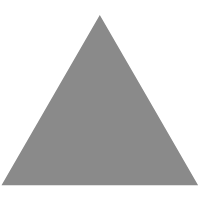
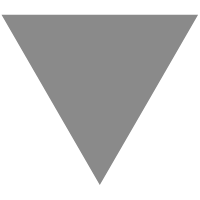
Auto-Associative Neural Networks (AANNs) for predictive maintenance
source link: https://www.neuraldesigner.com/blog/auto-associative-neural-networks
Go to the source link to view the article. You can view the picture content, updated content and better typesetting reading experience. If the link is broken, please click the button below to view the snapshot at that time.
1. Introduction
Predictive maintenance is a proactive maintenance approach that uses data analysis and machine learning techniques to identify potential equipment failures before they occur. It involves collecting and analyzing data from equipment and systems to predict when maintenance will be required and to take action to prevent equipment failure and downtime.
Predictive maintenance is essential for several reasons, including:
- Reduced downtime: Predictive maintenance can identify potential issues and address them before they become critical failures. This helps avoid unexpected downtime.
- Increased safety: Predictive maintenance can prevent accidents and injuries caused by malfunctioning equipment.
- Cost savings: Predictive maintenance can prevent minor problems from becoming major failures, which can be costly to repair.
- Better asset management: Predictive maintenance can help organizations make informed decisions about maintenance schedules, replacement timelines, and asset retirement.
- Improved equipment life: Predictive maintenance can help prevent premature equipment failure.
Predictive maintenance is a complex problem that requires analyzing vast amounts of data from different sources, including equipment sensors, maintenance records, and other relevant factors such as environmental conditions and operating parameters.
However, more than historical data is needed to capture the full range of factors that influence equipment performance, making it difficult to build accurate predictive models. Traditional statistical and machine learning techniques may need to be more effective in identifying patterns and predicting failures in complex systems.
Unlike traditional statistical models, which rely on historical data, Auto-Associative Neural Networks (AANNs) can learn underlying patterns and structures in the data and use that information to detect any significant deviations from what is considered "normal". In other words, Auto-Associative Neural Networks are a powerful tool that can be used to identify anomalous states in data.

Anomaly detection involves identifying rare or unexpected events in data that deviate significantly from what is considered normal or expected.
Recommend
About Joyk
Aggregate valuable and interesting links.
Joyk means Joy of geeK