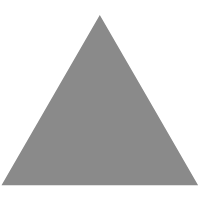
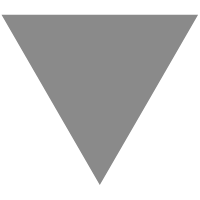
Secret Paragraphs from HBR’s Analytics
source link: https://www.tuicool.com/articles/hit/yyQBNvj
Go to the source link to view the article. You can view the picture content, updated content and better typesetting reading experience. If the link is broken, please click the button below to view the snapshot at that time.
My blog post for this week came out in HBR , so in case you missed that one, you can find it at:
What Great Data Analysts Do — and Why Every Organization Needs Them
I did my best to cut down the length quite aggressively before sending it in, so if you were left wanting more after reading the article , here are some secret paragraphs and B-sides for your amusement. They cover hybrid roles, the nature of research, Bat Signals, charlatans, and more. Enjoy!

Shopping for the trophy hire
Be careful which skills you put on a pedestal, since the effects of unwise choices an be devastating. In addition to mismanaged teams and unnecessary hires, you’ll see the real heroes quitting or reeducating themselves to fit your incentives du jour. A prime example of this phenomenon is in analytics.
Buyer beware
Unfortunately, relatively few analysts are the real deal — buyer beware: there are many data charlatans out there posing as data scientists or data analysts. These peddle nonsense, leaping beyond the data in undisciplined ways to “support” decisions based on wishful thinking. If your ethical standards are lax, perhaps you’d keep these snake oil salesmen around and house them in the marketing dark arts part of your business. Personally, I’d prefer not to.
The true nature of a statistician
What most people don’t realize is that statisticians are essentially epistemologists. Since there’s no magic that makes certainty out of uncertainty, their role is not to produce Truth but rather a sensible integration of palatable assumptions with available information.
Unsurprisingly, many statisticians react with vitriol toward “upstarts” who learn the equations without absorbing any of the philosophy. If dealing with statisticians seems exhausting, here’s a quick fix: don’t come to any conclusions beyond your data and you won’t need their services. ( Easier said than done, right? Especially if you want to make an important launch decision. )
Sloppy nonsense or stellar storytelling?
To avoid wasted time, analysts should lay out the story they’re tempted to tell and poke it the from several angles with followup investigations to see if it holds water before bringing it to decision-makers. If a decision-maker is in danger of being driven to take an important action based on an inspiring story, that is the Bat-Signal for the statisticians to swoop in and check (in new data, of course) that the action is a wise choice in light of assumptions the decision-maker is willing to live with and their appetite for risk.
The analyst-statistician hybrid
For analysts sticking to the facts, there’s no such thing as wrong, there’s only slow. Adding statistical expertise to “do things correctly” misses the point in an important way, especially because there’s a very important filter between exploratory data analytics and statistical rigor: the decision-maker . Someone with decision responsibility has to sign off on the business impact of pursing the analyst’s insight being worth a high-effort expert’s time. Unless the analyst-statistician hybrid is also a skilled decision-maker and business leader, their skillset forms a sandwich with a chasm in the middle.
An analyst who bridges that gap, however, is worth their weight in gold. Treasure them!
The analyst-ML expert hybrid
Analysts accelerate machine learning projects, so dual skillsets are very useful. Unfortunately, because of the differences in coding style and approach between analytics and ML engineering , it’s unusual to see peak expertise in one individual (and even rarer to that person to be slow and philosophical when needed, which is why the true full-stack data scientist is a rare beast indeed).
The dangers of under-appreciating analysts
What beginners don’t realize is that the work requires top analysts to have a better grasp of the mathematics of data science than either of the other applied breeds. Unless the task is complicated enough that it demands the invention a new hypothesis test or algorithm (the work of researchers ), statisticians and ML specialists can rely on checking that off-the-shelf packages and tests are right for the job, but they can often skip having to face the equations themselves.
For example, statisticians might forget the equations for a t -test’s p-value because they get it by hitting run on a software package, but they never forget how and when to use one, as well as the correct philosophical interpretation of the results. Analysts, on the other hand, aren’t looking to interpret. They’re after a view into the shape of a gory, huge, multidimensional dataset. By knowing the way the equation for the p-value slices their dataset, they can form a reverse view of what the patterns in original dataset must have been to produce the number they saw. Without an appreciation of the math, you don’t get that view. Unlike a statistician, though, they don’t care if the t -test is right for the data. They care that the t-test gives them a useful view of what’s going on in the current dataset. The distinction is subtle, but it’s important. Statisticians deal with things outside the data, while analysts stick to things inside it. At peak excellence, both are deeply mathematical and they often use the same equations, but their jobs are entirely different.
Similarly, analysts often use machine learning algorithms to slice their data, identify compelling groupings, and examine anomalies. Since their goal is not performance but inspiration, their approach is different and might appear sloppy to the ML engineer . Again, it’s the use of the same tool for a different job.
To summarize what’s going on with an analogy: pins are used by surgeons, tailors, and office workers. That doesn’t mean the jobs are the same or even comparable, and it would be dangerous to encourage all your tailors and office workers to study surgery to progress in their careers.
Care and feeding of researchers
If this doesn’t sound bad enough, many leaders try to hire PhDs and overemphasize research — as opposed to applied — versions of the statistician and ML engineer … without having a problem that is valuable, important, and known to be impossible to solve with all the existing algorithms out there.
That’s only okay if you’re investing in a research division and you’re not planning to ask your researchers what they’ve done for you lately. Research for research’s sake is a high-risk investment and very few companies can afford it, because getting nothing of value out of it is a very real possibility.
Researchers only belong outside of a research division if you have appropriate problems for them to solve — their skillset is creating new algorithms and tests from scratch where an off-the-shelf version doesn’t exist — otherwise they’ll experience a bleak Sisyphean spiral (which would be entirely your fault, not theirs). Researchers typically spend over a decade in training, which merits at least the respect of not being put to work on completely irrelevant tasks.
As a result, the right time to hire them to an applied project tends to be after your analysts helped you identify a valuable project and attempts to complete it with applied data scientists have already failed. That’s when you bring on the professional inventors.

Recommend
About Joyk
Aggregate valuable and interesting links.
Joyk means Joy of geeK