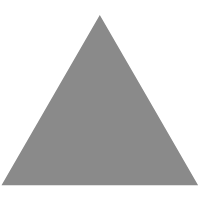
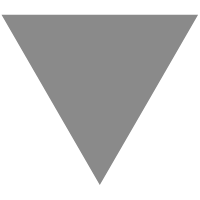
Tesla: The Autopilot 3D Update Will Be A Significant Stock Catalyst特斯拉:自动驾...
source link: https://www.apexyun.com/untitled-39/
Go to the source link to view the article. You can view the picture content, updated content and better typesetting reading experience. If the link is broken, please click the button below to view the snapshot at that time.
Tesla: The Autopilot 3D Update Will Be A Significant Stock Catalyst特斯拉:自动驾驶仪3D技术更新将成为重要的股票催化剂
Eady Capital
Summary
摘要
- Tesla's upcoming 3D update will bring a sudden increase in AI-assisted driving functionality.
- 特斯拉即将发布的3D更新将增加AI辅助驾驶功能。
- Analysts and investors who have failed to realize Tesla's competitive advantage in autonomous driving technology are in for an abrupt awakening.
- 未能意识到特斯拉在自动驾驶技术上的竞争优势的分析师和投资者,将会突然觉醒。
- Financial models mostly still treat Tesla like a conventional automaker but that will no longer be appropriate in the future. 3.大多数金融模型仍将特斯拉视为传统的汽车制造商,但这在未来将不再适用。
Analysts and investors systemically underrate Tesla's (TSLA) competitive position in autonomous driving. Assessments of the competitive landscape lean far too much on qualitative judgments of autonomous vehicle performance in demo videos, as well as other PR, marketing, and branding exercises. Sell-side analysts assigned to Tesla have, historically, been mostly auto analysts without the time or obsessiveness to dig deeply into deep learning and robotics. This means Tesla's autonomy advantage is getting underpriced or simply going unpriced in the market.
分析师和投资者系统性地低估了特斯拉(Tesla)在自动驾驶领域的竞争地位。对竞争环境的评估过于依赖于对演示视频中自动驾驶汽车性能的定性判断,以及其他公关、营销和品牌塑造活动。从历史上看,被指派到特斯拉的卖方分析师大多是汽车分析师,这意味着他们没有时间或精力深度学习和研究机器人技术。这意味着特斯拉的自主优势正在被低估,或者根本无法在市场上定价。
In the three years I've been writing about Tesla, I've been banging the table, insisting that scale of data matters above almost all else. I've also argued again and again that it is a mistake to assume that Tesla's progress on autonomy will be smooth and continuous, rather than lumpy and punctuated by fits, starts, and plateaus. I surmise that deep learning R&D has two phases. The data collection and labeling phase can be relatively rapid. The speed depends on the number of robots a company has in the wild and the number of workers it employs to label data.
在我撰写关于特斯拉的文章的三年中,我一直坚持认为数据规模比几乎所有其他事情都重要。我也一次又一次地指出,认为特斯拉在自动驾驶方面的进展将是平稳而持续的,而不是磕磕绊绊、断断续续的。我推测,深度学习研发有两个阶段。数据收集和标记阶段可能相对快速。速度取决于公司在野外拥有的机器人数量以及它雇佣的数据标签工人数量。
Then, there is the plodding, unpredictable phase wherein AI scientists and engineers endeavor to build a system that ingests labelled data and outputs useful robot behaviors, such as steering, accelerating, and braking at the right times, in the right magnitudes. Getting data is like filling up a gas tank. The AI scientists' and engineers' job is like building an engine. From the odometer's perspective, progress can be nothing, nothing, nothing, and then, in an instant, the ignition switch is turned and the car zooms off.
接下来是一个缓慢而不可预测的阶段,人工智能科学家和工程师致力于构建一个系统,以适当的时间、合适的力度摄取标签数据并输出有用的机器人行为,比如转向、加速和刹车。获取数据就像给油箱加油。AI科学家和工程师的工作就像制造引擎一样。从里程表的角度来看,进展可能是什么都没有,然后,在一瞬间,点火开关被打开,汽车就启动了。
One remarkable example of non-linear progress in AI is OpenAI's breakthrough on the classic video game Montezuma's Revenge. This graph tells it all:
AI非线性发展的一个显著例子就是OpenAI在经典视频游戏Montezuma's Revenge上的突破。这张图表说明了一切:
In this case, data was collected from the game and labelled automatically, so the dataset creation phase was much faster than the science and engineering phase. Moreover, overall progress on Montezuma's Revenge in the AI community was the opposite of smooth and continuous.
