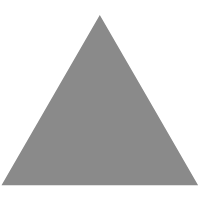
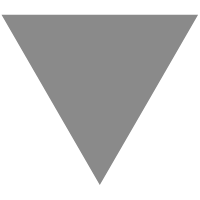
GitHub - wepe/efficient-decision-tree-notes: 高效决策树算法系列笔记
source link: https://github.com/wepe/efficient-decision-tree-notes
Go to the source link to view the article. You can view the picture content, updated content and better typesetting reading experience. If the link is broken, please click the button below to view the snapshot at that time.
README.md
efficient-decision-tree-notes
这个笔记记录高效的决策树系列算法,主要阅读论文,结合一些开源框架,希望在弄清算法的基础上,尝试着改进算法,尝试着工程实现.
我们知道,目前比较流行的两个GBDT开源框架是XGBoost和LightGBM,无论内存占用还是计算速度,它们都做到了淋漓尽致.在LightGBM的Feature上提到了,XGBoost的decision tree用的是pre-sorted based的算法,也就是在tree building之前对各维特征先排序,代表性的算法是SLIQ[1]和SPRINT[2].而LightGBM的decision tree是histogram based的算法,也就是先将特征离散化,代表性的算法是CLOUDS[3],Mcrank[4]和Machado[5].
SLIQ和SPRINT算法的特点决定了树生长的方式是level-wise(breadth-first)的,与之对应的是leaf-wise(depth-wise,best-wise[6])的方式,LightGBM正是采用leaf-wise的方式.
内容大致按以下几部分展开:
-
基于特征预排序的算法SLIQ
-
基于特征预排序的算法SPRINT
-
基于特征离散化的算法CLOUDS
-
研究开源框架: LightGBM,XGBoost
-
自己动手实现一个高效决策树 tgboost
参考文献
[1] Mehta, Manish, Rakesh Agrawal, and Jorma Rissanen. “SLIQ: A fast scalable classifier for data mining.” International Conference on Extending Database Technology. Springer Berlin Heidelberg, 1996.
[2] Shafer, John, Rakesh Agrawal, and Manish Mehta. “SPRINT: A scalable parallel classifier for data mining.” Proc. 1996 Int. Conf. Very Large Data Bases. 1996.
[3] Ranka, Sanjay, and V. Singh. “CLOUDS: A decision tree classifier for large datasets.” Proceedings of the 4th Knowledge Discovery and Data Mining Conference. 1998.
[4] Machado, F. P. “Communication and memory efficient parallel decision tree construction.” (2003).
[5] Li, Ping, Qiang Wu, and Christopher J. Burges. “Mcrank: Learning to rank using multiple classification and gradient boosting.” Advances in neural information processing systems. 2007.
[6] Shi, Haijian. “Best-first decision tree learning.” Diss. The University of Waikato, 2007.
Recommend
About Joyk
Aggregate valuable and interesting links.
Joyk means Joy of geeK