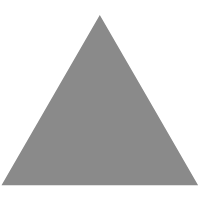
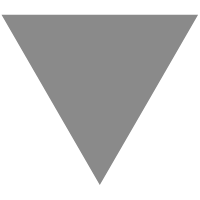
The Dark Silicon Problem and What It Means for CPU Designers (2013)
source link: https://www.tuicool.com/articles/hit/6NvMjuV
Go to the source link to view the article. You can view the picture content, updated content and better typesetting reading experience. If the link is broken, please click the button below to view the snapshot at that time.
If you've been paying attention to ARM press releases over the last year, you'll have seen a lot of references to something ominously called the dark silicon problem . Given the amount of marketing terminology surrounding it, you might be forgiven for assuming that it's a marketing buzzword of some kind. But dark silicon is a real problem. In this article, we'll take a look at what the dark silicon problem means for CPU designers.
What's the Problem?
Once upon a time, transistors on an integrated circuit were a scarce resource. The 8086 had fewer than 30,000 transistors, which today seems a tiny number to implement a general-purpose processor, yet that was about three times the number in competing processors. CPU designers had to ensure that each one of these transistors was doing something useful, because adding another thousand transistors would significantly increase the cost of the part.
Today, CPUs and GPUs have transistor counts measured in the billions. Adding a few thousand transistors to make a particular task faster doesn't noticeably increase the cost, but a new problem has arisen: power usage, which is closely related to heat dissipation. For every watt of power the CPU consumes, it must dissipate a watt of heat.
Unfortunately, while the number of transistors that can be put on a chip cheaply has increased, the power consumption per transistor hasn't dropped at a corresponding rate. The amount of power per transistor has dropped, but more slowly than the size of the transistor has shrunk. Eventually, you hit some real physical limits; this problem isn't just theoretical. The first mainstream microprocessor to encounter this serious problem was the Pentium 4, a decade ago.
The heat generation per unit area of an integrated circuit passed the surface of a 100-watt light bulb in the mid 1990s, and now is somewhere between the inside of a nuclear reactor and the surface of a star. When something is generating that much heat, keeping it in the temperature range where silicon transistors work is quite difficult.
So you can put billions of transistors on a chip, but if you want to make a CPU rather than a barbecue, you can't use all of those transistors at the same time. This fact has made a big difference in how CPUs are designed, and the problem will only increase in the future. A processor that can use only 5% of its transistors at any given time will have very different characteristics from one that can use 50%.
Dedicated Coprocessors
One of the first changes has been the rise of dedicated coprocessors and specialized instructions. Floating-point units (FPUs) were the first example, but they were added for performance, not power efficiency. You can emulate floating-point arithmetic by using integer instructions—but taking 10–100 times as long. Adding a dedicated floating-point unit cost some transistors, so it wasn't until the 486 line that FPUs started becoming a standard component in Intel CPUs. With the 386, the choice was either to have an FPU or to use those transistors to make the rest of the pipeline faster. The correct decision was obvious—the option that made all code faster, not just the subset that was mainly floating-point arithmetic.
Now, however, the situation is reversed. The FPU is effectively free. The transistors it uses can't be used to make the rest of the pipeline faster, for two reasons. First, most of the tricks that can be used to improve throughput are already being used, and the rest quickly get into diminishing returns. Second is the dark silicon issue. Adding transistors to the main pipeline that you're going to use all the time means that you have to settle for a much lower clock rate if you want to keep the same power envelope. In contrast, the floating-point unit consumes very little power when you're not executing floating-point instructions; when you are, the FPU executes them a lot faster (and more power-efficiently) than if you were doing the same thing with the integer pipeline.
Floating-point coprocessors were the first example, and they were extended to become SIMD coprocessors. Modern CPUs go even further. For example, the ARMv8 architecture has a small number of instructions dedicated to performing AES encryption. The latest iteration of SSE has several instructions so obscure that few algorithms will ever use them.
These instructions are increasingly worth adding. Dedicated silicon is always more efficient than using general-purpose instructions to implement any given algorithm, so using them always saves power (and gives you a performance win). When they're not used, their cost is constantly dropping.
Instruction Decoders and Friends
So far, I've talked about transistors as if they're equivalent to arithmetic/logic units—the bits of the chip that actually do the calculations. On a modern chip, however, a lot of peripheral circuitry is required for the chip to be a general-purpose processor. The most obvious is the instruction decoder, which is near the start of the pipeline, and is responsible (in the loosest possible terms) for passing the inputs to each of the execution units.
