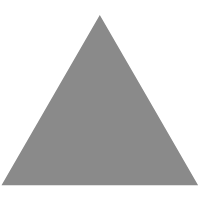
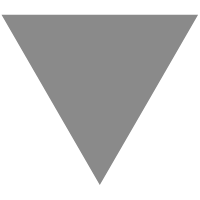
Introducing ONNX support
source link: https://www.tuicool.com/articles/hit/a6FzYnR
Go to the source link to view the article. You can view the picture content, updated content and better typesetting reading experience. If the link is broken, please click the button below to view the snapshot at that time.
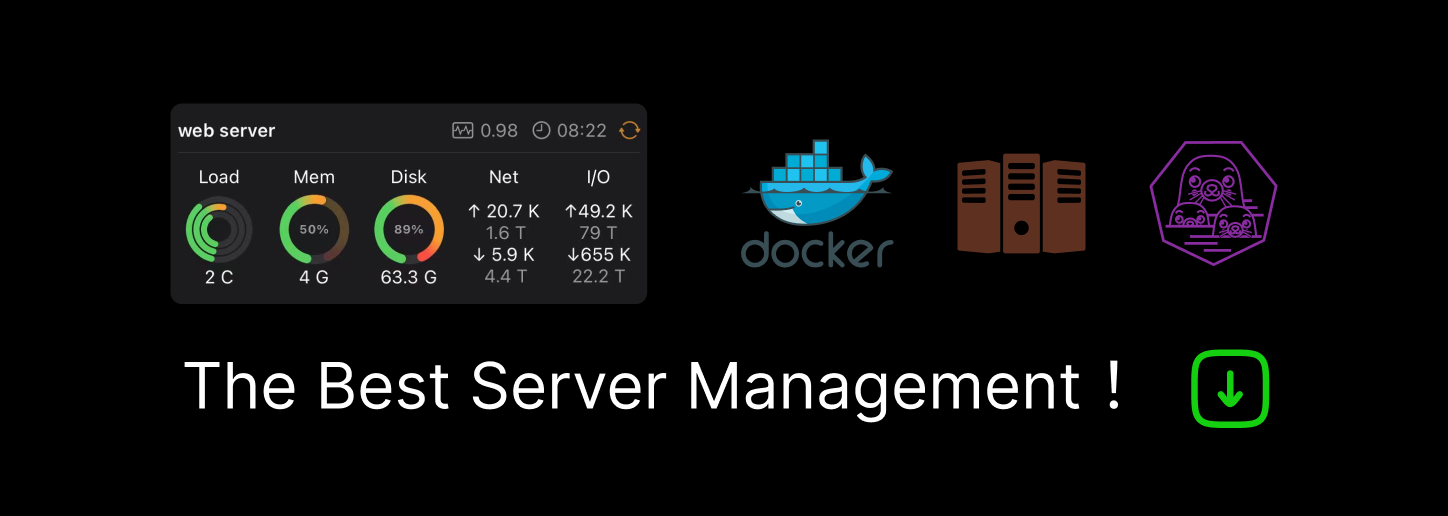
ONNX (Open Neural Network eXchange) is an open format for the sharing of neural network and other machine learned models between various machine learning and deep learning frameworks. As the open big data serving engine, Vespa aims to make it simple to evaluate machine learned models at serving time at scale. By adding ONNX support in Vespa in addition to our existing TensorFlow support, we’ve made it possible to evaluate models from all the commonly used ML frameworks with low latency over large amounts of data.
With the rise of deep learning in the last few years, we’ve naturally enough seen an increase of deep learning frameworks as well: TensorFlow, PyTorch/Caffe2, MxNet etc. One reason for these different frameworks to exist is that they have been developed and optimized around some characteristic, such as fast training on distributed systems or GPUs, or efficient evaluation on mobile devices. Previously, complex projects with non-trivial data pipelines have been unable to pick the best framework for any given subtask due to lacking interoperability between these frameworks. ONNX is a solution to this problem.
ONNX is an open format for AI models, and represents an effort to push open standards in AI forward. The goal is to help increase the speed of innovation in the AI community by enabling interoperability between different frameworks and thus streamlining the process of getting models from research to production.
