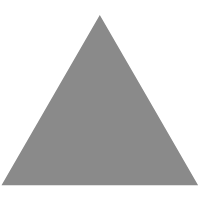
51
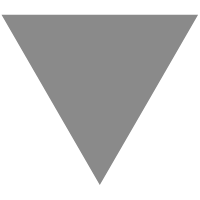
functools 源码阅读
source link: https://jiajunhuang.com/articles/2017_01_08-functools_source_code.md.html?amp%3Butm_medium=referral
Go to the source link to view the article. You can view the picture content, updated content and better typesetting reading experience. If the link is broken, please click the button below to view the snapshot at that time.
functools主要包括这几个东西: wraps
, partial
, lru_cache
, 还有一些内置
帮助函数例如 c3_mro
。我们主要看上面三个:
wraps
wraps实现很简单,就是把a函数的某些属性拷贝到b函数。
partial
partial的实现,看下面代码就可以了:
class Partial: def __new__(*args, **kwargs): cls, func, *args = args self = super(Partial, cls).__new__(cls) self.func = func self.args = args self.kwargs = kwargs return self def __call__(*args, **kwargs): self, *new_args = args new_kwargs = self.kwargs.copy() new_kwargs.update(kwargs) return self.func(*self.args, *new_args, **new_kwargs) mypartial = Partial # noqa if __name__ == "__main__": def foo(first, second, hello="world"): print("first, second, hello = %s, %s, %s" % (first, second, hello)) bar = mypartial(foo, second=2, hello="hello") bar(1)
主要原理就是先用一个类来保存原函数的一些状态,然后重写 __call__
方法,把
对应的已经partial的参数一起塞进去。
lru_cache
(Least-recently-used cache)
这个比较有意思, def lru_cache(maxsize=128, typed=False):
,如果maxsize为0,
那就直接返回函数结果,如果为None,那就直接用一个字典存储,如果为具体数值,
那么还会有一个环状双向链表来保存顺序。
lru_cache
的实现主要包括三个部分:
- `class _HashedSeq(list)` - `def _make_key(...省略一把参数...)` - `def lru_cache(maxsize=128, typed=False)`
其中第一个类用来保存hash值,第二个用来根据函数的参数生成key,第三个基于前两个
实现了 lru_cache
。
下面是我的一个实现:
class Link: __slots__ = 'prev', 'next', 'key', 'value' def _make_key(args, kwargs, kwargs_mark=(object(), )): key = args if kwargs: sorted_kwargs = sorted(kwargs.items()) key += kwargs_mark for kwarg in sorted_kwargs: key += kwarg return hash(key) def lru_cache(maxsize=128): def decorator(user_func): return _lru_cache_wrapper(user_func, maxsize) return decorator def _lru_cache_wrapper(user_func, maxsize): cache = {} root = Link() root.prev, root.next, root.key, root.value = root, root, None, None def decorator(*args, **kwargs): nonlocal cache, root key = _make_key(args, kwargs) if key in cache: value = cache[key] else: value = user_func(*args, **kwargs) # 更新环状双向链表 last = root.prev link = Link() link.prev = last link.next = root link.key, link.value = key, value root.prev = last.next = link if key not in cache: # 更新缓存信息和环状双向链表,因为缓存有大小限制 cache[key] = value last = root.prev root = root.next root.prev = last last.next = root return value return decorator if __name__ == "__main__": import time # 普通递归版fib函数 start = time.time() def fib(x): if x == 0 or x == 1: return x else: return fib(x - 1) + fib(x - 2) result = fib(35) end = time.time() print("result: %s, use time: %.6f" % (result, end - start)) # 为了方便看,还是不用函数形式的decorator,而是直接把fib函数抄一遍 start = time.time() @lru_cache() def fib_cache(x): if x == 0 or x == 1: return x else: return fib_cache(x - 1) + fib_cache(x - 2) result = fib_cache(35) end = time.time() print("result: %s, use time: %.6f" % (result, end - start))
运行结果:
root@arch tests: python myfunctools.py result: 9227465, use time: 4.576693 result: 9227465, use time: 0.000146
Recommend
About Joyk
Aggregate valuable and interesting links.
Joyk means Joy of geeK