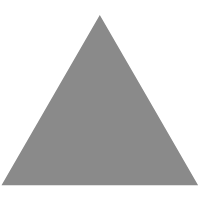
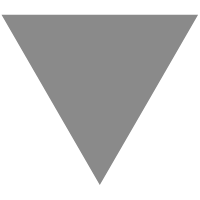
Will AI target your job next?
source link: https://aifuture.substack.com/p/will-ai-target-your-job-next
Go to the source link to view the article. You can view the picture content, updated content and better typesetting reading experience. If the link is broken, please click the button below to view the snapshot at that time.
Will AI target your job next?
A new way to predict how close we are to automation
Yesterday OpenAI announced the commercialization of DALL·E 2. In my last article, I dissected how DALL·E 2 is OpenAI’s opening salvo against Google and is primed to usher in a new AI workforce. Now anyone can sign up to “employ” DALL·E 2 as their business’ graphic designer. With this move, DALL·E 2 is ready to take on Google in this new commercialization of AI workforce tools.
What started as a free (yet limited) tool was OpenAI’s strategic move to gather millions of users and data. This set up the pathway for their latest enterprise solution opening up a 100 billion-dollar market. And it also spells the beginning of the end for a human design workforce as AI will eventually replace low-to-medium-skilled graphic designers. In the not-so-distant future, all types of workers will be displaced as AI upends industries.
Are you going to be replaced by an AI? The short answer is: yes–it’s only a matter of time. The long answer is more complicated as it varies on the type of work you do and the rate of AI development. And, how can we predict when specific jobs will be automated by AI? To answer that we need to travel around the world, figuratively.
Subscribe to AI Future for free to receive new posts.
The future comes faster than you think
Humans are great at extrapolating linearly. We can easily judge the distance of 30 linear steps across a room. But, where would you be if you took 30 exponential steps? It gets a lot more difficult to estimate in this case. In 30 exponential steps, you could have circumnavigated the Earth 26 times!
Here is another way to look at exponential steps. Imagine each of the circles in the chart below are like tsunami waves. You don’t see the tsunami until the water starts to recede and then boom!
Even though each row is equally spaced at 10 exponential steps apart, the tipping point occurs from 20 to 30 exponential steps and then all of a sudden you get a tsunami wave hitting the shore. Exponential progress is imperceptible–until it’s too late. And just like a tsunami, it forever changes the landscape.
Once an AI gets just 1% efficient at automation, it achieves 100% efficiency quickly thereafter.
Take Google’s AlphaGo for example. It took years for AlphaGo to get to human-level performance in the game of Go in order to beat a level 2 master. It finally did so in October 2015. Not but five months later it had improved so significantly that it beat the best player in the world (at level 9). Nine months after that endeavor, a successive AI named AlphaGo Zero beat the original AlphaGo with a score of 100 to 0. The kicker? It only took 21 days to train AlphaGo Zero.
Most of us get stuck thinking linearly. If you asked me last year whether AI could offset 10% of the work that visual designers undertake, I would have thought it very unlikely. Right now most people think it would be absurd for Github’s Copilot or DeepMind’s AlphaCode to perform 80% of software development tasks in the next five years. Most experts correctly point out all the shortcomings of a system and the challenges that remain.
But, they fail to take into account two exponential drivers:
1) The 60% decrease in AI computing hardware every year which translates to the ability to train exponentially larger AI models (ARK Invest 2022 Big Ideas Report).
2) The constant stream of new AI advancements on the algorithm and software side leading to its own exponential gains in AI benchmarks.
These two exponentials are building upon one another to create a monumental tsunami. Just look at how quickly new AI models perform on the gold-standard benchmark (MMLU).
Now you’re probably asking yourself: How do these examples of software improvements apply to my job? Let's apply exponential thinking and the rapid progress of AI to the labor economy.
The Tipping Point
Let’s start with a thought experiment. Will a state-of-the-art AI such as Google’s PaLM be able to automate 5% of content writing or customer support work? If so, would writers and customer support reps start losing their jobs?
What about when the AI can automate 50%?
At some point along the continuum, there will simply not be enough new work to make up for the efficiency gains by the AI models. To top it off, AI is tackling many of these creative job functions in parallel. That won’t leave enough time for the majority of people to reskill before those other jobs are also displaced. When the AI automation level passes the tipping point (red dashed line below), workers will be displaced because there simply won’t be enough jobs left for humans to populate–AI would have taken them over at that point.
Each job function’s tipping point will vary based on the job supply and demand. Let’s dive in…
Software Developers
Software developer jobs are poised to grow 22% through 2030 according to the U.S. Bureau of Labor and Statistics. Let’s assume that will be annualized growth, to be extra generous to the idea that AI will enable the creation of many new jobs as new possibilities open up. That’s a ~5x cumulative growth, which equates to an AI needing to automate 80% of work by 2030 to cross the job supply-demand tipping point.
