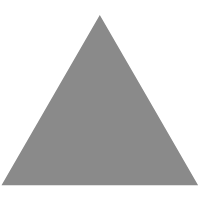
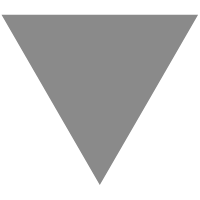
GPUs as a service with Kubernetes Engine are now generally available
source link: https://chinagdg.org/2018/06/gpus-as-a-service-with-kubernetes-engine-are-now-generally-available/
Go to the source link to view the article. You can view the picture content, updated content and better typesetting reading experience. If the link is broken, please click the button below to view the snapshot at that time.

GPUs as a service with Kubernetes Engine are now generally available
Source: GPUs as a service with Kubernetes Engine are now generally available from Google Cloud Platform
By Yoshi Tamura, Product Manager, Google Kubernetes Engine
[Editor’s note: This is one of many posts on enterprise features enabled by Kubernetes Engine 1.10. For the full coverage, follow along here.]
Today, we’re excited to announce the general availability of GPUs in Google Kubernetes Engine, which have become one of the platform’s fastest growing features since they entered beta earlier this year, with core-hours soaring by 10X since the end of 2017.
Together with the GA of Kubernetes Engine 1.10, GPUs make Kubernetes Engine a great fit for enterprise machine learning (ML) workloads. By using GPUs in Kubernetes Engine for your CUDA workloads, you benefit from the massive processing power of GPUs whenever you need, without having to manage hardware or even VMs. We recently introduced the latest and the fastest NVIDIA Tesla V100 to the portfolio, and the P100 is generally available. Last but not least, we also offer the entry-level K80, which is largely responsible for the popularity of GPUs. All our GPU models are available as Preemptible GPUs, as a way to reduce costs while benefiting from GPUs in Google Cloud. Check out the latest prices for GPUs here.
As the growth in GPU core-hours indicates, our users are excited about GPUs in Kubernetes Engine. Ocado, the world’s largest online-only grocery retailer, is always looking to apply state-of-the-art machine learning models for Ocado.com customers and Ocado Smart Platform retail partners, and runs the models on preemptible, GPU-accelerated instances on Kubernetes Engine.
“GPU-attached nodes combined with Kubernetes provide a powerful, cost-effective and flexible environment for enterprise-grade machine learning. Ocado chose Kubernetes for its scalability, portability, strong ecosystem and huge community support. It’s lighter, more flexible and easier to maintain compared to a cluster of traditional VMs. It also has great ease-of-use and the ability to attach hardware accelerators such as GPUs and TPUs, providing a huge boost over traditional CPUs.”
— Martin Nikolov, Research Software Engineer, Ocado
GPUs in Kubernetes Engine also have a number of unique features:
- Node Pools allow your existing cluster to use GPUs whenever you need.
- Cluster Autoscaler automatically creates nodes with GPUs whenever pods requesting GPUs are scheduled, and scale down to zero when GPUs are no longer consumed by any active pods.
- Taint and toleration technology ensures that only pods that request GPUs will be scheduled on the nodes with GPUs, and prevents pods that do not require GPUs from running on them.
- Resource quota that allows administrators to limit resource consumption per namespace in a large cluster shared by multiple users or teams.
We also heard from you that you need an easy way to understand how your GPU jobs are performing: how busy the GPUs are, how much memory is available, and how much memory is allocated. We are thrilled to announce that you can now monitor those information natively from the GCP Console.You can also visualize these metrics in Stackdriver.

The general availability of GPUs in Kubernetes Engine represents a lot of hard work behind the scenes, polishing the internals for enterprise workloads. Jiaying Zhang, the technical lead for this general availability, led the Device Plugins effort in Kubernetes 1.10, working closely with the OSS community to understand its needs, identify common requirements, and come up with an execution plan to build a production-ready system.
Try them today
To get started using GPUs in Kubernetes Engine using our free-trial of $300 credits, you’ll need to upgrade your account and apply for a GPU quota for the credits to take effect. For a more detailed explanation of Kubernetes Engine with GPUs, for example how to install NVIDIA drivers and how to configure a pod to consume GPUs, check out the documentation.
In addition to GPUs in Kubernetes Engine, Cloud TPUs are also now publicly available in Google Cloud. For example, RiseML uses Cloud TPUs in Kubernetes Engine for a hassle-free machine learning infrastructure that is easy-to-use, highly scalable, and cost-efficient. If you want to be among the first to access Cloud TPUs in Kubernetes Engine, join our early access program today.
Thanks for your feedback on how to shape our roadmap to better serve your needs. Keep the conversation going by connecting with us on the Kubernetes Engine Slack channel.
Recommend
About Joyk
Aggregate valuable and interesting links.
Joyk means Joy of geeK