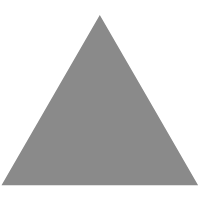
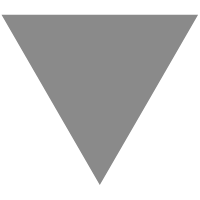
n维空间下两个随机向量的夹角分布
source link: https://kexue.fm/archives/7076
Go to the source link to view the article. You can view the picture content, updated content and better typesetting reading experience. If the link is broken, please click the button below to view the snapshot at that time.
昨天群里大家讨论到了$n$维向量的一些反直觉现象,其中一个话题是“一般$n$维空间下两个随机向量几乎都是垂直的”,这就跟二维/三维空间的认知有明显出入了。要从理论上认识这个结论,我们可以考虑两个随机向量的夹角$\theta$分布,并算算它的均值方差。
首先,我们来推导$\theta$的概率密度函数。呃,其实也不用怎么推导,它是$n$维超球坐标的一个直接结论。
要求两个随机向量之间的夹角分布,很显然,由于各向同性,所以我们只需要考虑单位向量,而同样是因为各向同性,我们只需要固定其中一个向量,考虑另一个向量随机变化。不是一般性,考虑随机向量为
\begin{equation}\boldsymbol{x}=(x_1,x_2,\dots,x_n)\end{equation}
而固定向量为
\begin{equation}\boldsymbol{y}=(1,0,\dots,0)\end{equation}
将$\boldsymbol{x}$变换为超球坐标(关于$n$维球的知识可以参考 维基百科 ):
\begin{equation}
\left\{\begin{aligned}
x_{1}&=\cos(\varphi_{1})\\
x_{2}&=\sin(\varphi_{1})\cos(\varphi_{2})\\
x_{3}&=\sin(\varphi_{1})\sin(\varphi_{2})\cos(\varphi_{3})\\
&\,\,\vdots \\
x_{n-1}&=\sin(\varphi_{1})\cdots \sin(\varphi_{n-2})\cos(\varphi_{n-1})\\
x_{n}&=\sin(\varphi_{1})\cdots \sin(\varphi_{n-2})\sin(\varphi_{n-1})
\end{aligned}\right.
\end{equation}
其中$\varphi_{n−1}\in [0, 2\pi)$而剩下的$\varphi$范围是$[0, \pi]$。此时,$\boldsymbol{x}$和$\boldsymbol{y}$的夹角是:
\begin{equation}\arccos \langle \boldsymbol{x},\boldsymbol{y}\rangle = \arccos \cos(\varphi_{1}) = \varphi_{1}
\end{equation}
也就是说两者的夹角正好是$\varphi_1$。那么,$\boldsymbol{x}$和$\boldsymbol{y}$的夹角不超过$\theta$的概率是:
\begin{equation}\begin{aligned}
P_n(\varphi_1\leq\theta) =& \frac{\int_0^{2\pi}\cdots\int_0^{\pi}\int_0^{\theta}\sin^{n-2}(\varphi_{1})\sin^{n-3}(\varphi_{2})\cdots \sin(\varphi_{n-2})\,d\varphi_{1}\,d\varphi_{2}\cdots d\varphi_{n-1}}{\int_0^{2\pi}\cdots\int_0^{\pi}\int_0^{\pi}\sin^{n-2}(\varphi_{1})\sin^{n-3}(\varphi_{2})\cdots \sin(\varphi_{n-2})\,d\varphi_{1}\,d\varphi_{2}\cdots d\varphi_{n-1}}\\
=&\frac{\text{(n-1)维单位超球的表面积}\times\int_0^{\theta}\sin^{n-2}\varphi_{1} d\varphi_1}{\text{n维单位超球的表面积}}\\
=&\frac{\Gamma(\frac{n}{2})}{\Gamma(\frac{n-1}{2})\sqrt{\pi}} \int_0^{\theta}\sin^{n-2}\varphi_1 d\varphi_1
\end{aligned}
\end{equation}
这表明$\theta$的概率密度函数就是
\begin{equation}
p_n(\theta) = \frac{\Gamma(\frac{n}{2})}{\Gamma(\frac{n-1}{2})\sqrt{\pi}}\sin^{n-2} \theta
\label{eq:theta}\end{equation}
有时候我们想关心$\eta=\cos\theta$的分布,这时候需要做一下概率密度的换元
\begin{equation}\begin{aligned}
p_n(\eta)=&\frac{\Gamma(\frac{n}{2})}{\Gamma(\frac{n-1}{2})\sqrt{\pi}}\sin^{n-2} (\arccos\eta)\left|\frac{d\theta}{d\eta}\right|\\
=&\frac{\Gamma(\frac{n}{2})}{\Gamma(\frac{n-1}{2})\sqrt{\pi}}(1-\eta^2)^{(n-3)/2}\\
\end{aligned}\label{eq:cos}\end{equation}
由$\eqref{eq:theta}$和$\eqref{eq:cos}$我们可以看到,当$n=2$时,夹角$\theta$的分布是一个均匀分布,而当$n=3$时,夹角余弦$\cos\theta$的分布是均匀分布。这两个结果说明在我们所能感知到的二维和三维空间中,角度的分布是比较均匀的。但是$n$比较大的时候呢?比如$n=50,100$?
