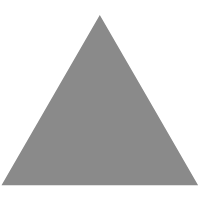
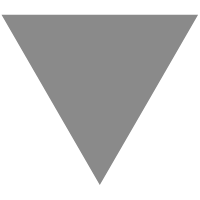
kaggle赛题-临床患者病历评分baseline(NLP)
source link: https://mp.weixin.qq.com/s?__biz=MjM5ODkzMzMwMQ%3D%3D&%3Bmid=2650429622&%3Bidx=5&%3Bsn=ab2238f7d685b4caf602716fa2b5716e
Go to the source link to view the article. You can view the picture content, updated content and better typesetting reading experience. If the link is broken, please click the button below to view the snapshot at that time.
William来源:投稿 作者:William
编辑:学姐
研究生毕业于美国TOP20大学
现就职国内某互联网大厂
赛题分析+baseline
1、赛题链接
https://www.kaggle.com/c/nbme-score-clinical-patient-notes
2、赛题描述
本次竞赛的目标是通过建立一个模型来将不同病情的临床表现在病例中找出,具体而言就是将病情描述+病人病例 一起输入模型, 在病例中提取出对应的span位置。
商业价值:帮助医生可以快速的定位病人的病情,从而对症下药
※ 比赛时间线
2022 2月 1日 年 - 开始日期。
2022 4 月 26 日 年 - 报名截止日期。您必须在此日期之前接受比赛规则才能参加比赛。
2022 4 月 26 日 年 - 团队合并截止日期。这是参与者可以加入或合并团队的最后一天。
2022 5 月 3 日 年 - 最终提交截止日期。
※ 丰厚的奖金
一等奖:15,000美元
二等奖:10,000美元
三等奖:8,000美元
四等奖:7,000美元
五等奖:5,000美元
六等奖:5,000美元
3、数据描述
本次比赛提供了5份数据分别是 train, test, features, patient_notes, submission, 其中test, submission为提交答案时用。
重点是如下3个文件
train文件标记了每个病例中,不同症状的相关描述
features中给出了所有病症的名称和id
patient_notes中给出了每份病例的详细描述
3.1 训练数据分析:
id
- 一个unique 标记符来表示 patient note number - feature number对.pn_num
- patient note number 可以当成病例号.feature_num
- feature number 可以看作不同病症的一个id.case_num
- 病例所属的case id 之后会用来关联起病人patient note的文本描述和对应症状的文本描述.annotation
- patient note中体现相关症状的描述, 一个病例中可能对同一个疾病症状存在多处描述.location
- annotation所在的病例中的char 级别的位置.
Number of rows in train data: 14300
Number of columns in train data: 6
Number of values in train data: 85800
Number of Empty annotions and locations = 4399
在标记数据中,有的病情会在病例中有多处体现,具体的分布如下:
可以看到大部分病情之在病例中有一处对应,大约有4399条在原文中并没有找到对应的部分。
平均annotation 的char 级别长度为16.53,具体的分布如下:
3.2 病例数据分析
Columns Name以其释意
pn_num - patient note 病例id.
case_num - case num 用来关联起病人patient note的文本描述和对应症状的文本描述.
Number of rows in 病例: 42146
Number of columns in 病例: 3
Number of values in 病例: 126438
下面是这个病例的内容
病例的char级别的长度分布如下
3.3 Feature数据分析
feature_num - feature number 可以看作不同病症的一个id.
case_num - 病例所属的case id 之后会用来关联起病人patient note的文本描述和对应症状的文本描述.