Because if its place in the pipeline, the instruction decoder is among the small set of things that must draw power all the time. Every instruction must be decoded and dispatched. The complexity of the instruction decoder was one of the most significant driving forces behind the RISC movement. It's something of a tradeoff, because having a complex instruction decoder means that you can also have denser instruction encoding, which means in turn that you need less instruction cache for the same number of instructions. This is traditionally a win for x86, which has a variable-length instruction set, with instructions somewhere between 1 and 15 bytes. RISC architectures typically had a fixed 32-bit (4-byte) instruction set and a larger average instruction size.
ARM also has a variable-length instruction set called Thumb-2 . In its much simpler encoding, every instruction is either 16 or 32 bits and is usually denser than x86 code. The decoder for ARM instructions is also a lot simpler. Modern Xeons are an exception to the rule that the decoder must be powered constantly, because in tight loops they cache the decoded micro-ops and don't use the main decoder. Unfortunately, the micro-ops are of similar decoding complexity to ARM or Thumb-2 instructions, so this is only a power saving relative to the cost of x86 decoding.
This is also a tradeoff when it comes to dedicated instructions for more obscure uses. The transistors used to implement them may no longer be a scarce resource, but short instruction encodings are. On x86, they can just use the longer encodings, but ARM offers a relatively small number of possible opcodes.
Heterogeneous Multicore
The typical solution to this issue is to have dedicated asynchronous coprocessors. The GPU is one example, and an ARM system on chip (SoC) typically has a small collection of others for image, video, and sound processing. They don't take up space in the instruction encodings because they appear as devices, and therefore are used by writing values to specific addresses. The downside of this approach is its relatively high overhead; it's only worth using for relatively long-running calculations, such as interpolation on an image or decoding a video frame. Fortunately, these applications tend to be the most interesting kinds of problems to offload to dedicated coprocessors.
If the coprocessors are on the same die as the main processor, they can be behind the same memory management unit as well and have a unified view of memory, making them cheap to invoke from userspace. In this case, the line between synchronous and asynchronous is slightly blurred. If you start a job running on a coprocessor but put the main CPU into a low-power state until it's finished, you have a synchronous operation, with some parts of the program being offloaded to the more efficient coprocessor. This is more or less how FPUs have worked since around the time of the DEC Alpha: an asynchronous pipeline, with very cheap operations to synchronize it with the rest of the chip.
You can think of ARM's big.LITTLE designs as being a very simple case of heterogeneous multicore. They typically have a small set of Cortex-A15 and Cortex-A7 cores that have identical instruction sets, but very different internal implementations. The A15 is a multiple-issue, superscalar, out-of-order chip. The A7 is in-order, and dual-issue in the best case. Therefore, although they can run the same programs, the A15 is a lot faster, whereas the A7 requires a lot less power.
Note
For more on big.LITTLE, see my article Scheduling Challenges with ARM's big.LITTLE Architecture .
The Future
Over the next decade, barring unexpected shifts in technology, CPU and SoC designers will have a lot of transistors to dedicate to infrequent use. These transistors will offer lots of potential, ranging from accelerating specific algorithms to providing Turing-complete processors optimized for different usage patterns. Modern GPUs and CPUs currently fall into this latter category. Both are capable of running any algorithm, but the CPU is heavily optimized for instruction-level parallelism and locality of reference, whereas the GPU is optimized for code with few branches and streaming accesses to memory.
As with any change in the hardware, this increase in capability will bring new challenges for compilers and operating systems. How do you schedule a process with different threads that not only run in parallel, but on entirely different core types? Do you always expose the parts on different cores as different threads, or do you allow a sequential task to move from one type of core to another? If the latter, how do you handle scheduling for a single "thread" that needs to move from a CPU to a DSP and back again over the course of a function? How do you expose this in a programming language?
Summary
The dark silicon problem, as with the increased focus on power consumption over the last decade, changes the set of constraints that CPU designers must take into account. Bringing a new processor to market takes 3–5 years, and the effect won't be noticeable for another couple of process generations, but it's something to think about for anyone who designs ICs. By the time they're entering production, it's likely to be a dominant factor limiting their performance.
Recommend
About Joyk
Aggregate valuable and interesting links.
Joyk means Joy of geeK