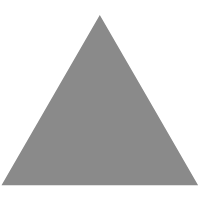
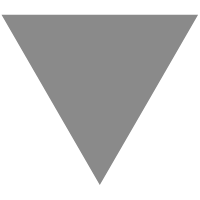
[2207.08643] A Sublinear-Time Quantum Algorithm for Approximating Partition Func...
source link: https://arxiv.org/abs/2207.08643
Go to the source link to view the article. You can view the picture content, updated content and better typesetting reading experience. If the link is broken, please click the button below to view the snapshot at that time.
Quantum Physics
[Submitted on 18 Jul 2022 (v1), last revised 7 Jan 2023 (this version, v2)]
A Sublinear-Time Quantum Algorithm for Approximating Partition Functions
We present a novel quantum algorithm for estimating Gibbs partition functions in sublinear time with respect to the logarithm of the size of the state space. This is the first speed-up of this type to be obtained over the seminal nearly-linear time algorithm of Štefankovič, Vempala and Vigoda [JACM, 2009]. Our result also preserves the quadratic speed-up in precision and spectral gap achieved in previous work by exploiting the properties of quantum Markov chains. As an application, we obtain new polynomial improvements over the best-known algorithms for computing the partition function of the Ising model, counting the number of k-colorings, matchings or independent sets of a graph, and estimating the volume of a convex body.
Our approach relies on developing new variants of the quantum phase and amplitude estimation algorithms that return nearly unbiased estimates with low variance and without destroying their initial quantum state. We extend these subroutines into a nearly unbiased quantum mean estimator that reduces the variance quadratically faster than the classical empirical mean. No such estimator was known to exist prior to our work. These properties, which are of general interest, lead to better convergence guarantees within the paradigm of simulated annealing for computing partition functions.
Comments: | 24 pages; v2: improved Theorem 3.3 and volume estimation |
Subjects: | Quantum Physics (quant-ph); Computational Complexity (cs.CC); Data Structures and Algorithms (cs.DS); Statistics Theory (math.ST); Machine Learning (stat.ML) |
Cite as: | arXiv:2207.08643 [quant-ph] |
(or arXiv:2207.08643v2 [quant-ph] for this version) | |
https://doi.org/10.48550/arXiv.2207.08643 | |
Journal reference: | Proceedings of the 34th Symposium on Discrete Algorithms (SODA), pages 1245-1264, 2023 |
Related DOI: |
Submission history
From: Yassine Hamoudi [view email][v1] Mon, 18 Jul 2022 14:41:48 UTC (38 KB)
[v2] Sat, 7 Jan 2023 09:40:50 UTC (41 KB)
Recommend
About Joyk
Aggregate valuable and interesting links.
Joyk means Joy of geeK