feature_text - 疾病文本描述
Number of rows in feature: 143
Number of columns in feature: 3
Number of values in feature: 429
其实feature就是疾病对应的专业描述,像下面这个例子
'Family-history-of-MI-OR-Family-history-of-myocardial-infarction'
病例的char级别的长度分布如下,平均char级别长度为23
micro-averaged:
https://scikit-learn.org/stable/modules/model_evaluation.html#from-binary-to-multiclass-and-multilabel
https://scikit-learn.org/stable/modules/model_evaluation.html#precision-recall-f-measure-metrics
5、构建训练数据下面演示如何将3个数据merge到一起, 具体可以详见baseline代码,里面有更为详细的介绍。
领baseline代码
关注公众号
回复“top”添加小享领取
import pandas as pd
import ast
train = pd.read_csv('train.csv')
train['annotation'] = train['annotation'].apply(ast.literal_eval)
train['location'] = train['location'].apply(ast.literal_eval)
features = pd.read_csv('features.csv')
train = train.merge(features, on=['feature_num', 'case_num'], how='left')
train = train.merge(patient_notes, on=['pn_num', 'case_num'], how='left')
merge以后数据张这样子, feature text是疾病名称,pn history就是病例annotation就是对应的体现这种疾病的具体描述。
根据label 生成 tensor,结合代码,我们看一下label 数据是怎么生成的
举个例子(不一定对应真实数据,仅作为说明使用):
PN:17-year-old male, has come to the student health clinic complaining of heart pounding. Mr. Cleveland's mother has given verbal consent for a history, physical examination, and treatment
-began 2-3 months ago,sudden,intermittent for 2 days(lasting 3-4 min),worsening,non-allev/aggrav
-associated with dispnea on exersion and rest,stressed out about school
-reports fe feels like his heart is jumping out of his chest
-ros:denies chest pain,dyaphoresis,wt loss,chills,fever,nausea,vomiting,pedal edeam
-pmh:non,meds :aderol (from a friend),nkda
-fh:father had MI recently,mother has thyroid dz
-sh:non-smoker,mariguana 5-6 months ago,3 beers on the weekend, basketball at school
-sh:no std
feature:'Family-history-of-MI-OR-Family-history-of-myocardial-infarction'
annotation: father had MI recently
我们需要做的就是把 pn 中 tokenized 以后原始father had MI recently 这个标记位置对应
的token位置标记为1,其他位置标记为0,cls,sep pading位置标记为-1
def create_label(cfg, text, annotation_length, location_list):
encoded = cfg.tokenizer(text,
add_special_tokens=True,
max_length=CFG.max_len,
padding="max_length",
return_offsets_mapping=True)
offset_mapping = encoded['offset_mapping']
ignore_idxes = np.where(np.array(encoded.sequence_ids()) != 0)[0]
label = np.zeros(len(offset_mapping))
label[ignore_idxes] = -1
if annotation_length != 0:
for location in location_list:
for loc in [s.split() for s in location.split(';')]:
start_idx = -1
end_idx = -1
start, end = int(loc[0]), int(loc[1])
for idx in range(len(offset_mapping)):
if (start_idx == -1) & (start < offset_mapping[idx][0]):
start_idx = idx - 1
if (end_idx == -1) & (end <= offset_mapping[idx][1]):
end_idx = idx + 1
if start_idx == -1:
start_idx = end_idx
if (start_idx != -1) & (end_idx != -1):
label[start_idx:end_idx] = 1
return torch.tensor(label, dtype=torch.float)
6、Baseline流程
1、加载数据,切分CV,定义dataloader
from transformers.models.deberta_v2 import DebertaV2TokenizerFast
tokenizer = DebertaV2TokenizerFast.from_pretrained(CFG.model)
#tokenizer = AutoTokenizer.from_pretrained(CFG.model)
CFG.tokenizer = tokenizer
# ====================================================
# Define max_len
# ====================================================
for text_col in ['pn_history']:
pn_history_lengths = []
tk0 = tqdm(patient_notes[text_col].fillna("").values, total=len(patient_notes))
for text in tk0:
length = len(tokenizer(text, add_special_tokens=False)['input_ids'])
pn_history_lengths.append(length)
LOGGER.info(f'{text_col} max(lengths): {max(pn_history_lengths)}')
