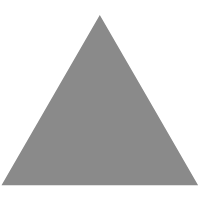
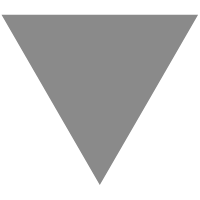
OpenHarmony AI推理任务管理与统一推理接口介绍
source link: https://os.51cto.com/article/705067.html
Go to the source link to view the article. You can view the picture content, updated content and better typesetting reading experience. If the link is broken, please click the button below to view the snapshot at that time.
AI推理任务管理与统一推理接口提供了在OpenHarmony标准系统上基于CPU进行AI推理任务调度管理的能力,对AI算法能力进行生命周期管理和按需部署,同时,提供适配不同推理框架层级的统一推理接口,基于NCNN、MNN、Paddlelite三大常用端侧推理框架进行了接口的统一封装。基于统一接口,开发者不需再关心不同推理框架API的差异,可以在各框架之间自如切换,同时,提供了端侧框架的编译脚本,开发者可以直接编译、调用这三个框架,代码仓库地址如下:
图 1 AI推理任务管理与统一推理接口架构图:
各模块介绍:
- 任务调度:任务创建、任务分发、任务销毁。
- 框架调度:推理框架加载、卸载。
- 算法引擎:推理算法加载、执行、卸载,推理结果管理。
- 接口管理:对应用层提供统一的框架层接口。
框架适配层:适配不同的第三方推理框架,屏蔽接口差异,为算法引擎提供统一的接口。
./foundation/ai_std
├── interfaces #对外接口定义
├── services
│ ├── client #应用层接口
│ ├── common #公共接口
│ │ ├── platform
│ │ │ ├── dl_operation #dl接口封装
│ │ │ ├── event #事件管理
│ │ │ ├── lock #读写锁管理
│ │ │ ├── os_wrapper #内核接口封装
│ │ │ ├── queuepool #队列池管理
│ │ │ ├── semaphore #信号量管理
│ │ │ ├── threadpool #线程池管理
│ │ │ └── time #时间接口
│ │ ├── protocol
│ │ │ ├── data_channel #推理数据通道管理
│ │ │ ├── retcode_inner #内部返回值定义
│ │ │ └── struct_definition #内部数据结构定义
│ │ └── utils
│ │ ├── constants #内部常量定义
│ │ ├── encdec #数据序列化与反序列化
│ │ ├── file_operation #文件操作接口封装
│ │ ├── inf_cast_impl.h #对象类型转换
│ │ ├── infer_guard.h #堆内存释放管理接口
│ │ ├── infer_macros.h #公共宏定义
│ │ └── log #log接口定义
│ └── server
│ | ├── engine #推理引擎管理
│ | └── plugin_manager #框架调度管理
│ ├── frwkAdapter
│ │ ├── ai_interpreter.cpp #统一推理接口
│ │ └── src
│ │ ├── common #框架适配器公共函数
│ │ ├── mnn #mnn框架适配
│ │ ├── ncnn #ncnn框架适配
│ │ └── paddlelite #paddlelite框架适配
└── test #测试代码
图 2 类关系图:
主要类的功能:
- AiInference:对应用层开放的接口create、SyncInfer、Destroy都定义在该类中。
- ClientFactory:客户端工厂类,整合应用数据调用、管理session、管理serviceAdapter对象的创建和销毁。
- ServiceAdapter:推理服务适配器,为推理任务分配clientId,维护客户端与服务端连接ID,创建推理request,由推理response获取推理结果tensor。
- ServiceExecutor:维护推理引擎管理器的创建与销毁。
- EngineManager:管理推理引擎的创建与销毁。
- Engine:推理引擎,推理的主要功能都由它管理。
- PluginManager:管理框架调度器的创建与销毁。
- Plugin:管理第三方框架与推理模型的加载、卸载。
- AIInterpreter:框架适配器接口类,为AI任务调度管理提供统一的接口,屏蔽不同推理框架的差异。
- EngineWorker:推理线程入口函数由该类提供。
- SyncMsgHandler:负责推理请求的发送、推理结果接收。
图 3 AI任务调度管理时序图:
主要流程:
- 推理任务创建流程:首先分配客户端sessionId,服务端分配clientId,由sessionId和clientId组合生成唯一的transactionId,然后根据框架类型和推理网络加载模型、拉起推理线程。
- 执行推理流程:由sessionId和clientId找到上个流程中创建的推理引擎,创建推理request,压入推理线程队列中,等待推理返回的response。
- 推理任务销毁流程:销毁创建流程中申请的资源包括实例化的对象、申请的内存等, 推理线程终止,线程回收,队列回收。
语言限制:C/C++语言。
操作系统限制:OpenHarmony操作系统。
1.实例化类AiInference。
