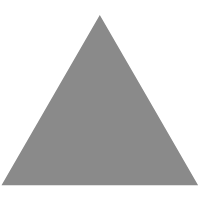
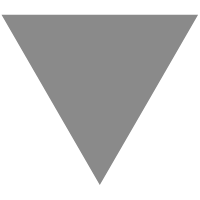
Future Benefits Of Using AI As A Site Reliability Engineer With Insight From Cha...
source link: https://build5nines.com/future-benefits-of-using-ai-as-a-site-reliability-engineer-with-insight-from-chatgpt/
Go to the source link to view the article. You can view the picture content, updated content and better typesetting reading experience. If the link is broken, please click the button below to view the snapshot at that time.
Future Benefits of using AI as a Site Reliability Engineer with insight from ChatGPT

Artificial Intelligence (AI) has the potential to greatly assist Site Reliability Engineers (SREs), or even DevOps Engineers, in a number of ways. Some potential applications of AI in SRE work might include automation of routine tasks, such as monitoring systems for issues and alerting team members when issues are detected, as well as responding to issues automatically by running scripts or performing other actions. AI can also be used for predictive maintenance to predict when issues are likely to occur, and for root cause analysis to identify the underlying cause of an issue. In addition, AI can be used to identify opportunities for improving system performance and suggest optimization strategies, allowing SREs to optimize their systems more effectively. Overall, AI has the potential to significantly improve the efficiency and effectiveness of SREs, enabling them to better manage and maintain complex systems.
For this article, I thought I would consult ChatGPT, the latest Artificial Intelligence (AI) chat bot available today, to help me with this article on ways that AI might benefit the job role of Site Reliability Engineers (SREs) in the future. So, I guess this article is co-written by myself (Chris Pietschmann) and ChatGPT. Please post a comment and let me know what you think, or just feedback in general on how you think AI will impact the role of Site Reliability Engineers or DevOps Engineers. I look forward to your feedback.
Some potential applications of Artificial Intelligence (AI) for Site Reliability Engineers includes:
- Automation of routine tasks: AI can be used to automate routine tasks such as monitoring, alerting, and responding to issues, freeing up SREs to focus on more complex tasks.
- Predictive maintenance: AI can be used to analyze patterns in system performance data and predict when issues are likely to occur, allowing SREs to proactively address potential problems before they cause disruptions.
- Root cause analysis: AI can be used to analyze large amounts of data from multiple sources to identify the root cause of issues, which can save SREs time and effort in identifying the underlying cause of problems.
- Performance optimization: AI can be used to identify opportunities for improving system performance and suggest optimization strategies, allowing SREs to optimize their systems more effectively.
Overall, AI has the potential to significantly improve the efficiency and effectiveness of SREs, enabling them to better manage and maintain complex systems.
Automation of routine tasks
AI has the potential to significantly improve the efficiency and effectiveness of SREs by automating a wide range of routine tasks. Some examples of how AI could be used to automate routine tasks include:
- Monitoring systems for issues: AI can be used to continuously monitor systems for performance issues or other problems, alerting SREs when issues are detected. This can be especially useful in systems with a large number of components or complex configurations, as it can be difficult for SREs to manually monitor every aspect of the system. AI could be used to analyze data from logs, metrics, and other sources to identify potential issues and alert SREs when action is required.
- Responding to issues automatically: In some cases, AI can be used to respond to issues automatically by running scripts or performing other actions. This can help to minimize downtime and prevent issues from escalating. For example, AI could be used to automatically restart a service that has crashed or to scale up or down in response to changing workloads.
- Alerting team members: When issues are detected, AI can be used to alert the appropriate team members, either by sending notifications or by automatically opening a ticket in a support system. This can help to ensure that issues are addressed promptly and that the right team members are aware of the problem.
- Automating routine tasks: In addition to monitoring and responding to issues, AI can be used to automate other routine tasks that are often performed by SREs. For example, AI could be used to automate the process of deploying code updates or to automatically provision and configure new servers or other resources.
Overall, AI has the potential to significantly improve the efficiency of SREs by automating a wide range of routine tasks, freeing up SREs to focus on more complex tasks that require human judgement and expertise.
Predictive maintenance
Predictive maintenance is the process of using data to predict when maintenance will be needed on equipment or systems, allowing maintenance to be scheduled proactively rather than reactively. AI has the potential to significantly improve the effectiveness of predictive maintenance by analyzing patterns in data and predicting when maintenance will be needed. Some ways in which AI could be used for predictive maintenance include:
- Analyzing patterns in data: AI can be used to analyze data from sensors, logs, and other sources to identify patterns that may indicate that maintenance is needed. For example, AI could be used to analyze data from sensors on a fleet of vehicles to predict when maintenance will be needed based on patterns in the data.
- Predicting maintenance needs: Based on the patterns identified in the data, AI can predict when maintenance will be needed and alert SREs or maintenance teams to schedule maintenance proactively. This can help to minimize downtime and prevent issues from occurring.
- Optimizing maintenance schedules: AI can be used to optimize maintenance schedules based on the predicted maintenance needs, ensuring that maintenance is performed at the most appropriate time. This can help to minimize the impact of maintenance on system availability and performance.
- Improving maintenance efficiency: By predicting when maintenance will be needed, AI can help to ensure that maintenance is performed at the most appropriate time, reducing the frequency of unnecessary maintenance and improving the efficiency of maintenance processes.
Overall, AI has the potential to greatly improve the effectiveness of predictive maintenance by enabling SREs and maintenance teams to proactively address potential issues before they cause disruptions.