for text_col in ['feature_text']:
features_lengths = []
tk0 = tqdm(features[text_col].fillna("").values, total=len(features))
for text in tk0:
length = len(tokenizer(text, add_special_tokens=False)['input_ids'])
features_lengths.append(length)
LOGGER.info(f'{text_col} max(lengths): {max(features_lengths)}')
CFG.max_len = max(pn_history_lengths) + max(features_lengths) + 3 # cls & sep & sep
LOGGER.info(f"max_len: {CFG.max_len}")
# ====================================================
# Dataset
# ====================================================
def prepare_input(cfg, text, feature_text):
inputs = cfg.tokenizer(text, feature_text,
add_special_tokens=True,
max_length=CFG.max_len,
padding="max_length",
return_offsets_mapping=False)
for k, v in inputs.items():
inputs[k] = torch.tensor(v, dtype=torch.long)
return inputs
def create_label(cfg, text, annotation_length, location_list):
encoded = cfg.tokenizer(text,
add_special_tokens=True,
max_length=CFG.max_len,
padding="max_length",
return_offsets_mapping=True)
offset_mapping = encoded['offset_mapping']
ignore_idxes = np.where(np.array(encoded.sequence_ids()) != 0)[0]
label = np.zeros(len(offset_mapping))
label[ignore_idxes] = -1
if annotation_length != 0:
for location in location_list:
for loc in [s.split() for s in location.split(';')]:
start_idx = -1
end_idx = -1
start, end = int(loc[0]), int(loc[1])
for idx in range(len(offset_mapping)):
if (start_idx == -1) & (start < offset_mapping[idx][0]):
start_idx = idx - 1
if (end_idx == -1) & (end <= offset_mapping[idx][1]):
end_idx = idx + 1
if start_idx == -1:
start_idx = end_idx
if (start_idx != -1) & (end_idx != -1):
label[start_idx:end_idx] = 1
return torch.tensor(label, dtype=torch.float)
class TrainDataset(Dataset):
def __init__(self, cfg, df):
self.cfg = cfg
self.feature_texts = df['feature_text'].values
self.pn_historys = df['pn_history'].values
self.annotation_lengths = df['annotation_length'].values
self.locations = df['location'].values
def __len__(self):
return len(self.feature_texts)
def __getitem__(self, item):
inputs = prepare_input(self.cfg,
self.pn_historys[item],
self.feature_texts[item])
label = create_label(self.cfg,
self.pn_historys[item],
self.annotation_lengths[item],
self.locations[item])
return inputs, label
2、定义模型
# ====================================================
# Model
# ====================================================
class CustomModel(nn.Module):
def __init__(self, cfg, config_path=None, pretrained=False):
super().__init__()
self.cfg = cfg
if config_path is None:
self.config = AutoConfig.from_pretrained(cfg.model, output_hidden_states=True)
else:
self.config = torch.load(config_path)
if pretrained:
self.model = AutoModel.from_pretrained(cfg.model, config=self.config)
else:
self.model = AutoModel(self.config)
self.fc_dropout_0 = nn.Dropout(0.1)
self.fc_dropout_1 = nn.Dropout(cfg.fc_dropout)
self.fc_dropout_2 = nn.Dropout(0.3)
self.fc_dropout_3 = nn.Dropout(0.4)
self.fc_dropout_4 = nn.Dropout(0.5)
self.fc = nn.Linear(self.config.hidden_size, 1)
self._init_weights(self.fc)
def _init_weights(self, module):
if isinstance(module, nn.Linear):
module.weight.data.normal_(mean=0.0, std=self.config.initializer_range)
if module.bias is not None:
module.bias.data.zero_()
elif isinstance(module, nn.Embedding):
module.weight.data.normal_(mean=0.0, std=self.config.initializer_range)
if module.padding_idx is not None:
module.weight.data[module.padding_idx].zero_()
elif isinstance(module, nn.LayerNorm):
module.bias.data.zero_()
