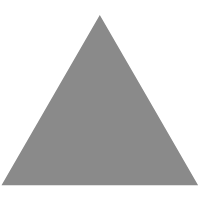
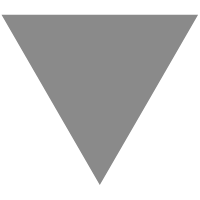
使用 Flamegraphs 对 Go 程序进行性能分析
source link: https://studygolang.com/articles/13540?amp%3Butm_medium=referral
Go to the source link to view the article. You can view the picture content, updated content and better typesetting reading experience. If the link is broken, please click the button below to view the snapshot at that time.
2018 年 2 月 28 日
应用的性能问题生来就是无法预料的 —— 而且他们总是在最坏的时间露头。让情况更糟的是,很多性能分析工具都是冷冰冰的,复杂难懂的,用起来彻头彻尾令人困惑的 —— 来自于 `valgrind` 和 `gdp` 这样最受推崇的性能分析工具的用户体验。
`Flamegraphs` 是由 linux 性能分析大师 Brendan Gegg 创造的一个工具,在一般的 linux 性能追踪 dump 之上生成一个 SVG 可视化层 ,给定位和解决性能问题这个复杂的过程带来了一些“温暖”。在这篇文章中,我们会一步一步地用 `flamegraphs` 对一个简单的 golang 写的 web 应用进行性能分析。
## 开始之前的一些题外话
只有当你知道自己的程序有性能问题时,才对它进行性能分析和优化。过早的性能优化不仅会在当下浪费你的时间,而且如果以后你不得不进行重构时,这些精心调整过的脆弱的代码,会拖慢你。
## 示例程序
我们将用一个小的 HTTP 服务器来演示,这个服务器通过 `GET /ping` 暴露了一个 healthcheck 的 API. 为了可视化,我们同时包含了一个小的 [statsd](https://www.datadoghq.com/blog/statsd/) 客户端用来记录服务器处理的每个请求的延迟。为了保持简单,我们的代码仅仅用到 go 的标准库,不过即使你习惯使用 `gorilla/mux` 或者别的流行的库,这些代码对你来说也不会太陌生。
```go import ( "fmt" "log" "net/http" "strings" "net" "time" )
// SimpleClient is a thin statsd client. type SimpleClient struct { c net.PacketConn ra *net.UDPAddr }
// NewSimpleClient instantiates a new SimpleClient instance which binds // to the provided UDP address. func NewSimpleClient(addr string) (*SimpleClient, error){ c, err := net.ListenPacket("udp", ":0") if err != nil { return nil, err }
ra, err := net.ResolveUDPAddr("udp", addr) if err != nil { c.Close() return nil, err }
return &SimpleClient{ c: c, ra: ra, }, nil }
// Timing sends a statsd timing call. func (sc *SimpleClient) Timing(s string, d time.Duration, sampleRate float64, tags map[string]string) error { return sc.send(fmtStatStr( fmt.Sprintf("%s:%d|ms",s, d/ time.Millisecond), tags), ) }
func (sc *SimpleClient) send(s string) error { _, err := sc.c.(*net.UDPConn).WriteToUDP([]byte(s), sc.ra) if err != nil { return err }
return nil }
func fmtStatStr(stat string, tags map[string]string) string { parts := []string{} for k, v := range tags { if v != "" { parts = append(parts, fmt.Sprintf("%s:%s", k, v)) } }
return fmt.Sprintf("%s|%s", stat, strings.Join(parts, ",")) }
func main() { stats, err := NewSimpleClient("localhost:6060") if err != nil { log.Fatal("could not start stas client: ", err) }
// add handlers to default mux http.HandleFunc("/ping", pingHandler(stats))
s := &http.Server{ Addr: ":8080", }
log.Fatal(s.ListenAndServe()) }
func pingHandler(s *SimpleClient) http.HandlerFunc{ return func(w http.ResponseWriter, r *http.Request) { st := time.Now() defer func() { _ = s.Timing("http.ping", time.Since(st), 1.0, nil) }()
w.WriteHeader(200) }} ```
## 安装性能分析工具
go 的标准库内置了诊断性能问题的工具,有一套丰富而完整的工具可以嵌入 go 简单高效的运行时。如果你的应用使用的是默认的 `http.DefaultServeMux` ,那么集成 `pprof` 无需额外的代码,只需在你的 `import` 头部加入以下语句。
