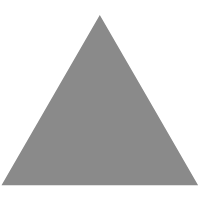
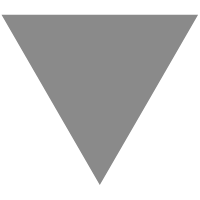
GAN(七)——GAN参考资源(3)
source link: http://antkillerfarm.github.io/gan%20&%20vae/2021/01/07/GAN_7.html
Go to the source link to view the article. You can view the picture content, updated content and better typesetting reading experience. If the link is broken, please click the button below to view the snapshot at that time.
GAN参考资源
https://zhuanlan.zhihu.com/p/41114883
手机照片脑补成超大画幅,这个GAN想象力惊人
https://mp.weixin.qq.com/s/bKve_tZi9usz4oX0T3S15A
悉尼大学陶大程:遗传对抗生成网络有效解决GAN两大痛点
https://zhuanlan.zhihu.com/p/46629127
生成对抗网络-GAN—一个好老师的重要性
https://mp.weixin.qq.com/s/Sp0EYvaq-1u0mtnrrmFNCQ
为什么说GANs是一个绝妙的艺术创作工具?
https://mp.weixin.qq.com/s/uHEAtuY1_KZdUAdDAwFi_A
以为GAN只能“炮制假图”?它还有这7种另类用途
https://mp.weixin.qq.com/s/Yf5quOXmzJAy0GnJnvam5g
台湾学者研发新型二元神经元GAN!有望用于AI作曲
https://mp.weixin.qq.com/s/8aL7COItG7lS4q5-3IZCmQ
定制人脸图像没那么难!使用TL-GAN模型轻松变脸
https://mp.weixin.qq.com/s/9t0GvQW-cmakM0E9dWxBcg
旧照片着色修复神器!自注意力GAN效果惊艳
https://mp.weixin.qq.com/s/cUFQ6EADa39h2eFoa_Dh0A
最高76%破解成功率!GAN已经能造出“万能指纹”,你的手机还安全吗?
https://mp.weixin.qq.com/s/_tABIMkWX8L5xQFmvPI7rw
有效稳定对抗模型训练过程,伯克利提出变分判别器瓶颈
https://mp.weixin.qq.com/s/xr9fDv9DFkwi2ImV4RZAHg
换个角度看GAN:另一种损失函数
https://mp.weixin.qq.com/s/U1rrPfJDLgXHRj__XwrMZw
只有条件GAN才能稳定训练?对抗+自监督的无监督方法了解一下
https://mp.weixin.qq.com/s/xHKQlFFkBQLBg2GdZuGPSw
提升GAN训练的技巧汇总
https://mp.weixin.qq.com/s/ctB90bNhaMYvbLE4yketHQ
一文读懂对抗机器学习Universal adversarial perturbations
https://mp.weixin.qq.com/s/zRNtEKS2dKxyQU4VZm6Mwg
用GANs来自动生成音乐
https://mp.weixin.qq.com/s/zwzl-Tel3Avc4Dm7L5FS5A
NLP中的对抗训练+PyTorch实现
https://mp.weixin.qq.com/s/Ze2BXEexTIpNluRSdfeCsA
GAN和PS合体会怎样?东京大学图像增强新研究:无需配对图像,增强效果还可解释
https://mp.weixin.qq.com/s/qLhnvLhXHhRoPGtPWYCY0w
ICCV2019最佳论文SinGAN全面解读
https://zhuanlan.zhihu.com/p/97138846
《SinGAN:从单个自然图像中学习生成模型》论文笔记
https://mp.weixin.qq.com/s/mXjNtZvUHutBABh-f9qisQ
那些底层的图像处理问题中,GAN能有什么作为?
https://mp.weixin.qq.com/s/zVGDYhBiLCNhKTnkzbMxGA
时间序列GAN,Subadditivity of Probability Divergences
https://mp.weixin.qq.com/s/ssD3NAvGx5Eu4-oWy4KtzA
如何生动有趣地对GAN进行可视化?
https://mp.weixin.qq.com/s/qz4wUpSeF8Nlem8x4CrR-Q
学习一个宫崎骏画风的图像风格转换GAN
https://zhuanlan.zhihu.com/p/50790727
SeqGAN: Sequence GAN with Policy Gradient
https://mp.weixin.qq.com/s/bH5yYbwq6NGQJ84xUDhoxg
生成对抗网络在图像翻译上的应用
https://mp.weixin.qq.com/s/3Gsmrl4HbcnXpje0nyAq2w
中国西北大学和北京大学的研究结果是否将终结CAPTCHA验证码时代?
