab_polarity.py --mode 1 --model GCAE --use_elmo 2 --EPOCHS 5 --check_dir cp_GCAE_2
- 微调Bert阶段:
- 和主题分类类似,但是需要一个aspect预测的结果作为输入。运行data文件夹下的build_test_for_predict.py脚本后, 将生成的test.tsv放在bert/glue_data/polarity_ensemble_online/下即可。
- 设置环境变量:
export GLUE_DIR=glue_data export BERT_BASE_DIR=chinese_L-12_H-768_A-12
- 在bert/文件夹下运行下面的命令进行fine-tune (5cv): (需要一块8GB显存的GPU)
python run_classifier_ensemble_polarity.py --task_name Polarity --do_train --do_eval --do_lower_case --data_dir $GLUE_DIR/polarity_ensemble_online --vocab_file $BERT_BASE_DIR/vocab.txt --bert_config_file $BERT_BASE_DIR/bert_config.json --init_checkpoint $BERT_BASE_DIR/pytorch_model.bin --max_seq_length 128 --train_batch_size 24 --learning_rate 2e-5 --num_train_epochs 5 --output_dir $GLUE_DIR/polarity_ensemble_online --seed 42
- fine-tune之后会在各自的fold的文件夹下得到对应的checkpoint,最好的模型是model_best.pt
- 使用预训练好的模型:
- 如果不做上面两步长时间的训练可以直接用我们训练好的模型
- 从百度云中下载之后解压到backup_polarity.zip得到60个checkpoint,将backup_bert.zip中的polarity_ensemble_polarity放在bert/glue_data/下。
- 注意文件夹的对应关系
- 预测和stacking阶段:
- 我们首先用BERT模型进行预测,每个fold下有一个model_best.pt的checkpoint,我们通过下面的命令加载它们并进行预测(记得像微调时一样设置环境变量):
python run_classifier_ensemble_polarity.py --task_name Polarity --do_test --do_predict --do_lower_case --data_dir $GLUE_DIR/polarity_ensemble_online --vocab_file $BERT_BASE_DIR/vocab.txt --bert_config_file $BERT_BASE_DIR/bert_config.json --init_checkpoint $BERT_BASE_DIR/pytorch_model.bin --max_seq_length 128 --train_batch_size 24 --learning_rate 2e-5 --num_train_epochs 10 --output_dir polarity_output_ensemble_online/ --eval_batch_size 32
- 然后我们将五折的预测结果结合起来:
python generate_npy_for_polarity.py polarity_ensemble_online
我们将生成的npy文件夹拷贝到polarity_level_aspect路径下:
cp -r ../bert/glue_data/polarity_ensemble_online/npy cp_Bert/
类似地我们先生成60个checkpoint的oof的npy:
python ab_polarity.py --mode 2 --use_elmo 2 --saved 0 --test_dir cp_HEAT_0#cp_AT_LSTM_0#cp_HEAT_ft2#cp_AT_LSTM_ft2#cp_HEAT_2#cp_AT_LSTM_2#cp_HEAT_tc#cp_AT_LSTM_tc#cp_GCAE_0#cp_GCAE_2#cp_GCAE_ft2#cp_GCAE_tc
然后将这13个模型进行最终的stacking融合:
python ab_polarity.py --mode 2 --saved 1 --test_dir cp_HEAT_0#cp_AT_LSTM_0#cp_HEAT_ft2#cp_AT_LSTM_ft2#cp_HEAT_2#cp_AT_LSTM_2#cp_HEAT_tc#cp_AT_LSTM_tc#cp_GCAE_0#cp_GCAE_2#cp_GCAE_ft2#cp_GCAE_tc#cp_Bert
这样我们最终在data文件夹下生成了test_predict_polarity_ensemble.txt文件,里面即为预测结果。
提交:
- 在data目录下, 运行提交脚本:
cd data
python submit2.py
生成的submi2.csv 即为我们的提交文件。
Contact:
sqfzf69(At)163.com
Recommend
About Joyk
Aggregate valuable and interesting links.
Joyk means Joy of geeK