There is one commonality between the frameworks mentioned above that enables an open format such as ONNX, and that is that they all make use of dataflow graphs in one way or another. While there are differences between each framework, they all provide APIs enabling developers to construct computational graphs and runtimes to process these graphs. Even though these graphs are conceptually similar, each framework has been a siloed stack of API, graph and runtime. The goal of ONNX is to empower developers to select the framework that works best for their project, by providing an extensible computational graph model that works as a common intermediate representation at any stage of development or deployment.
Vespa is an open source project which fits well within such an ecosystem, and we aim to make the process of deploying and serving models to production that have been trained on any framework as smooth as possible. Vespa is optimized toward serving and evaluating over potentially very large datasets while still responding in real time. In contrast to other ML model serving options, Vespa can more efficiently evaluate models over many data points . As such, Vespa is an excellent choice when combining model evaluation with serving of various types of content.
Our ONNX support is quite similar to ourTensorFlow support. Importing ONNX models is as simple as adding the model to the Vespa application package (under “models/”) and referencing the model using the new ONNX ranking feature:
expression: sum(onnx("my_model.onnx"))
The above expression runs the model and sums it to a single scalar value to use in ranking. You will have to provide the inputs to the graph. Vespa expects you to provide a macro with the same name as the input tensor. In the macro you can specify where the input should come from, be it a document field, constant or a parameter sent along with the query. More information can be had in the documentation about ONNX import .
Internally, Vespa converts the ONNX operations to Vespa’s tensor API. We do the same for TensorFlow import. So the cost of evaluating ONNX and TensorFlow models are the same. We have put a lot of effort in optimizing the evaluation of tensors, and evaluating neural network models can be quite efficient .
ONNX support is also quite new to Vespa, so we do not support all current ONNX operations . Part of the reason we don’t support all operations yet is that some are potentially too expensive to evaluate per document, such as convolutional neural networks and recurrent networks (LSTMs etc). ONNX also contains an extension, ONNX-ML , which contains additional operations for non-neural network cases. Support for this extension will come later at some point. We are continually working to add functionality, so please reach out to us if there is something you would like to have added.
Going forward we are continually working on improving performance as well as supporting more of the ONNX (and ONNX-ML) standard. You can read more about ranking with ONNX models in the Vespa documentation . We are excited to announce ONNX support. Let us know what you are building with it!
Recommend
-
1340
README.md TensorRT backend for ONNX Parses ONNX models for execution with TensorRT.
-
42
How to Convert Your Keras Model to ONNX Intuition I love Keras for its simplicity. With about 10 minutes, I can build a deep learning model with its sequential or functional API with elegant code. However, Keras always load...
-
16
Operationalizing ML models with ONNX, C# .... and Pokemon!On .NET Live - Operationalizing ML models with ONNX, C# .... and Pokemon! - YouTube
-
251
README.md This repository represents Ultralytics open-source research into future object detection methods, and incorporates lessons learned and best practices evolved...
-
11
作者丨立交桥跳水冠军 来源丨https://zhuanlan.zhihu.com/p/272767300 编辑丨GiantPandaCV 之前几个月参与了Open MMlab的...
-
16
0x0. 背景 最近看了一些ONNX的资料,一个最大的感受就是这些资料太凌乱了。大多数都是在介绍ONNX模型转换中碰到的坑点以及解决办法。很少有文章可以系统的介绍ONNX的背景,分析ONNX格式,ONNX简化方法等。所以,综合了相当多资料之后我准备写一篇...
-
199
Continuing from Introducing OnnxSharp and ‘dotnet onnx’, in this post I will look at using OnnxSharp to set dynamic batch s...
-
8
Intro When building a Machine Learning model, you’re probably using some of the popular frameworks like TensorFlow/PyTorch/sklearn. You run experiments, play with different models and architectures, fine-tune hyperparame...
-
13
作业帮基于 WeNet + ONNX 的端到端语音识别方案 2021 年 7 月 19 日
-
7
How to Run Machine-Learning Models in the Browser using ONNXAugust 25th 2021 new story3...
About Joyk
Aggregate valuable and interesting links.
Joyk means Joy of geeK