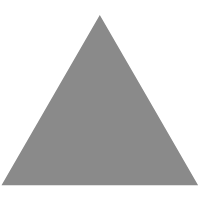
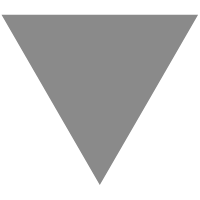
Elasticsearch Relevance Engine brings new vectors to generative AI
source link: https://venturebeat.com/ai/elasticsearch-relevance-engine-brings-new-vectors-to-generative-ai/
Go to the source link to view the article. You can view the picture content, updated content and better typesetting reading experience. If the link is broken, please click the button below to view the snapshot at that time.
Elasticsearch Relevance Engine brings new vectors to generative AI

Join top executives in San Francisco on July 11-12, to hear how leaders are integrating and optimizing AI investments for success. Learn More
Elastic is expanding the capabilities of its enterprise search technology today with the debut of the Elasticsearch Relevance Engine (ESRE), which integrates artificial intelligence (AI) and vector search to improve search relevance and support generative AI initiatives.
Elastic has been building out its enterprise Elasticsearch technology for the last decade, using the open-source Apache Lucene data indexing and search project as a foundational component. In February 2022 the company introduced a preview of its support for vector embeddings, enabling the Elasticsearch technology to act like a vector database, which is a critical part of the AI landscape.
With the new ESRE set of features, Elasticsearch now has broader vector support. Elastic is also integrating its own transformer neural network model into ESRE to help provide better semantic search results.
Going a step further, ESRE will enable enterprises to bring their own transformer models, such as OpenAI’s GPT-4, to get the benefits of generative AI in their Elasticsearch content.
Event
Transform 2023
Join us in San Francisco on July 11-12, where top executives will share how they have integrated and optimized AI investments for success and avoided common pitfalls.
“ESRE is really how we’ve finally had the opportunity to combine all of these underlying search relevance technologies into one cohesive offering,” Matt Riley, general manager, enterprise search at Elastic, told VentureBeat.
With Elasticsearch, evolution of search is ‘transformational’
For the last decade, Elasticsearch has relied on the BM25f best match algorithm to help rank and score documents to provide relevant results for search queries.
With the introduction of vector search as part of ESRE, enterprises can now search using BM25f as well as vectors. With vectors, content is assigned a numerical representation and relevance is determined by finding numbers that are close to each other using approaches such as approximate nearest neighbor (ANN).
“First and foremost at Elastic is our goal to provide the best possible ways for our customers to get relevant documents out of the vast amount of data that they store in Elasticsearch, whether that’s a vector search, or a text search using BM25f, or a hybrid combination of the two,” Riley said.
While the introduction of vector search can help improve relevance, enterprises need more to get better results from text-based queries. That’s where a new transformer model developed by Elastic, which uses a technique known as a late encoding model — a type of sparse encoding — comes into play. The model is able to understand text to help enterprises get very precise results from queries.
“Late interaction models are actually very good at doing semantic retrieval on text that the model wasn’t necessarily trained on,” Riley said.
BYOM — bring your own (transformer) model
With ESRE, Elastic is also opening up Elasticsearch to enable enterprises to bring their own AI models to gain insight from data.
As part of ESRE, Elastic is supporting an integration with OpenAI and its GPT-4 LLM that will allow organizations to use the power of generative AI with Elasticsearch content. Organizations will also be able to use open-source LLMs on Hugging Face to summarize text, do sentiment analysis and answer questions.
Riley noted that enabling an organization to connect to OpenAI and other LLMs is all about creating a bridge between the data that sits inside of Elasticsearch and LLMs, which would not have been able to train on the private data.
“I’m very excited to continue seeing the transformation of these transformer models,” Riley said. “It’s a whole new category of things that people will start building now that we have these new capabilities there.”
VentureBeat's mission is to be a digital town square for technical decision-makers to gain knowledge about transformative enterprise technology and transact. Discover our Briefings.
Recommend
About Joyk
Aggregate valuable and interesting links.
Joyk means Joy of geeK