在这种情况下,数据是从游戏中收集并自动标记,因此数据集创建阶段要比科学和工程阶段快得多。此外,蒙特祖玛(Montezuma)的复仇在AI社区的整体进展也不是顺利和持续的。
Tesla has over 900,000 robots on the road. All its competitors combined have less than 2,000 robot vehicles in the United States and, given that most testing occurs in the U.S., the global total is likely not much higher. The performance of deep neural networks scales predictably with data, such that a data advantage of this magnitude could yield anywhere from a 2x to 30x performance advantage.
特斯拉在路上有超过90万个机器人。在美国,其所有竞争对手的合计机器人数量不到2000辆,而且鉴于大多数测试都在美国进行,因此全球总数可能不会高很多。深度神经网络的性能可预测地随着数据的变化而变化,这样的数据优势可以产生2倍到30倍的性能优势。
A major caveat is that data needs to be labelled either manually or automatically. As I've written about extensively, Tesla has many promising options for automatically labeling data and for using its vast fleet of cars to make manual data labeling far more efficient. (That is, to get far more neural network performance out of the same amount of human labor.) The challenge for Tesla's AI scientists and engineers is to pursue those options and make them work as well at commercial scale as they do in academic proofs of concept. In other words, to build an engine that can run on the ample fuel available.
一个重要的警告是,数据需要手动或自动地标记。正如我所写的那样,特斯拉有许多很有前途的选择来自动标记数据,并使用其庞大的车队来使手动数据标记更加高效。(也就是说,在同样的人力资源中获得更多的神经网络性能。)特斯拉的AI科学家和工程师面临的挑战是,寻求这些选择,并让它们在商业规模上像在概念的学术证明中那样发挥作用。换句话说,就是制造一个能使用充足的燃料运行的引擎。
Going 3D
走向3D
Apparently, the Tesla AI team's most significant work right now is shifting from a 2D paradigm in computer vision to a 3D paradigm. Elon Musk first described the concept on the Third Row Tesla podcast:
显然,特斯拉AI团队目前最重要的工作是将计算机视觉从2D范式转换为3D范式。埃隆·马斯克(Elon Musk)在特斯拉第三行播客中首次描述了这一概念:
https://youtu.be/oW-cENkT8ws(视频可省)
Musk recently elaborated on the work involved in making this shift:
马斯克最近详细阐述了这一转变所涉及的工作:
Twitter:
“Whole Mars
@WholeMarsBlog
·
Jul 2, 2020
Replying to @elonmusk @romanhistory1 and @HardcoreHistory
how’s the autopilot rewrite going?
you guys calling it PlaidNet?
Elon Musk
@elonmusk
Going well. Team is kicking ass & it’s an honor to work with them. Pretty much everything had to be rewritten, including our labeling software, so that it’s fundamentally “3D” at every step from training through inference.
4:54 AM · Jul 2, 2020”
“Whole Mars
@WholeMarsBlog
2020年7月2日
回复@elonmusk @romanhistory1和@HardcoreHistory
自动驾驶仪的重写怎么样了?
你们叫它 PlaidNet么?
Elon Musk
@elonmusk
顺利。团队很出色,和他们一起工作是一种荣耀。几乎所有的东西都需要重写,包括我们的标签软件,所以从训练到推理的每一步都是“3D”的。
2020年7月2日凌晨4:54”
“Tesla Owners East Bay
@TeslaOwnersEBay
·
Jul 2, 2020
Replying to @elonmusk @WholeMarsBlog and 2 others
Any updates on reverse summon?
Elon Musk
@elonmusk
A lot of functionality will happen all at once when we transition to the new software stack. Most likely, it will be releasable in 2 to 4 months. Then it’s a question of what functionality is proven safe enough to enable for owners.
5:01 AM · Jul 2, 2020
1.4K
277 people are Tweeting about this”
Tesla Owners East Bay
@TeslaOwnersEBay
·
2020年7月2日
回复了@elonmusk @WholeMarsBlog和其他两个博客
反向召唤有什么更新吗?
Elon Musk
@elonmusk
当我们转换到新的软件栈时,许多功能将同时发生。最有可能的是,它将在2到4个月内发布。接下来的问题是,什么样的功能被证明足够安全,可以让用户使用。
2020年7月2日上午5:01
1.4 k
277人在推特上讨论这个问题"
——————来自推特内容
What does it mean to transition from 2D to fundamentally 3D? As best as I can surmise, it's about how sensor data is represented to neural networks.
