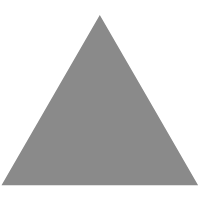
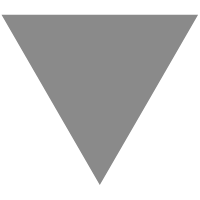
GitHub - NVIDIA/waveglow: A Flow-based Generative Network for Speech Synthesis
source link: https://github.com/NVIDIA/waveglow
Go to the source link to view the article. You can view the picture content, updated content and better typesetting reading experience. If the link is broken, please click the button below to view the snapshot at that time.
README.md
WaveGlow: a Flow-based Generative Network for Speech Synthesis
Ryan Prenger, Rafael Valle, and Bryan Catanzaro
In our recent paper, we propose WaveGlow: a flow-based network capable of generating high quality speech from mel-spectrograms. WaveGlow combines insights from Glow and WaveNet in order to provide fast, efficient and high-quality audio synthesis, without the need for auto-regression. WaveGlow is implemented using only a single network, trained using only a single cost function: maximizing the likelihood of the training data, which makes the training procedure simple and stable.
Our PyTorch implementation produces audio samples at a rate of more than 500 kHz on an NVIDIA V100 GPU and Mean Opinion Scores show that it delivers audio quality as good as the best publicly available WaveNet implementation.
Visit our website for audio samples.
Setup
- Clone our repo and initialize submodule
git clone https://github.com/NVIDIA/waveglow.git
git submodule init
git submodule update
- Install requirements (same as those from submodule)
pip3 install -r tacotron2/requirements.txt
Generate audio with our pre-existing model
- Download our published model
- Download mel-spectrograms
- Generate audio
python3 inference.py -f <(ls mel_spectrograms/*.pt) -w waveglow_old.pt -o . --is_fp16 -s 0.6
Train your own model
- Download LJ Speech Data. In this example it's in
data/
- Make a list of the file names to use for training/testing
ls data/*.wav | tail -n+10 > train_files.txt
ls data/*.wav | head -n10 > test_files.txt
- Train your WaveGlow networks
mkdir checkpoints
python train.py -c config.json
For multi-GPU training replace train.py
with distributed.py
. Only tested with single node and NCCL.
5. Make test set mel-spectrogramspython mel2samp.py -f test_files.txt -o . -c config.json
6. Do inference with your network
ls *.pt > mel_files.txt
python3 inference.py -f mel_files.txt -w checkpoints/waveglow_10000 -o . --is_fp16 -s 0.6
Recommend
About Joyk
Aggregate valuable and interesting links.
Joyk means Joy of geeK