module.weight.data.fill_(1.0)
def feature(self, inputs):
outputs = self.model(**inputs)
last_hidden_states = outputs[0]
return last_hidden_states
def forward(self, inputs):
feature = self.feature(inputs)
#output_0 = self.fc(self.fc_dropout_0(feature))
output_1 = self.fc(self.fc_dropout_1(feature))
#output_2 = self.fc(self.fc_dropout_2(feature))
#output_3 = self.fc(self.fc_dropout_3(feature))
#output_4 = self.fc(self.fc_dropout_4(feature))
output = output_1 #(output_0 + output_1 + output_2 + output_3 + output_4) / 5
return output
3、定义训练函数
# ====================================================
# Helper functions
# ====================================================
class AverageMeter(object):
"""Computes and stores the average and current value"""
def __init__(self):
self.reset()
def reset(self):
self.val = 0
self.avg = 0
self.sum = 0
self.count = 0
def update(self, val, n=1):
self.val = val
self.sum += val * n
self.count += n
self.avg = self.sum / self.count
def asMinutes(s):
m = math.floor(s / 60)
s -= m * 60
return '%dm %ds' % (m, s)
def timeSince(since, percent):
now = time.time()
s = now - since
es = s / (percent)
rs = es - s
return '%s (remain %s)' % (asMinutes(s), asMinutes(rs))
def train_fn(fold, train_loader, model, criterion, optimizer, epoch, scheduler, device):
model.train()
scaler = torch.cuda.amp.GradScaler(enabled=CFG.apex)
losses = AverageMeter()
start = end = time.time()
global_step = 0
for step, (inputs, labels) in enumerate(train_loader):
for k, v in inputs.items():
inputs[k] = v.to(device)
labels = labels.to(device)
batch_size = labels.size(0)
y_preds = model(inputs)
loss = criterion(y_preds.view(-1, 1), labels.view(-1, 1))
loss = torch.masked_select(loss, labels.view(-1, 1) != -1).mean()
if CFG.gradient_accumulation_steps > 1:
loss = loss / CFG.gradient_accumulation_steps
losses.update(loss.item(), batch_size)
loss.backward()
#grad_norm = torch.nn.utils.clip_grad_norm_(model.parameters(), CFG.max_grad_norm)
if (step + 1) % CFG.gradient_accumulation_steps == 0:
optimizer.step()
optimizer.zero_grad()
global_step += 1
if CFG.batch_scheduler:
scheduler.step()
end = time.time()
if step % CFG.print_freq == 0 or step == (len(train_loader)-1):
print('Epoch: [{0}][{1}/{2}] '
'Elapsed {remain:s} '
'Loss: {loss.val:.4f}({loss.avg:.4f}) '
'LR: {lr:.8f} '
.format(epoch+1, step, len(train_loader),
remain=timeSince(start, float(step+1)/len(train_loader)),
loss=losses,
lr=scheduler.get_lr()[0]))
return losses.avg
def train_fn_adv(fold, train_loader, model, criterion, optimizer, epoch, scheduler, device):
model.train()
scaler = torch.cuda.amp.GradScaler(enabled=CFG.apex)
losses = AverageMeter()
start = end = time.time()
global_step = 0
fgm = FGM(model)
for step, (inputs, labels) in enumerate(train_loader):
for k, v in inputs.items():
inputs[k] = v.to(device)
labels = labels.to(device)
batch_size = labels.size(0)
y_preds = model(inputs)
loss = criterion(y_preds.view(-1, 1), labels.view(-1, 1))
loss = torch.masked_select(loss, labels.view(-1, 1) != -1).mean()
if CFG.gradient_accumulation_steps > 1:
loss = loss / CFG.gradient_accumulation_steps
losses.update(loss.item(), batch_size)
loss.backward()
grad_norm = torch.nn.utils.clip_grad_norm_(model.parameters(), CFG.max_grad_norm)
if (step + 1) % CFG.gradient_accumulation_steps == 0:
fgm.attack()
# embedding参数被修改,此时,输入序列得到的embedding表征不一样
y_preds_adv = model(inputs)
loss_adv = criterion(y_preds_adv.view(-1, 1), labels.view(-1, 1))
loss_adv = torch.masked_select(loss_adv, labels.view(-1, 1) != -1).mean()
# 反向传播,并在正常的grad基础上,累加对抗训练的梯度
loss_adv.backward()