OpenAI estimates that OpenAI Codex (what powers Github’s Copilot) can already complete 37% of coding tasks. DeepMind’s non-commercial AlphaCode is a whopping 59% better at coding competitions than Codex. (That being said, it’s not commercially available yet so I am going to be conservative and not factor it in.). Let’s ratchet down OpenAI’s estimate and assume that Codex can automate 20% of human software developer tasks today. Here’s what this looks like:
Graphics Designers
Graphics design jobs are poised to grow 3% through 2030. Again to be generous, applying an annual growth of 3% leads to a 26% cumulative growth. That equates to an AI needing to automate only 21% of work by 2030 to cross the job supply-demand tipping point. Since OpenAI’s DALL·E 2 was just released yesterday for commercial use, I have marked the point for “today” at 10%.
Writers
Writing jobs are poised to grow 9% through 2030. If we annualize that number, that’s roughly a 50% cumulative growth. That equates to an AI needing to automate 50% of work by 2030 to cross the job supply-demand tipping point. The 300+ startups and apps leveraging OpenAI’s GPT-3 commercially are largely focused on writing applications. This maturation means we are slightly further up the curve compared to design AIs like DALL·E 2.
You might still not be convinced your job will go away for a variety of reasons…
Objection #1: AI lacks the human touch.
“But what about the human touch and perspective?” Many would argue DALL·E 2 is showing brief glimpses of perspective, and certainly a lot of ingenuity. What was considered impossible is starting to become possible. Just look at the image below.
The AI “understands” the following:
The physics of sunlight and the light shining on her face.
Humans have a certain look on their faces when they concentrate on tasks.
It did all of the above and created multiple image variations–all in the span of 20 seconds!
Objection #2: AI is complementary and will allow humans to focus on high-value work.
This objection goes like this, “AI will just automate my low-value tasks. That will free up my time to do more high-value work for my company.” This sounds reasonable at first but fails to consider the labor market dynamics. Such a situation only works up to the point where a company has enough work to go around. For developers, there is a large gap in the market currently. But for designers, that gap is small as we saw above.
Objection #3: AI improvements will hit a ceiling.
One might point out that AI improvements will hit a ceiling that is beneath the level of human capability. Of all the arguments, I believe this is the most credible one, but still unlikely to happen. All the large language AI models have shown no signs of slowing down in terms of performance improvements. And, it seems unlikely that they will peeter out under the level of your average human performance.
Objection #4: Humans can re-skill into jobs we can’t yet imagine.
Good point. But, can we reskill millions of people faster than AI can learn those new jobs? I don’t think we can en masse–especially with the education-to-career pathway as long and broken as it is. Maybe a small minority of humans will persevere to learn a new skill and effectively apply it to the labor force, but that too will be short-lived as human performance peaks while AI keeps improving ad infinitum.
Which jobs are affected next?
A few years ago I was sitting in a lecture hall at Stanford University listening to Dr. Vivienne Ming, an AI expert, discuss the future of work. She said, “The last thing an AI or robot will solve is to walk to a strawberry field, pick the strawberries, and place it on a farmstand.” In other words, and paradoxically, work in labor-intensive jobs will be the last to be automated. Many used to think those jobs would be the first in line for automation, and creative jobs will be automated last. But that has not proven out. This is now becoming the new order of AI automation:
Creative jobs like software development, graphics design, writing, photography, etc.
Professional jobs like accountants, lawyers, project managers
Jobs requiring movement and dexterity with your hands, arms and/or legs
What this all means
The nature of deceptive exponential progress might leave one dismissive of new technological developments or simply unaware of them. But, by thinking exponentially you can be better prepared for the future. In your job, investments, and business ask whether the new innovation is going to benefit from exponential changes. If so, is it in the early innings where progress looks flat? Or is it further along the curve?
While society faces some imminent challenges on the job front I am very optimistic about the role AI can have on humanity overall. We don’t even need artificial general intelligence (AGI), in order to achieve meaningful human progress. Transformative advances can still happen with near-term AI like improved batteries to combat climate change, faster and better drug discovery, and vertical farming to help reduce water and fertilizer waste. In fact, those advances are already happening today. One thing’s for sure though, artificial intelligence will be a hell of a tsunami.
Up next, I’ll introduce my 3 AI paradigms and how to value the market opportunities. If you enjoyed reading this post, please subscribe below. See you in the future!
Thanks for reading AI Future! Subscribe for free to receive new posts and support my work.
If you loved this post, please share it with 5 of your friends and see what they think.
Recommend
About Joyk
Aggregate valuable and interesting links.
Joyk means Joy of geeK