https://zhuanlan.zhihu.com/p/53260242
抛开复杂证明,我们从直觉上理解W-GAN为啥这么好训
https://mp.weixin.qq.com/s/FJA8Tctq_p4Mj-KgNn_OGg
为什么让GAN一家独大?Facebook提出非对抗式生成方法GLANN
https://mp.weixin.qq.com/s/SGCoCy8wJEhEpLSHrYK3pQ
韩松、朱俊彦等人提出GAN压缩法:算力消耗不到1/9,现已开源
https://mp.weixin.qq.com/s/D-rh9m7G-ERjWEEG6BQJNg
每个人都能用英伟达GAN造脸了
https://mp.weixin.qq.com/s/qRW344wWgS9PJ6UCwknS8g
Local GAN
https://mp.weixin.qq.com/s/-rwzlX-WipaIdV6ESOimxw
GAN加持!英伟达发布“山寨”游戏创造器,已完美复现《吃豆人》
https://mp.weixin.qq.com/s/orm5r4XHyotBCpBKqfZ-ng
GANSynth:使用GAN制作音乐
https://mp.weixin.qq.com/s/4zKgFfyLGAmqgHtf_Wb0nw
使用GAN生成序列数据
https://mp.weixin.qq.com/s/oRCbr0TCzFmTuf5jpWKBaA
GAN版马里奥创作家来了:一个样本即可训练,生成关卡要素丰富
https://mp.weixin.qq.com/s/2NrPolWxV-L-dFUXgGOd9w
在GAN中通过上下文的复制和粘贴,在没有数据集的情况下生成新内容
https://mp.weixin.qq.com/s/iqCMA7E_vtdymVxxz7bpRA
生成对抗网络(GAN)的数学原理全解
https://mp.weixin.qq.com/s/D0gLR6YU3rYTbFqSCwyi9Q
Semi-Supervised GAN
https://mp.weixin.qq.com/s/MY-nx_MDyBJHUidS3Xqs7g
最新《生成式对抗网络GAN进展》综述论文,41页pdf阐述GAN在计算机视觉应用进展
https://mp.weixin.qq.com/s/fQiZpUbeYvFnNZYYSE4dMQ
用GANs来做数据增强
https://mp.weixin.qq.com/s/KT_YHNLGdOI-mr4EnYgGvw
使用有限的数据来训练GANs
https://mp.weixin.qq.com/s/_cKtmNZbqHwZszocPXuy8g
让GAN随音乐律动的Python工具,网友:这是我见过的GAN的最佳用法
https://mp.weixin.qq.com/s/skDZcvuek3pAV1U07aORVA
改善图像处理效果的五大生成对抗网络
https://mp.weixin.qq.com/s/a1f_8wP0bMyNf99ihaCISg
对抗学习如何应用到推荐系统?ECIR2021<对抗学习推荐系统>教程,197页ppt
https://mp.weixin.qq.com/s/n8c2-NPtVe-k2L6qvEHsUA
改善图像处理效果的五大生成对抗网络
https://mp.weixin.qq.com/s/imOFQL01RaXUuZwew1izzg
基于GAN来做低光照图像增强,EnlightenGAN论文解读
VAE参考资源+
https://mp.weixin.qq.com/s/t4YYIl4o_TAPG7737ZfiaA
面向无监督任务:DeepMind提出神经离散表示学习生成模型VQ-VAE
https://mp.weixin.qq.com/s/51Xu7osdVa-fCV-IZbHdCA
Wasserstein自编码器
https://mp.weixin.qq.com/s/0HK026K6jru10VscvT2rOQ
哈佛大学提出变分注意力:用VAE重建注意力机制
https://mp.weixin.qq.com/s/790wbFnxkNbNRampiV-0MQ
谷歌大脑提出对抗正则化方法,显著改善自编码器的泛化和表征学习能力
https://mp.weixin.qq.com/s/iOdh1iIP0GIYe4gRDE0z-g
漫谈概率PCA和变分自编码器
https://mp.weixin.qq.com/s/pBnKNRc56HhBWvrYaZjGdw
稳定、表征丰富的球面变分自编码器
https://mp.weixin.qq.com/s/QOdQKdLolR-YTihzaA81yw
黄怀波 :自省变分自编码器理论及其在图像生成上的应用
https://mp.weixin.qq.com/s/FqY9I02blg3S8_K50B7czQ
UC伯克利提出小批量MH测试:令MCMC方法在自编码器中更强劲
https://zhuanlan.zhihu.com/p/48985202
谈谈变分自编码器背后的数学知识
https://mp.weixin.qq.com/s/fYR2dS3wCMMVk3s9O4nqUw
自编码表示学习 25页最新进展综述,90篇参考文献
https://zhuanlan.zhihu.com/p/52974147
VAE的细节:p(x∣z)的噪音,与p(z∣x)的编码坍塌
https://mp.weixin.qq.com/s/uNjF6NxVRs_gAKAmxRpThQ
华为美研所提出自动编码变换网络AET:用无监督逼近全监督效果
https://zhuanlan.zhihu.com/p/60330303
用于协同过滤的变分自编码器论文引介
https://mp.weixin.qq.com/s/CSx7dnqPjVDAvM6ena-FTw
从俄罗斯方块到星际2,全都用得上:DeepMind无监督分割大法,为游戏而生
https://mp.weixin.qq.com/s/XMLYjw_wN-M8jluczkbcyw