```go import ( _ "net/http/pprof" ) ```
你可以通过启动服务器并在任意浏览器访问 `debug/pprof` 来验证你的配置是否都正确。对我们的示例应用来说 —— pprof 接口暴露在 `localhost:8080/debug/pprof` 。
## 生成 Flamegraph
`flamegraph` 工具的工作是接收你系统的已有的一个堆栈跟踪文件,进行解析,生成一个 SVG 可视化。为了得到其中一个神秘的堆栈跟踪文件,我们可以使用随 go 一起安装的 [pprof](https://github.com/google/pprof) 工具。为了将东西整合在一起,免受安装和配置更多软件之苦,我们将使用 [uber/go-torch](https://github.com/uber/go-torch) 这个出色的库 —— 它为整个过程提供了非常方便的集装箱式的工作流程。
Flamegraph 可以生成自各种各样的配置文件,每一个都针对不同的性能指标。你可以使用同样的工具包和方法论来寻找 CPU 的性能瓶颈、内存泄漏,甚至是死锁的进程。
下面来为我们的示例应用生成一个 `flamegraph`,执行以下命令来抓取 `uber/go-torch` 容器并将其指向你的应用。
``` # run for 30 seconds docker run uber/go-torch -u http://<host ip>:8080/debug/pprof -p -t=30 > torch.svg ```
### 生成请求负载
如果你的应用服务器运行在本地,或者是在 staging 环境,复现最初让系统出现性能问题的场景可能很困难。作为模拟生产环境的工作负载的一种途径,我们将使用一个叫做 [vegeta](https://github.com/tsenart/vegeta) 的负载测试小工具来模拟出与线上每台服务器处理的请求相当的吞吐量。
`vegeta` 有一个极其强大的可配置API,支持不同类型的负载测试及基准测试场景。在我们的简单服务器的案例里,我们可以通过以下单行指令来产生足够的流量,来让事情变得有趣。
``` # send 250rps for 60 seconds echo "GET http://localhost:8080/ping" | vegeta attack -rate 250 -duration=60s | vegeta report ```
运行这个脚本的同时用 `go-torch` 工具进行监听,就可以产生一个名为 `torch.svg` 的文件。用 Chrome 打开这个文件,就会有一个与你的程序对应的漂亮的 flamegraph 跟你打招呼!
``` open -a `Google Chrome` torch.svg ```

## 读懂 Flamegraph
flamegraph 中的每一个水平区段代表一个栈帧,决定它的宽度的是采样过程中你的程序被观察到在对这个帧进行求值的相对(%)时间。这些区段在垂直方向上根据在调用栈中的位置被组织成一个个的 "flame",也就是说在图的 y轴方向上位于上方的函数是被位于下方的函数调用的 —— 自然地,在上方的函数比下方的函数占用了更小片的 CPU 时间。如果你想深入视图中的某一部分,很简单,只需要点击某一帧,这样位于这帧下方的帧都会消失,而且界面会自己调整尺寸。

*注:栈帧的颜色是无意义的,完全随机——色调和色度的区分是为了让图更易读。*
通过直接查看或是点击几个帧来缩小范围——有没有性能问题及问题是什么应该会即刻变得显而易见。记住 [80/20 规律](https://en.wikipedia.org/wiki/Pareto_principle) ,你的大部分性能问题都会出现在做了比它该做的多得多的少部分代码上——别把你的时间花在flamegraph图表中那些薄小的穗上。

举例来说,在我们的程序中,我们可以深入到那些较大片的区段,看到我们花了10%(!) 的时间在将结果通过网络 socket 刷到我们的统计服务器!幸运的是,修复这个很简单——通过在我们的代码中添加一个小的buffer,我们可以解决这个问题,然后产生一个新的,更纤细的图。
### Code Change
```go func (sc *SimpleClient) send(s string) error { sc.buffer = append(sc.buffer, s) if len(sc.buffer) > bufferCapacity {
b := strings.Join(sc.buffer, ",") _, err := sc.c.(*net.UDPConn).WriteToUDP([]byte(b), sc.ra) if err != nil { return err }
sc.buffer = nil }
return nil } ```
### New flamegraph

就是它了!Flamegraph是个窥测你的应用性能的简单且强大的工具。试着为你的应用产生一个 flamegraph——你的发现可能会给你带来惊喜:)
## 扩展阅读
想学习更多?这里有些不错的链接:
- [Flamegraphs - Brendan Gegg](http://www.brendangregg.com/flamegraphs.html) - [The Flame Graph - ACMQ](https://queue.acm.org/detail.cfm?id=2927301) - [The Mature Optimization Handbook](https://www.facebook.com/notes/facebook-engineering/the-mature-optimization-handbook/10151784131623920/) - [Profiling and Optimizing go Applications](https://www.youtube.com/watch?v=N3PWzBeLX2M)
via: http://brendanjryan.com/golang/profiling/2018/02/28/profiling-go-applications.html
作者: Brendanj Ryan 译者: krystollia 校对: rxcai
Recommend
About Joyk
Aggregate valuable and interesting links.
Joyk means Joy of geeK