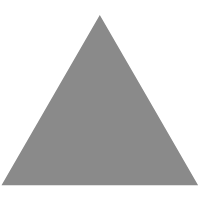
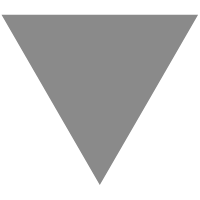
Document worth reading: “A Tutorial on Bayesian Optimization”
source link: https://www.tuicool.com/articles/hit/BRj6B3j
Go to the source link to view the article. You can view the picture content, updated content and better typesetting reading experience. If the link is broken, please click the button below to view the snapshot at that time.
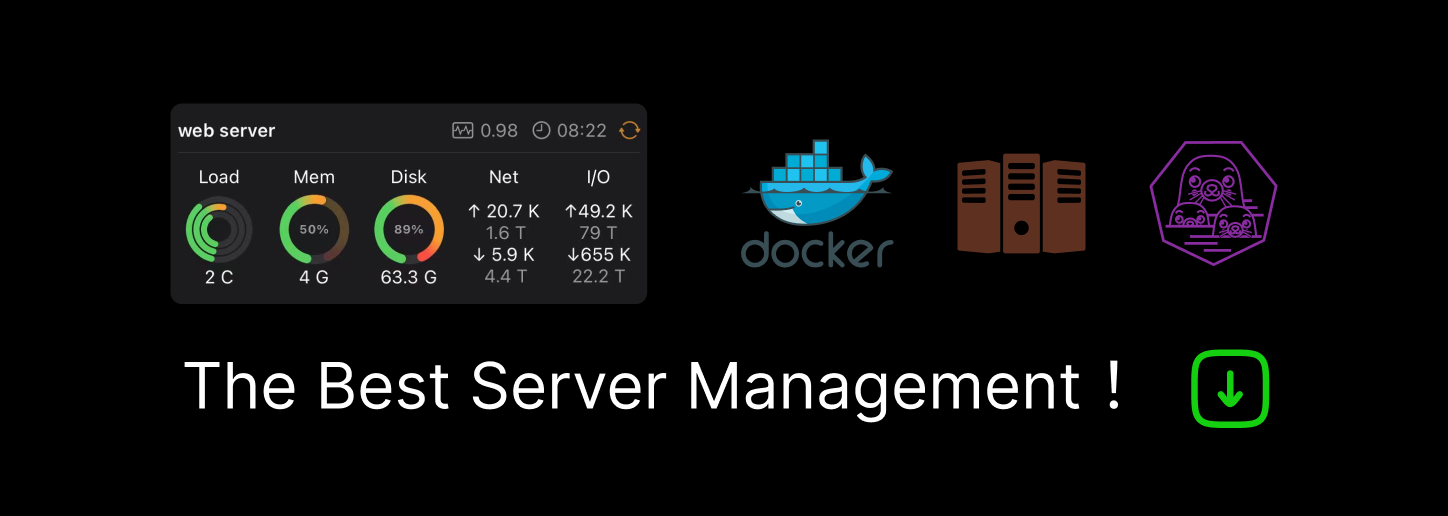
Bayesian optimization is an approach to optimizing objective functions that take a long time (minutes or hours) to evaluate. It is best-suited for optimization over continuous domains of less than 20 dimensions, and tolerates stochastic noise in function evaluations. It builds a surrogate for the objective and quantifies the uncertainty in that surrogate using a Bayesian machine learning technique, Gaussian process regression, and then uses an acquisition function defined from this surrogate to decide where to sample. In this tutorial, we describe how Bayesian optimization works, including Gaussian process regression and three common acquisition functions: expected improvement, entropy search, and knowledge gradient. We then discuss more advanced techniques, including running multiple function evaluations in parallel, multi-fidelity and multi-information source optimization, expensive-to-evaluate constraints, random environmental conditions, multi-task Bayesian optimization, and the inclusion of derivative information. We conclude with a discussion of Bayesian optimization software and future research directions in the field. Within our tutorial material we provide a generalization of expected improvement to noisy evaluations, beyond the noise-free setting where it is more commonly applied. This generalization is justified by a formal decision-theoretic argument, standing in contrast to previous ad hoc modifications. A Tutorial on Bayesian Optimization
Advertisements
Recommend
-
60
The full article is available at research.fb.com. Facebook relies on a large suite of backend systems to serve billions of people each day. Many of these systems have a large number of internal parameters. Fo...
-
46
The cost of communication is a substantial factor affecting the scalability of many distributed applications. Every message sent can incur a cost in storage, computation, energy and bandwidth. Consequently, reducing...
-
36
README.md ...
-
40
With the art and practice of government policy-making, public work, and citizen participation, many governments adopt information and communication technologies (ICT) as a vehicle to facilitate their relationship with...
-
14
Injecting Machine Learning And Bayesian Optimization Into HPC
-
7
贝叶斯优化 (Bayesian Optimization) 范叶亮 / 2020-06-06 分类: 机器学习,
-
7
Using Bayesian optimization for balancing metrics in recommendation systems Co-authors: Yunbo Ouyang,
-
10
An example of Bayesian hyperparameter optimization parallelized on cloud VMs using Kubeflow Published: Nov 22, 2022 Screenshot of the execution of the Kubeflow pipelin...
-
3
☂️ BoCoEL Bayesian Optimization as a Coverage Tool for Evaluating Large Language Models
-
5
Grow your net worth with smart tax optimizationSort by: The idea for Carry came when I sold my last startup Teachable and realized I knew nothing about how the US tax code works. It's super complicated (150,000...
About Joyk
Aggregate valuable and interesting links.
Joyk means Joy of geeK