2.调用AiInference的成员函数Create,传入参数frameworkType,推理网络名称,网络模型路径和推理网络版本号(默认值10000001)。各参数说明如下:
- frameworkType:推理框架ID,从如下枚举Framework_type中选取一个推理框架,当前支持AI_FRAMEWORK_ID_NCNN,AI_FRAMEWORK_ID_MNN和AI_FRAMEWORK_ID_PADDLELITE。
- algorithmName:推理的网络名称,如:mobilenetssd,yolov3。
- modelPathInfos:推理网络的模型路径信息,支持网络拓扑结构与权重数据合一和两个数据分开两个文件的场景,对于合一的场景,结构体的成员type取值NET_COMBINED_TYPE,对于两个数据分开文件表示的场景数组modelPathInfos有两个ModelPathInfo元素,两个元素的type字段分别取值NET_TOPO_FILE_TYPE和WEIGHT_FILE_TYPE,path字段为对应的文件路径。ModelPathType为type字段取值枚举,结构体ModelPathInfo为数组modelPathInfos的元素类型。具体使用方法请参考example章节。
- algorithmVersion:推理网络版本号,默认值10000001,当前不关心该参数值。
int32_t Create(int frameworkType, const std::string &algorithmName, std::vector<ModelPathInfo> &modelPathInfos,
long long algorithmVersion=ALGOTYPE_VERSION_DEFAULT);
typedef enum {
AI_FRAMEWORK_ID_INVALID=0, //invalid inference framework
AI_FRAMEWORK_ID_NCNN=1, //ncnn inference framework
AI_FRAMEWORK_ID_MNN=2, //mnn inference framework
AI_FRAMEWORK_ID_PADDLELITE=3, //paddle-lite inference framework
AI_FRAMEWORK_ID_COUNT
}Framework_type;
typedef enum {
NET_COMBINED_TYPE = 0, //topolopy and weight at one model file
NET_TOPO_FILE_TYPE = 1, //file type is neure network inference model topology
WEIGHT_FILE_TYPE = 2, //file type is neure network inference model weight
}ModelPathType;
typedef struct ModelPathInfo {
ModelPathType type; //model file type
std::string path; // model file path regard to file type
}ModelPathInfo;
3.构造推理输入Tensor,调用成员函数SyncExecute执行推理,函数的声明及参数定义如下。输入和输出参数的类型IOTensors定义如下,具体使用方法请参考example章节。
int32_t SyncExecute(const IOTensors &input, IOTensors &output);
using IOTensors = std::vector<IOTensor>;
typedef struct {
std::string name; //network node name
TensorType type; //data type in buffer
TensorLayout layout; //layout of tensor
std::vector<size_t> shape; //shape of tensor for input or output
std::pair<void *, size_t> buffer; //buffer address pointer and size
}IOTensor;
// Tensor type, regard with IOTensor.buffer pointer type
typedef enum {
UINT8 = 0,
INT8 = 1,
UINT16 = 2,
INT16 = 3,
UINT32 = 4,
INT32 = 5,
FLOAT16 = 6,
FLOAT32 = 7,
INT64 = 8,
UINT64 = 9,
}TensorType;
//Tensor layout
typedef enum {
NONE = 0,
NCHW = 1,
NHWC = 2,
NCHWC8 = 3,
ROW_MAJOR = 4,
LSTM_MTK = 5,
HWKC = 6,
HWCK = 7,
KCHW = 8,
CKHW = 9,
KHWC = 10,
CHWK = 11,
NC4HW4 = 12,
}TensorLayout;
```
4.调用成员函数Destroy销毁推理任务。
int32_t Destroy();
example
以下代码片段为使用第三方网络框架NCCN执行网络sequeezenet_v1.1推理的主要部分。
/**
* @brief Set inference input tensor.
*
* @param framework Indicates the inference framework.
* @param inputTensor Indicates the inference input tensor.