一种考虑缓和KL消失的简单VAE训练方法
https://mp.weixin.qq.com/s/0coPJFrW7anW7QuqjPBt3g
深度生成模型在信号处理领域的应用
https://mp.weixin.qq.com/s/GJr-YtV84eV1KbkyVSkcBA
超越BigGAN,DeepMind提出“史上最强非GAN生成器”VQ-VAE-2
https://mp.weixin.qq.com/s/3ZHlWlO0FdnQTnskcrz2IA
生成式模型入门:训练似然模型的技巧
https://mp.weixin.qq.com/s/KtKYk08xEqwA43Phxw2-_w
基于自编码器的通用性文本表征
https://mp.weixin.qq.com/s/hwb3eRWbwa9weCWz1ypOxw
VAE系解纠缠:从VAE到βVAE,再到β-TCVAE
https://mp.weixin.qq.com/s/YZcw5pnHzuACSvEmGZHnEQ
自回归模型:PixelCNN
https://mp.weixin.qq.com/s/yB7tq2vcBpVBnM-j9PgRsw
人脸合成效果媲美StyleGAN,而它是个自编码器
https://mp.weixin.qq.com/s/J59d1K5WX-wnzhws3k60AQ
万字长文带你了解变分自编码器VAEs
https://mp.weixin.qq.com/s/g-OPU4vp66HWcpqjdD2bBg
使用PyTorch从理论到实践理解变分自编码器VAE
https://mp.weixin.qq.com/s/8zi0JrloxuRdHcRm1dzCEQ
利用VAE和LSTM生成时间序列
目标检测进阶+
https://mp.weixin.qq.com/s/kz74yb857gmG1h7MyOWx_Q
目标检测正负样本区分策略和平衡策略总结(二)
https://mp.weixin.qq.com/s/gJuXl6Oh_N_fJgQ9jT268w
旷视研究院提出密集场景检测新方法:一个候选框,多个预测结果
https://mp.weixin.qq.com/s/iPx5K2cSs5jlCt9d9oKpww
VoVNet:实时目标检测的新backbone网络
https://mp.weixin.qq.com/s/FHHIdoXyaU4skX-zyZFQew
已开源!全新水下目标检测算法SWIPENet+IMA框架
https://mp.weixin.qq.com/s/-x9A1eG5BfGmqV31SdMCMg
目标检测算法之Light-Head R-CNN
https://mp.weixin.qq.com/s/On-pHCdGg3piTQtDV88kQA
特定场景下Yolo改进算法:Poly-Yolo
https://mp.weixin.qq.com/s/HW0SNf-NYjXqJwlungRFpg
综述:目标检测中的多尺度检测方法
https://zhuanlan.zhihu.com/p/158907507
From VanillaDet to AutoAssign
https://mp.weixin.qq.com/s/GL_q4VLCgbjTZq_zpTLq0w
Label Assign:提升目标检测上限
https://mp.weixin.qq.com/s/EO5h1zW4yrToqwTCnXtz4g
TTFNet: 最大程度提高训练效率的实时目标检测
https://mp.weixin.qq.com/s/6R3myLyAeMMS0lR1GSlvcA
细说物体检测中的Anchors
https://mp.weixin.qq.com/s/psXJNlEawZlQ-ZdktDpjOw
目标检测小tricks之样本不均衡处理
https://zhuanlan.zhihu.com/p/54334986
TridentNet:处理目标检测中尺度变化新思路
https://mp.weixin.qq.com/s/eODjZUQQiROID9i4WOeEFQ
三分支网络——目前目标检测性能最佳网络框架
https://mp.weixin.qq.com/s/reNCLvOyJHkJZimnMpbIig
目标检测算法优化技巧:Bag of Freebies for Training Object Detection
https://mp.weixin.qq.com/s/BXwL33qOf3f7BtJvHsi23Q
目标检测:Segmentation is All You Need?
https://mp.weixin.qq.com/s/NWILStthG4klkwrYVcGQSQ
ILC:用于自然场景多目标的计数模型
https://zhuanlan.zhihu.com/p/59915784
目标检测中的Consistent Optimization
https://mp.weixin.qq.com/s/ts4WFnuN4cHLfUh8–96Kw
Libra R-CNN:全面平衡的目标检测器
https://mp.weixin.qq.com/s/groq55Cbts272k1mfhJwaQ
超越bounding box的代表性点集:视觉物体表示的新方法
https://mp.weixin.qq.com/s/vy8xlxGY8-m7F5GQetlQeg
SaccadeNet:使用角点特征进行two-stage预测框精调
https://mp.weixin.qq.com/s/N8vjh3HPWOfxo7ZdOr_eOg
PAA论文解读:在物体检测中利用概率分布来将anchor分配为正负样本
https://mp.weixin.qq.com/s/nTzTjUDQc3jTu3AAQ1Hvbg
理解物体检测中的Objectness
Recommend
About Joyk
Aggregate valuable and interesting links.
Joyk means Joy of geeK