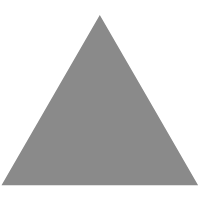
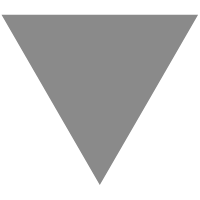
Understanding Performance Fluctuations in Quantum Processors
source link: https://chinagdg.org/2018/09/understanding-performance-fluctuations-in-quantum-processors/
Go to the source link to view the article. You can view the picture content, updated content and better typesetting reading experience. If the link is broken, please click the button below to view the snapshot at that time.
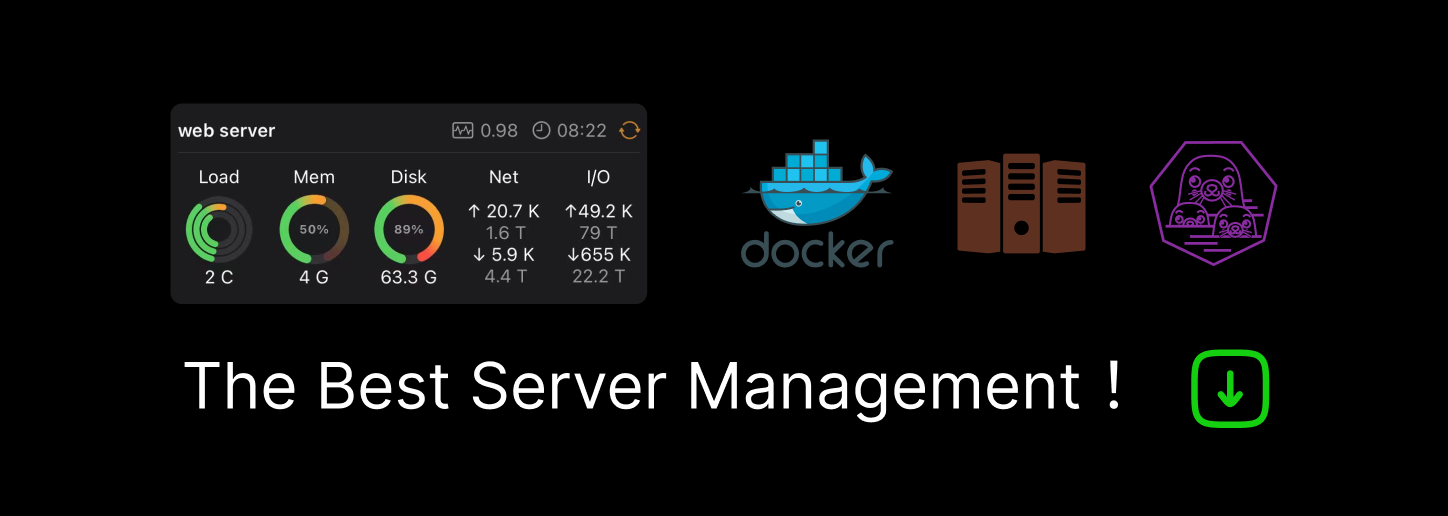

Understanding Performance Fluctuations in Quantum Processors
Source: Understanding Performance Fluctuations in Quantum Processors from Google Research
Posted by Paul V. Klimov, Research Scientist, Google AI Quantum Team
One area of research the Google AI Quantum team pursues is building quantum processors from superconducting electrical circuits, which are attractive candidates for implementing quantum bits (qubits). While superconducting circuits have demonstrated state-of-the-art performance and extensibility to modest processor sizes comprising tens of qubits, an outstanding challenge is stabilizing their performance, which can fluctuate unpredictably. Although performance fluctuations have been observed in numerous superconducting qubit architectures, their origin isn’t well understood, impeding progress in stabilizing processor performance.
In “Fluctuations of Energy-Relaxation Times in Superconducting Qubits” published in this week’s Physical Review Letters, we use qubits as probes of their environment to show that performance fluctuations are dominated by material defects. This was done by investigating qubits’ energy relaxation times (T1) — a popular performance metric that gives the length of time that it takes for a qubit to undergo energy-relaxation from its excited to ground state — as a function of operating frequency and time.
In measuring T1, we found that some qubit operating frequencies are significantly worse than others, forming energy-relaxation hot-spots (see figure below). Our research suggests that these hot spots are due to material defects, which are themselves quantum systems that can extract energy from qubits when their frequencies overlap (i.e. are “resonant”). Surprisingly, we found that the energy-relaxation hot spots are not static, but “move” on timescales ranging from minutes to hours. From these observations, we concluded that the dynamics of defects’ frequencies into and out of resonance with qubits drives the most significant performance fluctuations.

These defects — which are typically referred to as two-level-systems (TLS) — are commonly believed to exist at the material interfaces of superconducting circuits. However, even after decades of research, their microscopic origin still puzzles researchers. In addition to clarifying the origin of qubit performance fluctuations, our data shed light on the physics governing defect dynamics, which is an important piece of this puzzle. Interestingly, from thermodynamics arguments we would not expect the defects that we see to exhibit any dynamics at all. Their energies are about one order of magnitude higher than the thermal energy available in our quantum processor, and so they should be “frozen out.” The fact that they are not frozen out suggests their dynamics may be driven by interactions with other defects that have much lower energies and can thus be thermally activated.
The fact that qubits can be used to investigate individual material defects – which are believed to have atomic dimensions, millions of times smaller than our qubits – demonstrates that they are powerful metrological tools. While it’s clear that defect research could help address outstanding problems in materials physics, it’s perhaps surprising that it has direct implications on improving the performance of today’s quantum processors. In fact, defect metrology already informs our processor design and fabrication, and even the mathematical algorithms that we use to avoid defects during quantum processor runtime. We hope this research motivates further work into understanding material defects in superconducting circuits.
Recommend
-
26
On the Path to Cryogenic Control of Quantum Processors 2019-02-22adm...
-
3
Random Numbers in JavaScript: From Math.random() to the Quantum Fluctuations of a VacuumWhy JS Math.random() is not perfectly random and how you can get truly random numbers.
-
9
Blockchain is more than memes, wild price fluctuations and my old pal Elon’s tweetsMedia Sourcery, Inc. of Austin, Texas announces our Cold Chain solution, built for Trust and TransparencyThough many bl...
-
11
Keep it cool — Startup hopes the world is ready to buy quantum processors Quantum processors are mostly used via cloud services; QuantWare will ship you one.
-
5
PPC Google Ads issue resulted in spend fluctuations for tROAS and Maximize Conversion Value bid strategies on December 2 "The iss...
-
4
-
8
Introduction Most SAP Systems are configured in a three-tier configuration with multiple application servers. For a given peak number of users/transactions and a resulting peak usage of system resources CPU/Memory/Work Processes a frequen...
-
8
Compensating for grid fluctuations with bivalent furnaces by Fraun...
-
5
Crypto Market Faces Sharp Decline: Fraud Charges and Market Cap Fluctuations Send Investors on Edge
-
3
Market Overview The global activated carbon market is a cornerstone of environmental and industrial solutions, characterized by its remarkable versatility and vital role across multiple sectors. Activated carbon, a porous and highly a...
About Joyk
Aggregate valuable and interesting links.
Joyk means Joy of geeK