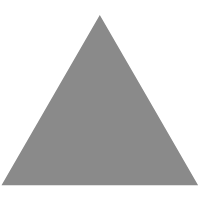
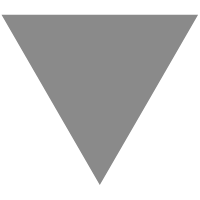
TimescaleDB vs. InfluxDB: purpose built differently for time-series data
source link: https://www.tuicool.com/articles/hit/bIbqYrE
Go to the source link to view the article. You can view the picture content, updated content and better typesetting reading experience. If the link is broken, please click the button below to view the snapshot at that time.
An in-depth look into how two leading time-series databases stack up in terms of data model, query language, reliability, performance, ecosystem, operational management, and company/community support

T ime-series data is emerging in more and more applications, including IoT, DevOps, Finance, Retail, Logistics, Oil and Gas, Manufacturing, Automotive, Aerospace, SaaS, even machine learning and AI. Most recently, the focus of time-series databases has been narrowly on metrics and monitoring; today it’s become clear that software developers really need a true time-series database designed for a variety of operational workloads.
If you are investing in a time-series database, that likely means you already have a meaningful amount of time-series data piling up quickly and need a place to store and analyze it. You may also recognize that the survival of your business will depend on the database you choose.
How to choose a time-series database
There are several factors to consider when evaluating a time-series database for your workload:
- Data model
- Query language
- Reliability
- Performance
- Ecosystem
- Operational management
- Company and community support
In this article, we compare two leading time-series databases,TimescaleDB and InfluxDB , to help software developers choose the time-series database best suited for their needs.
Typically database comparisons focus on performance benchmarks. Yet performance is just a part of the overall picture; it doesn’t matter how good benchmarks are if a database can’t be used in production because it has an incompatible data model or query language, or if it lacks reliability. With that in mind, we begin by comparing TimescaleDB and InfluxDB across three qualitative dimensions, data model, query language, and reliability, before diving deeper with performance benchmarks . We then round out with a comparison across database ecosystem, operational management, and company/community support .
Yes, we are the developers of TimescaleDB, so you might quickly disregard our comparison as biased. But if you let the analysis speak for itself, you’ll find that it tries to stay objective — indeed, we report several scenarios below in which InfluxDB outperforms TimescaleDB.
Also, this comparison isn’t a purely theoretical activity for us. Our company began as an IoT platform, where we first used InfluxDB to store our sensor data. However, owing to most of the differences listed below, we found InfluxDB unsatisfactory. So we built TimescaleDB as the first time-series database that satisfied our needs, and then discovered others who needed it as well, which is when we decided to open source the database. Today, less than 1.5 years later, TimescaleDB has already been downloaded hundreds of thousands of times and is deployed in production all around the world. ( More on the genesis of TimescaleDB. )
In the end, our goal is help you decide which is the best time-series database for your needs.
What about “scalability”?
If you look closely at the list above, you’ll see that “scalability” or even “clustering” is missing. What we’ve found is that when developers ask for either of these requirements, what they are really asking is some combination of a) performance metrics b) high availability and/or c) storage capacity. We find it more helpful to speak about these three topics independently, instead of lumping them into a catch-all term, which is what we’ve done below.
Recommend
About Joyk
Aggregate valuable and interesting links.
Joyk means Joy of geeK