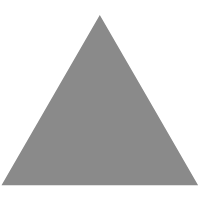
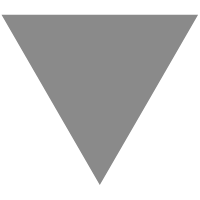
Optimizing shoppers’ journey with a headless approach to AI
source link: https://www.algolia.com/blog/ai/optimizing-shoppers-journey-with-a-headless-approach-to-ai/
Go to the source link to view the article. You can view the picture content, updated content and better typesetting reading experience. If the link is broken, please click the button below to view the snapshot at that time.
Optimizing shoppers’ journey with a headless approach to AI
Jan 26th 2021
Winning in today’s ultra competitive online world is all about creating an experience that is even better than what someone would experience when they walk into a store and talk to your best salesperson. Something that is effortless for the shopper and personalized to their needs.
Companies use many approaches to create these personalized experiences, including letting shoppers self declare their preferences and demographics, or buying third party data. More recently, AI techniques have been added to the mix. However, creating a delightful experience can’t be achieved by throwing a bunch of AI tools at the problem. When not well configured, AI optimizations, including personalizations can become creepy. Personalization that follows consumers across their online activities, outside of a given brand’s properties, or that is too strong, will deter those consumers. 63% of them say they would stop purchasing products and services from companies that take “creepy” marketing too far. AI optimization applied too strongly will not serve the experience, but rather detract from it, preventing users from discovering and enjoying the breadth of what you have to offer.
The need for in-session optimization
The last thing you want to do is offer your online shoppers an experience that is either clunky or outdated, such as pushing them a pair of sneakers they researched a week ago but ended up buying on another website, or endlessly promoting them smartphone cases after they’ve looked at the latest smartphone once, just out of curiosity.
Such suboptimal experiences result in shoppers clicking their way to another retailer.
And that’s when the retailers’ website remembers the shopper – or the shopper lets it remember them. When shoppers block cookies, don’t login before checking out, or simply change devices, most online shopping experiences get back to being generic, without any context about the shopper or what they’re interested in…
As global data privacy regulations become more restrictive, consumers insist on privacy, and large players like Apple drive initiatives to secure personal data and promote privacy rights, retailers will lose the ability to optimize and personalize digital experiences using existing approaches i.e. based on historical user data.
As a retailer, you need a new way to augment experiences for your shoppers. You’ll need to predict a shopper’s intent based on their very first actions in a session on a digital storefront, and optimize the experience based on this intent, in almost real time.
AI and ML can help retailers achieve this new way, by analyzing shoppers’ actions, assigning those users to a segment in real time, and optimizing the experience accordingly.
For AI: one size does not fit all
Achieving this vision requires harnessing the power of multiple ML models: : one to segment users based on the categories they visit, one based on the search query they type, one based on the product pages they view, then one to reconcile everything, one to optimize the search results accordingly, one to provide the right recommendations on each visited product page accordingly, etc etc.
And that’s for just one experience in one channel. Shoppers’ behaviors will be different on mobile, requiring different models. And what about voice assistants, in-store kiosks, even clienteling apps for your stores associates, that would also benefit from real-time AI optimizations.
All those different experiences on different channels, represent various steps in the shoppers journey, that each need their specific Machine Learning models to achieve satisfying results.
AI is hard
Unfortunately, creating these comprehensive experiences, responsive to shoppers’ intent, is impossible for most retailers.
On one hand, there are packaged, off-the-shelf AI approaches that address one specific step of the shoppers’ journey. But, these approaches leave little to no room for customization or fine-tuning, and are difficult to connect with other parts of the journey. It prevents retailers to leverage the outcomes of their AI and ML models from one part of the journey to the other.
On the other hand, creating and optimizing all these AI/ML models from scratch is out of reach for most retail companies. It requires assembling the perfect team of data scientists and Machine Learning engineers, and repeating the development work for each and every model.
Applying headless commerce to AI
Between those two ends of the spectrum, there is another way. Just like the monolithic ecommerce tech stack has been deconstructed into best of breed building blocks for order management, payment, marketing, emailing, etc, AI can be deconstructed in best of breed models to enhance specific parts of the shoppers’ journey.
Algolia AI Studio brings to AI what Headless Commerce brought to ecommerce tech stacks. A suite of AI Building Blocks that cover every step of the shoppers journey. Packaged into APIs, those models can be easily implemented into any website or application by developers. It streamlines the deployment of AI throughout the user journey into just three main steps: selecting the right AI model for the right use case, connecting the data sources to feed this model, and connecting the experience where the output of the AI model will be activated.
Algolia AI Studio comes with three main families of models:
Intent-based Search and Navigation
It identifies users’ shopping intent and shows them the right products whether they search or browse your digital storefront, or even interact with your voice assistant application.
Intrend, one of the most influential Italian factory outlets, increasing their average order value by 20% using Intent-based Search and Navigation.
Intent-based recommendations
It predicts right products to proactively showcase to shoppers based on their intent and context, such as a product page or a checkout page.
A retailer that developed an advanced content strategy, with buying guides for instance, could pull a recommendation API on their editorial content pages to showcase products relevant to this buying guide, and the actions the shopper reading the guide took in their current session.
Intent-based user segmentation
It classifies shoppers based on their predicted intent, which then triggers a series of predefined/orchestrated (sophisticated) scenarios to move the shopper forward in their shopping journey. This could include a likelihood to purchase, a prediction for cart abandonment, as well as other user journey attributes.
Mobexpert, a leading home and furniture retailer in Eastern Europe, already leverages Intent-Based User Segmentation to identify shoppers with a medium probability of purchasing, and push those shoppers discounts and free shipping to incentivize them to buy. They were able to double their conversion rate and increase their average order value by 45%
Recommend
About Joyk
Aggregate valuable and interesting links.
Joyk means Joy of geeK