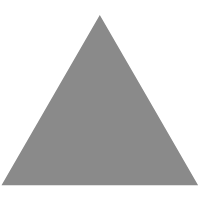
1
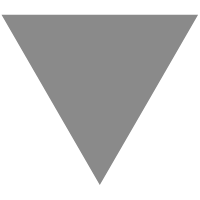
[2310.19786] From External to Swap Regret 2.0: An Efficient Reduction and Oblivi...
source link: https://arxiv.org/abs/2310.19786
Go to the source link to view the article. You can view the picture content, updated content and better typesetting reading experience. If the link is broken, please click the button below to view the snapshot at that time.
Computer Science > Machine Learning
[Submitted on 30 Oct 2023 (v1), last revised 6 Dec 2023 (this version, v3)]
From External to Swap Regret 2.0: An Efficient Reduction and Oblivious Adversary for Large Action Spaces
We provide a novel reduction from swap-regret minimization to external-regret minimization, which improves upon the classical reductions of Blum-Mansour [BM07] and Stolz-Lugosi [SL05] in that it does not require finiteness of the space of actions. We show that, whenever there exists a no-external-regret algorithm for some hypothesis class, there must also exist a no-swap-regret algorithm for that same class. For the problem of learning with expert advice, our result implies that it is possible to guarantee that the swap regret is bounded by {\epsilon} after \log(N)^{O(1/\epsilon)} rounds and with O(N) per iteration complexity, where N is the number of experts, while the classical reductions of Blum-Mansour and Stolz-Lugosi require O(N/\epsilon^2) rounds and at least \Omega(N^2) per iteration complexity. Our result comes with an associated lower bound, which -- in contrast to that in [BM07] -- holds for oblivious and \ell_1-constrained adversaries and learners that can employ distributions over experts, showing that the number of rounds must be \tilde\Omega(N/\epsilon^2) or exponential in 1/\epsilon.
Our reduction implies that, if no-regret learning is possible in some game, then this game must have approximate correlated equilibria, of arbitrarily good approximation. This strengthens the folklore implication of no-regret learning that approximate coarse correlated equilibria exist. Importantly, it provides a sufficient condition for the existence of correlated equilibrium which vastly extends the requirement that the action set is finite, thus answering a question left open by [DG22; Ass+23]. Moreover, it answers several outstanding questions about equilibrium computation and learning in games.
Subjects: | Machine Learning (cs.LG); Artificial Intelligence (cs.AI); Computer Science and Game Theory (cs.GT) |
Cite as: | arXiv:2310.19786 [cs.LG] |
(or arXiv:2310.19786v3 [cs.LG] for this version) | |
https://doi.org/10.48550/arXiv.2310.19786 |
Submission history
From: Noah Golowich [view email][v1] Mon, 30 Oct 2023 17:50:29 UTC (328 KB)
[v2] Tue, 31 Oct 2023 17:57:22 UTC (332 KB)
[v3] Wed, 6 Dec 2023 07:34:24 UTC (345 KB)
Recommend
About Joyk
Aggregate valuable and interesting links.
Joyk means Joy of geeK