从2D到3D的转变意味着什么?就我所能猜测到的来说,这与传感器数据表示为神经网络有关。
LiDAR laser pulses create a 3D representation of surrounding objects by creating a point whenever the light hits an object and returns to the sensor. This 3D representation of the world is known as a point cloud. It looks like this:
LiDAR激光脉冲通过在光线撞击物体并返回传感器时创建一个点来创建周围物体的3D表示。世界的3D表示称为点云。
https://youtu.be/XylvhH3NPiI(视频可省)
In 2018, computer vision researchers from Cornell University (including Yan Wang and Wei-Lun Chao) published a pre-print showing that cameras can be used to create 3D point clouds and that, more importantly, using this form of representation rather than 2D images improves neural networks' ability to estimate depth using cameras. Cameras can derive points through stereo vision, which is also how humans and some other mammals perceive depth at certain distances. It turns out what makes LiDAR so effective is not just the lasers but also the common practice of parsing LiDAR input as point clouds. Wang et al. called the approach of using cameras to generate point clouds "pseudo-LiDAR ". Since 2018, other researchers have built on this work.
2018年,来自康奈尔大学的计算机视觉研究人员(包括王燕和赵卫麟)发表了一份预印本,显示可以使用相机来创建3D点云,更重要的是,使用这种形式的表示方式不是2D图像提高了神经网络使用相机预测深度的能力。相机可以通过立体视觉获得点,这也是人类和其他哺乳动物在一定距离下感知深度的方式。事实证明,使LiDAR(激光雷达)如此有效的不仅仅在于激光器,还有将LiDAR(激光雷达)输入解析为点云的常见做法。Wang等人将利用相机生成点云的方法称为“伪LiDAR(激光雷达)”。自2018年以来,其他研究人员也在此基础上开展了研究。
Today, pseudo-LiDAR and associated approaches like pseudo-LiDAR++ and ViDAR (which stands for visual LiDAR or video LiDAR) are within spitting distance of matching LiDAR on 3D object detection and depth estimation, at least on certain academic benchmarks. In my understanding, pseudo-LiDAR++ is about one-third as accurate as LiDAR on the popular KITTI Vision benchmark.
如今,至少在某些学术基准上,伪LiDAR及其相关方法(如伪LiDAR ++和ViDAR(代表可视LiDAR或视频LiDAR))在3D对象检测和深度估计上与匹配LiDAR非常接近。据我了解,伪LiDAR ++的准确度大约是流行的KITTI Vision基准上LiDAR的三分之一。
Earlier this year, Tesla's Senior Director of AI, Andrej Karpathy, publicly disclosed that Tesla is working on a pseudo-LiDAR approach to depth estimation. Going back to Autonomy Day in 2019, Karpathy gave a demonstration of the 3D depth information that be obtained via stereo vision:
今年早些时候,特斯拉的AI高级总监安德烈·卡帕西(Andrej Karpathy)公开透露,特斯拉目前正在研究一种用于深度估算的伪LiDAR方法。回到2019年的自治日(Autonomy Day ),卡帕西演示了通过立体视觉获得的3D深度信息:
https://youtu.be/LR0bDLCElKg(视频)
In his most recent talks, Karpathy has shared a glimpse of the accuracy gains from 3D representations vs. 2D representations. These visualizations show curb detection, with the "ground truth" (presumably from LiDAR) on the left, camera-based detection using 2D representations on the right, and camera-based detection using 3D representations in the middle:
在他最近的演讲中,Karpathy分享了3D表示与2D表示中获得的准确性的一小部分。这些可视化显示了路缘检测,左侧是“ground truth”(可能来自LiDAR),右侧是使用2D表示的基于摄像头的检测,中间是使用3D表示的基于摄像头的检测:
If this one qualitative result is truly representative of Tesla's performance gains across the board, then the 3D update will surely bring a vast improvement to Tesla's AI-assisted driving software. From an outside perspective, this improvement will appear sudden and discontinuous.
如果这一定性的结果真正代表了特斯拉的整体性能提升,那么3D更新必将给特斯拉的AI辅助驾驶软件带来巨大的改进。从外部来看,这种改善会出现突发性和断续性。
Here comes the money
钱来了
When it comes to Tesla and autonomy, most analysts and investors take an "I'll believe it when I see it" approach. That's their prerogative, but, in my view, it means underestimating Tesla's earnings and cash flow in 2021 and beyond. Before full autonomy is within reach, Tesla will continue to press forward with heavily AI-assisted driving. Already, Teslas can automatically stop at traffic lights and stop signs:
谈到特斯拉和自治(autonomy)方面,大多数分析师和投资者采取的是“我看到就相信”的态度。当然,这是他们的权利,但在我看来,这意味着低估了特斯拉在2021年及以后的收益和现金流。在实现完全的自动驾驶之前,特斯拉将继续大力推进AI辅助驾驶。特斯拉已经可以在红绿灯和停车标志前自动停车:
https://youtu.be/BcY8iCQf924(视频可省)
AI-assisted driving is arguably Tesla drivers' favorite feature and the most obvious differentiator between Teslas and other vehicles. Competing automakers have been incredibly slow to implement something as basic as wireless software updates and none, as far as I'm aware, have publicly announced plans to build a deep learning pipeline around its production cars like Tesla has done. In 2021 and beyond, I believe differentiated software will create even more demand for Tesla's vehicles.
