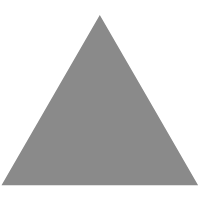
40
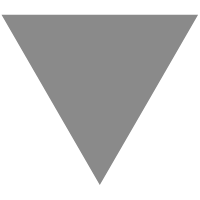
GitHub - taki0112/Tensorflow-Cookbook: Simple Tensorflow Cookbook for easy-to-us...
source link: https://github.com/taki0112/Tensorflow-Cookbook
Go to the source link to view the article. You can view the picture content, updated content and better typesetting reading experience. If the link is broken, please click the button below to view the snapshot at that time.
README.md
Web page
Contributions
In now, this repo contains general architectures and functions that are useful for the GAN.
I will continue to add useful things to other areas.
Also, your pull requests and issues are always welcome.
And write what you want to implement on the issue. I'll implement it.
How to use
Import
ops.py
- operations
- from ops import *
utils.py
- image processing
- from utils import *
Network template
def network(x, is_training=True, reuse=False, scope="network"): with tf.variable_scope(scope, reuse=reuse): x = conv(...) ... return logit
Insert data to network using DatasetAPI
Image_Data_Class = ImageData(img_size, img_ch, augment_flag) trainA = trainA.map(Image_Data_Class.image_processing, num_parallel_calls=16) trainA = trainA.shuffle(buffer_size=10000).prefetch(buffer_size=batch_size).batch(batch_size).repeat() trainA_iterator = trainA.make_one_shot_iterator() data_A = trainA_iterator.get_next() logit = network(data_A)
- See this for more information.
Option
padding='SAME'
- pad = ceil[ (kernel - stride) / 2 ]
pad_type
- 'zero' or 'reflect'
sn
- use spectral_normalization or not
Ra
- use relativistic gan or not
loss_func
- gan
- lsgan
- hinge
- wgan
- wgan-gp
- dragan
Caution
- If you don't want to share variable, set all scope names differently.
Weight
weight_init = tf.truncated_normal_initializer(mean=0.0, stddev=0.02) weight_regularizer = tf.contrib.layers.l2_regularizer(0.0001) weight_regularizer_fully = tf.contrib.layers.l2_regularizer(0.0001)
Initialization
Xavier
: tf.contrib.layers.xavier_initializer()He
: tf.contrib.layers.variance_scaling_initializer()Normal
: tf.random_normal_initializer(mean=0.0, stddev=0.02)Truncated_normal
: tf.truncated_normal_initializer(mean=0.0, stddev=0.02)Orthogonal
: tf.orthogonal_initializer(1.0) / # if relu = sqrt(2), the others = 1.0
Regularization
l2_decay
: tf.contrib.layers.l2_regularizer(0.0001)orthogonal_regularizer
: orthogonal_regularizer(0.0001) & orthogonal_regularizer_fully(0.0001)
Convolution
basic conv
x = conv(x, channels=64, kernel=3, stride=2, pad=1, pad_type='reflect', use_bias=True, sn=True, scope='conv')
partial conv (NVIDIA Partial Convolution)
x = partial_conv(x, channels=64, kernel=3, stride=2, use_bias=True, padding='SAME', sn=True, scope='partial_conv')
dilated conv
x = dilate_conv(x, channels=64, kernel=3, rate=2, use_bias=True, padding='SAME', sn=True, scope='dilate_conv')
Deconvolution
basic deconv
x = deconv(x, channels=64, kernel=3, stride=2, padding='SAME', use_bias=True, sn=True, scope='deconv')
Fully-connected
x = fully_conneted(x, units=64, use_bias=True, sn=True, scope='fully_connected')
Pixel shuffle
x = conv_pixel_shuffle_down(x, scale_factor=2, use_bias=True, sn=True, scope='pixel_shuffle_down') x = conv_pixel_shuffle_up(x, scale_factor=2, use_bias=True, sn=True, scope='pixel_shuffle_up')
down
===> [height, width] -> [height // scale_factor, width // scale_factor]up
===> [height, width] -> [height * scale_factor, width * scale_factor]
Block
residual block
x = resblock(x, channels=64, is_training=is_training, use_bias=True, sn=True, scope='residual_block') x = resblock_down(x, channels=64, is_training=is_training, use_bias=True, sn=True, scope='residual_block_down') x = resblock_up(x, channels=64, is_training=is_training, use_bias=True, sn=True, scope='residual_block_up')
down
===> [height, width] -> [height // 2, width // 2]up
===> [height, width] -> [height * 2, width * 2]
attention block
x = self_attention(x, channels=64, use_bias=True, sn=True, scope='self_attention') x = self_attention_with_pooling(x, channels=64, use_bias=True, sn=True, scope='self_attention_version_2') x = squeeze_excitation(x, channels=64, ratio=16, use_bias=True, sn=True, scope='squeeze_excitation') x = convolution_block_attention(x, channels=64, ratio=16, use_bias=True, sn=True, scope='convolution_block_attention')
Normalization
x = batch_norm(x, is_training=is_training, scope='batch_norm') x = instance_norm(x, scope='instance_norm') x = layer_norm(x, scope='layer_norm') x = group_norm(x, groups=32, scope='group_norm') x = pixel_norm(x) x = batch_instance_norm(x, scope='batch_instance_norm') x = condition_batch_norm(x, z, is_training=is_training, scope='condition_batch_norm'): x = adaptive_instance_norm(x, gamma, beta):
Activation
x = relu(x) x = lrelu(x, alpha=0.2) x = tanh(x) x = sigmoid(x) x = swish(x)
Pooling & Resize
x = up_sample(x, scale_factor=2) x = max_pooling(x, pool_size=2) x = avg_pooling(x, pool_size=2) x = global_max_pooling(x) x = global_avg_pooling(x) x = flatten(x) x = hw_flatten(x)
Loss
classification loss
loss, accuracy = classification_loss(logit, label)
pixel loss
loss = L1_loss(x, y) loss = L2_loss(x, y) loss = huber_loss(x, y) loss = histogram_loss(x, y)
histogram_loss
means the difference in the color distribution of the image pixel values.
gan loss
d_loss = discriminator_loss(Ra=True, loss_func='wgan-gp', real=real_logit, fake=fake_logit) g_loss = generator_loss(Ra=True, loss_func='wgan_gp', real=real_logit, fake=fake_logit)
- See this for how to use
gradient_penalty
kl-divergence (z ~ N(0, 1))
loss = kl_loss(mean, logvar)
Author
Junho Kim
Recommend
About Joyk
Aggregate valuable and interesting links.
Joyk means Joy of geeK