# 恢复embedding参数
fgm.restore()
optimizer.step()
optimizer.zero_grad()
global_step += 1
if CFG.batch_scheduler:
scheduler.step()
end = time.time()
if step % CFG.print_freq == 0 or step == (len(train_loader)-1):
print('Epoch: [{0}][{1}/{2}] '
'Elapsed {remain:s} '
'Loss: {loss.val:.4f}({loss.avg:.4f}) '
'LR: {lr:.8f} '
.format(epoch+1, step, len(train_loader),
remain=timeSince(start, float(step+1)/len(train_loader)),
loss=losses,
lr=scheduler.get_lr()[0]))
return losses.avg
# ====================================================
# train loop
# ====================================================
def train_loop(folds, fold):
LOGGER.info(f"========== fold: {fold} training ==========")
# ====================================================
# loader
# ====================================================
train_folds = folds[folds['fold'] != fold].reset_index(drop=True)
valid_folds = folds[folds['fold'] == fold].reset_index(drop=True)
valid_texts = valid_folds['pn_history'].values
valid_labels = create_labels_for_scoring(valid_folds)
train_dataset = TrainDataset(CFG, train_folds)
valid_dataset = TrainDataset(CFG, valid_folds)
train_loader = DataLoader(train_dataset,
batch_size=CFG.batch_size,
shuffle=True,
num_workers=CFG.num_workers, pin_memory=True, drop_last=True)
valid_loader = DataLoader(valid_dataset,
batch_size=CFG.batch_size,
shuffle=False,
num_workers=CFG.num_workers, pin_memory=True, drop_last=False)
# calculate warm up steps
CFG.num_warmup_steps = int(CFG.num_warmup_steps * len(train_dataset) / CFG.batch_size * CFG.epochs)
# ====================================================
# model & optimizer
# ====================================================
model = CustomModel(CFG, config_path=None, pretrained=True)
torch.save(model.config, OUTPUT_DIR+'config.pth')
model.to(device)
def get_optimizer_params(model, encoder_lr, decoder_lr, weight_decay=0.0):
param_optimizer = list(model.named_parameters())
no_decay = ["bias", "LayerNorm.bias", "LayerNorm.weight"]
optimizer_parameters = [
{'params': [p for n, p in model.model.named_parameters() if not any(nd in n for nd in no_decay)],
'lr': encoder_lr, 'weight_decay': weight_decay},
{'params': [p for n, p in model.model.named_parameters() if any(nd in n for nd in no_decay)],
'lr': encoder_lr, 'weight_decay': 0.0},
{'params': [p for n, p in model.named_parameters() if "model" not in n],
'lr': decoder_lr, 'weight_decay': 0.0}
]
return optimizer_parameters
optimizer_parameters = get_optimizer_params(model,
encoder_lr=CFG.encoder_lr,
decoder_lr=CFG.decoder_lr,
weight_decay=CFG.weight_decay)
optimizer = AdamW(optimizer_parameters, lr=CFG.encoder_lr, eps=CFG.eps, betas=CFG.betas)
# ====================================================
# scheduler
# ====================================================
def get_scheduler(cfg, optimizer, num_train_steps):
if cfg.scheduler=='linear':
scheduler = get_linear_schedule_with_warmup(
optimizer, num_warmup_steps=cfg.num_warmup_steps, num_training_steps=num_train_steps
)
elif cfg.scheduler=='cosine':
scheduler = get_cosine_schedule_with_warmup(
optimizer, num_warmup_steps=cfg.num_warmup_steps, num_training_steps=num_train_steps, num_cycles=cfg.num_cycles
)
return scheduler
num_train_steps = int(len(train_folds) / CFG.batch_size * CFG.epochs)
scheduler = get_scheduler(CFG, optimizer, num_train_steps)
# ====================================================
# loop
# ====================================================
criterion = nn.BCEWithLogitsLoss(reduction="none")
best_score = 0.