AI辅助驾驶可以说是特斯拉使用者最喜欢的功能,也是特斯拉和其他汽车之间最明显的区别。与之竞争的汽车制造商在实施无线软件更新这样的基础技术方面进展缓慢,据我所知,还没有一家公司像特斯拉那样,公开宣布计划围绕其汽车生产建立一个深度学习渠道。我相信,2021年以后,差异化软件将为特斯拉汽车带来更多需求。
For a Model 3 Standard Range Plus with no other add-ons, the $8,000 "Full Self-Driving Capability" option is 17% of the purchase price. That is high-margin revenue since the marginal cost of downloading software is negligible. Musk has repeatedly said that the price will increase as more functionality is added. Moreover, Tesla has plans to sell the software as a monthly subscription. This will surely expand the customer base.
对于没有其他附加组件的Model 3 Standard Range Plus,售价8,000美元的“完全自动驾驶功能”选项为购买价格的17%。这是一笔高利润收入,因为下载软件的边际成本可以忽略不计。马斯克多次表示,随着功能的增加,价格将会上涨。此外,特斯拉计划以每月订购的形式销售该软件。这必将扩大客户基础。
Growing revenue from AI-assisted driving software means analysts should think about Tesla's prospective margins beyond an apples-to-apples comparison to industry peers. Moreover, Tesla's software advantage warrants bullish growth assumptions.
AI辅助驾驶软件带来的收入不断增长,意味着分析师们应该考虑特斯拉的预期利润率,而不是仅与其业内同行进行比较。此外,特斯拉在软件方面的优势证明了乐观的增长假设。
Adam Jonas from Morgan Stanley (MS) recently published a $2,070 bull case for Tesla based on a forecast of 6 million vehicle sales in 2030. In my view, this level of sales volume for 2030 makes sense, given that Tesla is stepping into the gray area between being a car company and being an AI and robotics company. It isn't clear to me how any competitor is going to merge auto manufacturing competency with software and AI competency fast enough to slow down Tesla's current trajectory of hypergrowth. As such, even at roughly $1,400, I still consider the stock to have a significant upside.
摩根士丹利(Morgan Stanley)的亚当·乔纳斯(Adam Jonas)最近发布了特斯拉2030年汽车销量为600万辆的看涨预测,认为特斯拉的股价将上涨2070美元。在我看来,考虑到特斯拉正步入汽车公司与人工智能(AI)和机器人公司之间的灰色地带,这种2030年的销量水平是合理的。目前尚不清楚,竞争对手如何能将汽车制造能力、软件和人工智能能力迅速合并,以减缓特斯拉目前的高速增长轨迹。因此,即使股价在1400美元左右,我仍然认为该股仍有很大的上涨空间。
We also might think beyond vehicle sales. After the 3D update rolls out to customers, I predict that more analysts and investors will start thinking seriously about robotaxis. Given the uncertainty, it's difficult to know how to price the robotaxi opportunity. However, private market investors have managed to do this with Waymo (GOOG, GOOGL) and Cruise (GM). Why can't the public market do it with Tesla?
我们还可以考虑汽车销售以外的问题。我预计,在“3D更新”向客户推出后,会有更多的分析师和投资者开始认真考虑机器人(robotaxis)出租车。虽然鉴于这种不确定性,很难知道如何为机器人出租车(robotaxis)的机会定价。然而,私人市场投资者已经通过Waymo (GOOG, GOOGL)和Cruise (GM)成功做到了这一点。为什么特斯拉无法在公众市场上做到这一点呢?
Disclosure: I am/we are long TSLA. I wrote this article myself, and it expresses my own opinions. I am not receiving compensation for it (other than from Seeking Alpha). I have no business relationship with any company whose stock is mentioned in this article.
披露:我/我们是long TSLA。这篇文章是我自己写的,表达了我自己的观点。我没有因此得到补偿(除了寻求阿尔法)。我和本文中提到的任何公司都没有业务关系。
Recommend
About Joyk
Aggregate valuable and interesting links.
Joyk means Joy of geeK