从$p_n(\theta)\sim\sin^{n-2}\theta$的形式可以发现,当$n\geq 3$时,最大概率是$\theta=\frac{\pi}{2}$(即90度),另外$\sin^{n-2}\theta$也是关于$\theta=\frac{\pi}{2}$对称的,所以它的均值也是$\frac{\pi}{2}$。但这还不能充分描述分布情况,我们还需要考虑方差
\begin{equation}
Var_n(\theta) = \frac{\Gamma(\frac{n}{2})}{\Gamma(\frac{n-1}{2})\sqrt{\pi}}\int_0^{\pi}\left(\theta-\frac{\pi}{2}\right)^2\sin^{n-2} \theta d\theta\end{equation}
这个积分有解析解,但是形式很麻烦(喜欢看的话可以自己用Mathematica去算),我们来看部分数值解就好:
$$\begin{array}{c|c}
\hline
n & \text{方差}\\
\hline
3 & 0.467401\\
10 & 0.110661\\
20 & 0.0525832\\
50 & 0.0204053\\
100 & 0.0101007\\
200 & 0.00502508\\
1000 & 0.001001\\
\hline
\end{array}$$
可以看到,随着$n$的增大,方差越来越小,这意味着高维空间中任意两个向量的夹角几乎都集中在$\frac{\pi}{2}$附近,换言之,高维空间中任意两个向量几乎都是垂直的。
如果想要解析近似解的读者,可以考虑用拉普拉斯近似,考虑用一个高斯分布去近似$p_n(\theta)$,即在$\theta=\frac{\pi}{2}$处对$\ln \sin^{n-2}\theta$进行展开:
\begin{equation}\ln \sin^{n-2}\theta=\frac{2-n}{2}\left(x - \frac{\pi}{2}\right)^2 + \mathscr{O}\left(\left(x - \frac{\pi}{2}\right)^4\right)\end{equation}
即
\begin{equation}\sin^{n-2}\theta\approx exp\left[-\frac{n-2}{2}\left(x - \frac{\pi}{2}\right)^2\right]\end{equation}
从这个近似形式看,我们可以近似地认为$\theta$服从均值为$\frac{\pi}{2}$、方差为$\frac{1}{n-2}$的正态分布,即当$n$较大时,方差近似为$\frac{1}{n-2}$,这也能看出$n$越大,方差越小。
本文对高维空间的夹角分布进行了推导,记录在此以备忘,同时也供有需要的读者参考。
转载到请包括本文地址: https://kexue.fm/archives/7076
更详细的转载事宜请参考: 《科学空间FAQ》
如果您还有什么疑惑或建议,欢迎在下方评论区继续讨论。
如果您觉得本文还不错,欢迎/本文。打赏并非要从中获得收益,而是希望知道科学空间获得了多少读者的真心关注。当然,如果你无视它,也不会影响你的阅读。再次表示欢迎和感谢!
Recommend
About Joyk
Aggregate valuable and interesting links.
Joyk means Joy of geeK