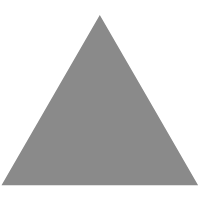
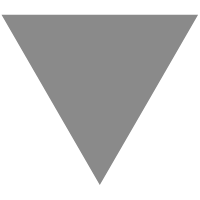
深度学习需要掌握的 13 个概率分布(附代码)
source link: http://mp.weixin.qq.com/s?__biz=MzIyNjM2MzQyNg%3D%3D&%3Bmid=2247533622&%3Bidx=2&%3Bsn=dd200ee1582a0277771da8bc8be9577b
Go to the source link to view the article. You can view the picture content, updated content and better typesetting reading experience. If the link is broken, please click the button below to view the snapshot at that time.
↑↑↑关注后" 星标 "Datawhale
每日干货 & 每月组队学习 ,不错过
Datawhale干货
知乎作者:Sophia 来源:深度学习自然语言处理
本文仅用于学术分享,著作权归作者所有。如有侵权,请联系后台作删文处理。
在逛Github时发现了一个不错的总结,对深度学习的概率分布进行了总结。
作者的Github开源地址:
https://github.com/graykode/distribution-is-all-you-needgithub.com

1. 均匀分布(连续)代码:
https://github.com/graykode/distribution-is-all-you-need/blob/master/uniform.py
均匀分布在 [a,b] 上具有相同的概率值,是简单概率分布。
2. 伯努利分布(离散)代码:
https://github.com/graykode/distribution-is-all-you-need/blob/master/bernoulli.py
先验概率 p(x)不考虑伯努利分布。因此,如果我们对最大似然进行优化,那么我们很容易被过度拟合。
利用二元交叉熵对二项分类进行分类。它的形式与伯努利分布的负对数相同。
3. 二项分布(离散)代码:
https://github.com/graykode/distribution-is-all-you-need/blob/master/binomial.py
参数为 n 和 p 的二项分布是一系列 n 个独立实验中成功次数的离散概率分布。
二项式分布是指通过指定要提前挑选的数量而考虑先验概率的分布。
4. 多伯努利分布/分类分布(离散)代码:
https://github.com/graykode/distribution-is-all-you-need/blob/master/categorical.py
多伯努利称为分类分布。 交叉熵和采取负对数的多伯努利分布具有相同的形式。
5. 多项式分布(离散)代码:
https://github.com/graykode/distribution-is-all-you-need/blob/master/multinomial.py
多项式分布与分类分布的关系与伯努尔分布与二项分布的关系相同。
6. β分布(连续)代码:
https://github.com/graykode/distribution-is-all-you-need/blob/master/beta.py
β分布与二项分布和伯努利分布共轭。
利用共轭,利用已知的先验分布可以更容易地得到后验分布。
当β分布满足特殊情况(α=1,β=1)时,均匀分布是相同的。
7. Dirichlet 分布(连续)代码:
https://github.com/graykode/distribution-is-all-you-need/blob/master/dirichlet.py
dirichlet 分布与多项式分布是共轭的。
如果 k=2,则为β分布。

8.伽马分布(连续)代码:
https://github.com/graykode/distribution-is-all-you-need/blob/master/gamma.py
如果 gamma(a,1)/gamma(a,1)+gamma(b,1)与 beta(a,b)相同,则 gamma 分布为β分布。
指数分布和卡方分布是伽马分布的特例。
9. 指数分布(连续)代码:
https://github.com/graykode/distribution-is-all-you-need/blob/master/exponential.py
指数分布是 α 为 1 时 γ 分布的特例。
10. 高斯分布(连续)代码:
https://github.com/graykode/distribution-is-all-you-need/blob/master/gaussian.py
高斯分布是一种非常常见的连续概率分布。
11. 正态分布(连续)代码:
https://github.com/graykode/distribution-is-all-you-need/blob/master/normal.py
正态分布为标准高斯分布,平均值为0,标准差为1。
12. 卡方分布(连续)代码:
https://github.com/graykode/distribution-is-all-you-need/blob/master/chi-squared.py
k 自由度的卡方分布是 k 个独立标准正态随机变量的平方和的分布。
卡方分布是 β 分布的特例。
13. t 分布(连续)代码:
https://github.com/graykode/distribution-is-all-you-need/blob/master/student-t.py
t分布是对称的钟形分布,与正态分布类似,但尾部较重,这意味着它更容易产生远低于平均值的值。
“整理不易, 点 赞 三连 ↓
Recommend
About Joyk
Aggregate valuable and interesting links.
Joyk means Joy of geeK