for epoch in range(CFG.epochs):
start_time = time.time()
# train
avg_loss = train_fn(fold, train_loader, model, criterion, optimizer, epoch, scheduler, device)
# eval
avg_val_loss, predictions = valid_fn(valid_loader, model, criterion, device)
predictions = predictions.reshape((len(valid_folds), CFG.max_len))
# scoring
char_probs = get_char_probs(valid_texts, predictions, CFG.tokenizer)
results = get_results(char_probs, th=0.5)
preds = get_predictions(results)
score = get_score(valid_labels, preds)
elapsed = time.time() - start_time
LOGGER.info(f'Epoch {epoch+1} - avg_train_loss: {avg_loss:.4f} avg_val_loss: {avg_val_loss:.4f} time: {elapsed:.0f}s')
LOGGER.info(f'Epoch {epoch+1} - Score: {score:.4f}')
if best_score < score:
best_score = score
LOGGER.info(f'Epoch {epoch+1} - Save Best Score: {best_score:.4f} Model')
torch.save({'model': model.state_dict(),
'predictions': predictions},
OUTPUT_DIR+f"{CFG.model.replace('/', '-')}_fold{fold}_best.pth")
predictions = torch.load(OUTPUT_DIR+f"{CFG.model.replace('/', '-')}_fold{fold}_best.pth",
map_location=torch.device('cpu'))['predictions']
valid_folds[[i for i in range(CFG.max_len)]] = predictions
torch.cuda.empty_cache()
gc.collect()
del scheduler
del optimizer
del model
return valid_folds
4、定义eval函数
def valid_fn(valid_loader, model, criterion, device):
losses = AverageMeter()
model.eval()
preds = []
start = end = time.time()
for step, (inputs, labels) in enumerate(valid_loader):
for k, v in inputs.items():
inputs[k] = v.to(device)
labels = labels.to(device)
batch_size = labels.size(0)
with torch.no_grad():
y_preds = model(inputs)
loss = criterion(y_preds.view(-1, 1), labels.view(-1, 1))
loss = torch.masked_select(loss, labels.view(-1, 1) != -1).mean()
if CFG.gradient_accumulation_steps > 1:
loss = loss / CFG.gradient_accumulation_steps
losses.update(loss.item(), batch_size)
preds.append(y_preds.sigmoid().to('cpu').numpy())
end = time.time()
if step % CFG.print_freq == 0 or step == (len(valid_loader)-1):
print('EVAL: [{0}/{1}] '
'Elapsed {remain:s} '
'Loss: {loss.val:.4f}({loss.avg:.4f}) '
.format(step, len(valid_loader),
loss=losses,
remain=timeSince(start, float(step+1)/len(valid_loader))))
predictions = np.concatenate(preds)
return losses.avg, predictions
def inference_fn(test_loader, model, device):
preds = []
model.eval()
model.to(device)
tk0 = tqdm(test_loader, total=len(test_loader))
for inputs in tk0:
for k, v in inputs.items():
inputs[k] = v.to(device)
with torch.no_grad():
y_preds = model(inputs)
preds.append(y_preds.sigmoid().to('cpu').numpy())
predictions = np.concatenate(preds)
return predictions
5、调参完成训练,上传权重提交成绩
# ====================================================
# CFG
# ====================================================
class CFG:
debug=False
apex=False
print_freq=100
num_workers=4
model="microsoft/deberta-v3-large"
scheduler='cosine' # ['linear', 'cosine']
batch_scheduler=True
num_cycles=0.5
num_warmup_steps=0.1
epochs=5
encoder_lr=2e-5
decoder_lr=2e-5
min_lr=1e-6
eps=1e-6
betas=(0.9, 0.999)
batch_size=8
fc_dropout=0.2
max_len=512
weight_decay=0.01
gradient_accumulation_steps=1
max_grad_norm=500
seed=42
n_fold=5
trn_fold=[4]
train=True
if CFG.debug:
CFG.epochs = 5
CFG.trn_fold = [0,1,2,3,4]
7、赛题难点思考
1、label中大量为0, 1很少label不平横对模型的影响
2、专业领域很多简称和没有在vocab中收录的词会不会对模型造成影响
8、无痛涨分Trick多drop out 对比学习
r_drop
进技术交流群请添加AINLP小助手微信(id: ainlper)
请备注具体方向+所用到的相关技术点
关于AINLP
AINLP 是一个有趣有AI的自然语言处理社区,专注于 AI、NLP、机器学习、深度学习、推荐算法等相关技术的分享,主题包括文本摘要、智能问答、聊天机器人、机器翻译、自动生成、知识图谱、预训练模型、推荐系统、计算广告、招聘信息、求职经验分享等,欢迎关注!加技术交流群请添加AINLPer(id:ainlper),备注工作/研究方向+加群目的。
阅读至此了,分享、点赞、在看三选一吧🙏
Recommend
About Joyk
Aggregate valuable and interesting links.
Joyk means Joy of geeK