Root cause analysis
Root cause analysis is the process of identifying the underlying cause of an issue in a system. This can be a time-consuming and complex task, especially in systems with a large number of components or complex configurations. AI has the potential to significantly improve root cause analysis by analyzing large amounts of data from multiple sources and identifying the root cause of an issue. Some ways in which AI could be used for root cause analysis include:
- Analyzing data from multiple sources: AI can be used to analyze data from a wide range of sources, such as logs, metrics, network traffic, and other data, to identify the root cause of an issue. By analyzing data from multiple sources, AI can provide a more comprehensive view of the system and help to identify the root cause of an issue more accurately.
- Identifying patterns in data: AI can be used to identify patterns in data that may indicate the root cause of an issue. For example, AI could be used to analyze data from a cloud computing platform to identify patterns that may indicate the root cause of an outage.
- Suggesting possible root causes: Based on the patterns identified in the data, AI can suggest possible root causes of an issue and provide evidence to support each suggestion. This can help SREs to quickly narrow down the possible root causes and focus on finding a solution.
- Improving root cause analysis efficiency: By automating the process of analyzing data and suggesting possible root causes, AI can significantly improve the efficiency of root cause analysis, allowing SREs to identify the root cause of an issue more quickly and with less effort.
Overall, AI has the potential to greatly improve the efficiency and effectiveness of root cause analysis by automating the process of analyzing data and identifying the root cause of an issue.
Performance optimization
Performance optimization is the process of improving the performance of a system by identifying and addressing bottlenecks or other issues that may be affecting performance. AI has the potential to significantly improve performance optimization by analyzing data and identifying opportunities for improvement. Some ways in which AI could be used for performance optimization include:
- Analyzing data to identify performance issues: AI can be used to analyze data from a wide range of sources, such as logs, metrics, and network traffic, to identify bottlenecks or other performance issues that may be affecting the system. By analyzing data from multiple sources, AI can provide a more comprehensive view of the system and help to identify performance issues more accurately.
- Suggesting optimization strategies: Based on the performance issues identified, AI can suggest optimization strategies that may improve system performance. For example, AI could suggest changes to the configuration of a database or the allocation of resources in a cloud computing platform to improve performance.
- Evaluating the impact of optimization strategies: AI can be used to evaluate the impact of optimization strategies by analyzing data before and after the changes are made. This can help SREs to determine whether the optimization strategies are effective and whether further optimization is needed.
- Continuous optimization: AI can be used to continuously monitor systems and identify new opportunities for optimization as they arise. This can help to ensure that systems are always running at peak efficiency.
Overall, AI has the potential to greatly improve the effectiveness of performance optimization by automating the process of analyzing data and identifying opportunities for improvement. This can help SREs to optimize their systems more effectively and deliver the best possible performance to users.
Benefits of AI for Site Reliability Engineers
There are a number of potential benefits for Site Reliability Engineers (SREs) to utilizing AI in their work:
- Improved efficiency: AI has the potential to significantly improve the efficiency of SREs by automating a wide range of routine tasks, such as monitoring systems for issues, alerting team members when issues are detected, and responding to issues automatically. This can free up SREs to focus on more complex tasks that require human judgement and expertise.
- Enhanced prediction and prevention: AI can be used for predictive maintenance to predict when issues are likely to occur and for root cause analysis to identify the underlying cause of an issue. By predicting and preventing issues before they occur, AI can help to minimize downtime and improve the reliability of systems.
- Improved performance optimization: AI can be used to identify opportunities for improving system performance and suggest optimization strategies, allowing SREs to optimize their systems more effectively. This can help to deliver the best possible performance to users and improve the overall user experience.
- Enhanced data analysis: AI has the ability to analyze large amounts of data from multiple sources, which can be particularly useful for identifying patterns and trends that may not be apparent to SREs. This can help SREs to gain a deeper understanding of their systems and make more informed decisions.
- Increased reliability: By automating routine tasks and predicting and preventing issues, AI can help to improve the reliability of systems, reducing the number of disruptions and improving the overall quality of service.
- Improved problem-solving: By automating the process of analyzing data and suggesting possible root causes and optimization strategies, AI can help to improve the efficiency of problem-solving, allowing SREs to identify and address issues more quickly.
Overall, the use of AI has the potential to significantly improve the efficiency and effectiveness of SREs, enabling them to better manage and maintain complex systems.
Will AI replace SRE jobs?
It is unlikely that the use of Artificial Intelligence (AI) will completely replace the need for Site Reliability Engineers (SREs). While AI has the potential to automate a wide range of tasks and improve the efficiency of SREs, there are still many tasks that require human judgement and expertise. For example, SREs are responsible for designing, implementing, and maintaining complex systems, which often requires a deep understanding of the system and its components. In addition, SREs are often called upon to troubleshoot complex issues and make decisions about how to best resolve problems. These tasks are not easily automated and require human expertise and judgement.
Furthermore, AI systems require human oversight and maintenance, and SREs will continue to play a critical role in managing and maintaining these systems. AI systems can be complex and may require regular updates and maintenance, and SREs will be responsible for ensuring that these systems are operating correctly and delivering the desired performance.
Overall, while AI has the potential to greatly assist SREs and improve the efficiency of their work, it is unlikely to completely replace the need for human SREs in the near future.
About the Author
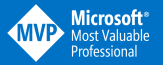
Chris Pietschmann
Chris Pietschmann is a Microsoft MVP (Azure & IoT) and HashiCorp Ambassador (2021) with 20+ years of experience designing and building Cloud & Enterprise systems. He has worked with companies of all sizes from startups to Fortune 100. He is also a Microsoft Certified Azure Solutions Architect and developer, a Microsoft Certified Trainer (MCT), and Cloud Advocate. He has a passion for technology and sharing what he learns with others to help enable them to learn faster and be more productive.
Recommend
About Joyk
Aggregate valuable and interesting links.
Joyk means Joy of geeK