* @return Returns {@link AI_RETCODE_SUCCESS} if the operation is successful;
* returns a non-zero error code defined by {@link AiRetCode} otherwise.
*
* @version 1.0
*/
int SetInputTensor(const std::string &framework, IOTensor *inputTensor) {
inputTensor->name = "input";
inputTensor->layout = TensorLayout::NCHW;
int32_t inputSize = 0;
if (framework == "ncnn") {
inputTensor->type = TensorType::FLOAT32;
inputTensor->shape = {1, 3, 227, 227};
inputSize = 227*227*3;
}
int8_t *data = (int8_t *)malloc(inputSize);
if (data == nullptr) {
return AI_RETCODE_NULL_PARAM;
}
memset(data, 1, inputSize);
inputTensor->buffer = std::make_pair((void *)data, inputSize);
return AI_RETCODE_SUCCESS;
}
/**
* @brief Test inference of network squeezenet_v1 in framework ncnn.
*
* @param modelPath Indicates the file path of inference model topo file.
* @param weightPath Indicates the file path of inference model weight file.
* @return Returns {@link AI_RETCODE_SUCCESS} if the operation is successful;
* returns a non-zero error code defined by {@link AiRetCode} otherwise.
*
* @version 1.0
*/
int TestNcnnInfer(const char *modelPath, const char *weightPath)
{
//construct model path param
ModelPathInfo modelPathInfo = {.type = NET_TOPO_FILE_TYPE, .path = modelPath};
ModelPathInfo weightPathInfo = {.type = WEIGHT_FILE_TYPE, .path = weightPath};
std::vector<ModelPathInfo> modelPaths;
modelPaths.emplace_back(modelPathInfo);
modelPaths.emplace_back(weightPathInfo);
//Instance a new inference instantiation
AiInference* pAiInfer = new AiInference();
if (pAiInfer == nullptr) {
return AI_RETCODE_NULL_PARAM;
}
//create a inference engine
int retcode = pAiInfer->Create(AI_FRAMEWORK_ID_NCNN, squeezenet_v1, modelPaths, ncnnVersion);
if (retcode != AI_RETCODE_SUCCESS) {
delete pAiInfer;
return AI_RETCODE_FAILURE;
}
//construct inference input tensors
IOTensor inputTensor;
retcode = SetInputTensor("ncnn", &inputTensor);
if (retcode != AI_RETCODE_SUCCESS) {
delete pAiInfer;
return retcode;
}
IOTensors inputs;
inputs.push_back(inputTensor);
IOTensors outputs;
retcode = pAiInfer->SyncExecute(inputs, outputs);
if (retcode != AI_RETCODE_SUCCESS) {
return AI_RETCODE_FAILURE;
}
//Destroy inference engine
retcode = pAiInfer->Destroy();
if (retcode != AI_RETCODE_SUCCESS) {
LOG_ERROR("inference engine destroy failed, retcode=[%d]\n", retcode);
return AI_RETCODE_FAILURE;
}
DestroyDataInfo(inputs, "input");
DestroyDataInfo(outputs, "output");
return AI_RETCODE_SUCCESS;
}
int main(int argc, char **argv)
{
LOG_INFO("start inference test, para count: %d\n", argc);
int c;
std::string modelPath = "";
std::string weightPaht = "";
std::string framework = "";
int loop_count = 1;
while ((c = getopt_long(argc, argv, short_options, long_options, NULL)) != -1)
{
switch (c)
{
case 'm':
modelPath.assign(optarg);
break;
case 'w':
weightPaht.assign(optarg);
break;
case 'f':
framework.assign(optarg);
break;
case 'l':
loop_count = atoi(optarg);
break;
default:
break;
}
}
int retcode = AI_RETCODE_SUCCESS;
//Different framework use different Model representation
if (strcmp(framework.c_str(), "paddlelite") == 0) {
retcode = TestPaddlelitInfer(modelPath.c_str());
} else if (strcmp(framework.c_str(), "ncnn") == 0) {
retcode = TestNcnnInfer(modelPath.c_str(), weightPaht.c_str());
} else if (strcmp(framework.c_str(), "mnn") == 0) {
retcode = TestMnnInfer(modelPath.c_str());
}
return retcode;
}
本文主要介绍了AI任务调度管理与统一推理框架接口的框架结构、类关系图和时序图,并列出了对应用层开放的接口及使用方法。
Recommend
About Joyk
Aggregate valuable and interesting links.
Joyk means Joy of geeK