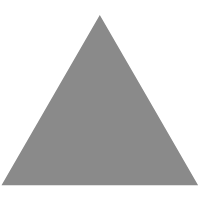
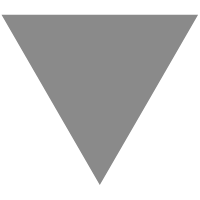
GitHub - hanxiao/bert-as-service: Mapping a variable-length sentence to a fixed-...
source link: https://github.com/hanxiao/bert-as-service
Go to the source link to view the article. You can view the picture content, updated content and better typesetting reading experience. If the link is broken, please click the button below to view the snapshot at that time.
README.md
bert-as-service
Using BERT model as a sentence encoding service, i.e. mapping a variable-length sentence to a fixed-length vector.
Author: Han Xiao https://hanxiao.github.io
BERT code of this repo is forked from the original BERT repo with necessary modification, especially in extract_features.py.
What is it?
BERT: Developed by Google, BERT is a method of pre-training language representations. It leverages an enormous amount of plain text data publicly available on the web and is trained in an unsupervised manner. Pre-training a BERT model is a fairly expensive yet one-time procedure for each language. Fortunately, Google released several pre-trained models where you can download from here.
Sentence Encoding/Embedding: sentence encoding is a upstream task required in many NLP applications, e.g. sentiment analysis, text classification. The goal is to represent a variable length sentence into a fixed length vector, each element of which should "encode" some semantics of the original sentence.
Finally, this repo: This repo uses BERT as the sentence encoder and hosts it as a service via ZeroMQ, allowing you to map sentences into fixed-length representations in just two lines of code.
Usage
1. Download the Pre-trained BERT Model
Download it from here, then uncompress the zip file into some folder, say /tmp/english_L-12_H-768_A-12/
2. Start a BERT service
python app.py -num_worker=4 -model_dir /tmp/english_L-12_H-768_A-12/
This will start a service with four workers, meaning that it can handel up to four concurrent requests. (These workers are behind a simple load balancer.)
3. Use Client to Encode Sentences
NOTE: please make sure your project includes
client.py
, as we need to importBertClient
class from this file. This is the only file that you will need as a client.
Now you can use pretrained BERT to encode sentences in your Python code simply as follows:
from service.client import BertClient ec = BertClient() ec.encode(['abc', 'defg', 'uwxyz'])
This will return a python object with type List[List[float]]
, each element of the outer List
is the fixed representation of a sentence.
Using BERT Service Remotely
One can also start the service on one (GPU) machine and call it from another (CPU) machine as follows
# on another CPU machine from service.client import BertClient ec = BertClient(ip='xx.xx.xx.xx', port=5555) # ip address of the GPU machine ec.encode(['abc', 'defg', 'uwxyz'])
NOTE: please make sure your project includes
client.py
, as we need to importBertClient
class from this file. Again, this is the only file that you will need as a client.
QA on Technical Details
Q: Where do you get the fixed representation? Did you do pooling or something?
A: I take the second-to-last hidden layer of all of the tokens in the sentence and do average pooling. See the function I added to the modeling.py
Q: Why not use the hidden state of the first token, i.e. the [CLS]
?
A: Because a pre-trained model is not fine-tuned on any downstream tasks yet. In this case, the hidden state of [CLS]
is not a good sentence representation. If later you fine-tune the model, you may use get_pooled_output()
to get the fixed length representation as well.
Q: Why not the last hidden layer? Why second-to-last?
A: The last layer is too closed to the target functions (i.e. masked language model and next sentence prediction) during pre-training, therefore may be biased to those targets.
Q: Could I use other pooling techniques?
A: For sure. Just follows get_sentence_encoding()
I added to the modeling.py. Note that, if you introduce new tf.variables
to the graph, then you need to train those variables before using the model. You may also want to check some pooling techniques I mentioned in my blog post.
Q: How many requests can a service handle concurrently?
A: The maximum number of concurrent requests is determined by num_worker
in app.py
. If you a sending more than num_worker
requests concurrently, the new requests will be temporally stored in a queue until a free worker becomes available.
Q: So one request means one sentence?
A: No. One request means a list of sentences sent from a client. Think the size of a request as the batch size. A request may contain 256, 512 or 1024 sentences. The optimal size of a request is often determined empirically. One large request can certainly improve the GPU utilization, yet it also increases the overhead of transmission. You may run python client_example.py
for a simple benchmark.
Q: How about the speed? Is it fast enough for production?
A: It highly depends on the max_seq_len
and the size of a request. On a single Tesla M40 24GB with max_seq_len=25
, you should get about 390/s using a 12-layer BERT. In general, I'd suggest smaller max_seq_len
(25) and larger request size (512/1024).
Q: Did you benchmark the efficiency?
A: Yes. I tested the service speed in terms of number of processed sentences per second under different max_seq_len
(a server side parameter) and batch size (a client side parameter). Here are the results on 6-core Tesla M40 24GB:
To reproduce the results, please refer to benchmark.py.
Q: What is backend based on?
A: ZeroMQ.
Recommend
About Joyk
Aggregate valuable and interesting links.
Joyk means Joy of geeK